PsfDeconNet: High-Resolution Seismic Imaging Using Point-Spread Function Deconvolution With Generative Adversarial Networks
IEEE TRANSACTIONS ON GEOSCIENCE AND REMOTE SENSING(2024)
摘要
Least-squares migration (LSM) aims to seek the best-fit solution for subsurface reflectivity with high image resolution and balanced amplitudes by minimizing the mismatching between synthetic and observed seismic data. It can be implemented either in data domain or in image domain. Data-domain LSM iteratively updates reflectivity using gradient-based algorithms. However, it requires expensive computation cost to converge to a good solution, which is still challenging for large-scale datasets under current computational capacity. Point-spread function (PSF) deconvolution is an efficient and accurate image-domain LSM approach to reduce migration artifacts caused by the limited aperture and the finite frequency wavelet and improve image resolution. However, seismic velocity models have millions of grid points, which makes it prohibitively expensive to directly compute the PSF and its deconvolution operator. To obtain high-resolution images and reduce computing cost, we develop a deep-learning-based method to directly approximate the deconvolution operator of each separated PSF. First, we calculate migrated image and PSFs using one pass of seismic modeling and traditional adjoint migration. Next, we train conditional generative adversarial networks (cGANs) by using the migrated images, PSFs, and migration velocity as the input and the true reflectivity as the labeled data. Finally, the trained network is applied to the migrated images, PSFs, and migration velocity of test datasets to predict the reflectivity model. With the well-trained cGANs, we can predict high-quality LSM images efficiently and save considerable computational cost. Numerical examples for synthetic models and field data demonstrate that the proposed method can accurately predict PSF deconvolution operators and provide high-quality deblurred LSM images with significantly reduced computational and memory costs.
更多查看译文
关键词
Computational seismology,conditional generative adversarial networks (cGANs),least-squares migration (LSM),point-spread function (PSF) deconvolution
AI 理解论文
溯源树
样例
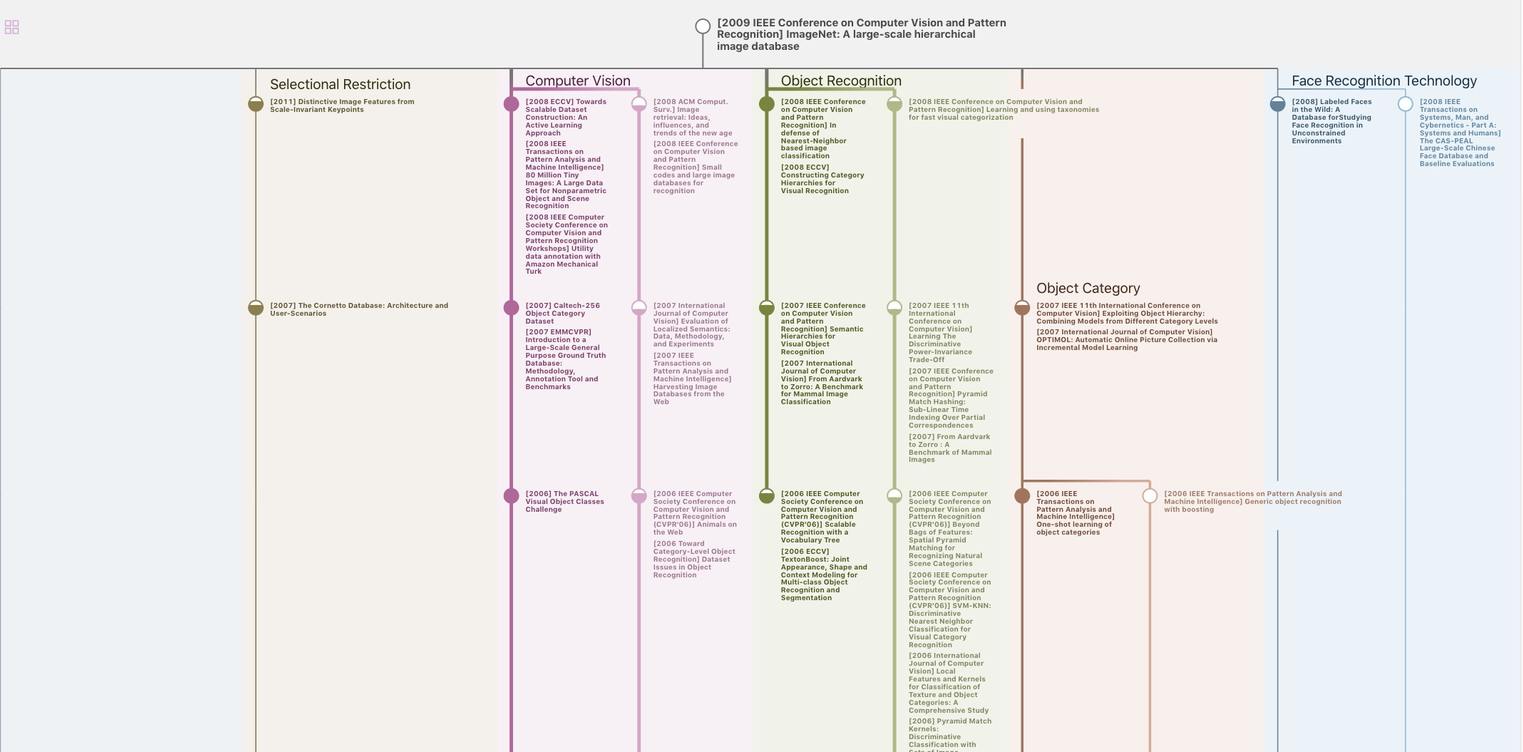
生成溯源树,研究论文发展脉络
Chat Paper
正在生成论文摘要