HyperSINet: A Synergetic Interaction Network Combined With Convolution and Transformer for Hyperspectral Image Classification
IEEE TRANSACTIONS ON GEOSCIENCE AND REMOTE SENSING(2024)
摘要
In hyperspectral images (HSIs), both local and nonlocal features play crucial roles in classification tasks. Vision transformers (VITs) can extract nonlocal features through attention mechanisms, while convolutional neural networks (CNNs) excel at handling local components. However, in traditional dual-branch models based on VIT and CNN, there is a lack of interaction during feature processing, leading to potential compatibility issues when merging the two types of features. In this article, we propose HyperSINet, a synergetic interaction network that combines VIT and CNN to establish interaction between the two branches, enabling mutual compensation between local and nonlocal features during the training process and ultimately enhancing the performance of classification tasks. Specifically, we devise a pair of interactors, namely, Conv2Trans and Trans2Conv, which serve as intermediaries between the two branches, enabling the VIT branch to refine its local details, while allowing the CNN branch to process larger receptive field nonlocal features. Typical feature maps are implemented to visualize the function of the interactors. Furthermore, within the VIT branch, a VIT encoder with the local mask is developed to strike a balance between emphasizing nonlocal features and preserving local details, while a lightweight CNN block is designed to process spectral and spatial features in the CNN branch. Extensive experiments conducted on four real-world datasets demonstrate that, under a reasonable count of parameters, HyperSINet surpasses several current state-of-the-art methods.
更多查看译文
关键词
Convolutional neural network (CNN),hyperspectral image (HIS) classification,interactors,synergetic interaction,vision transformer (VIT)
AI 理解论文
溯源树
样例
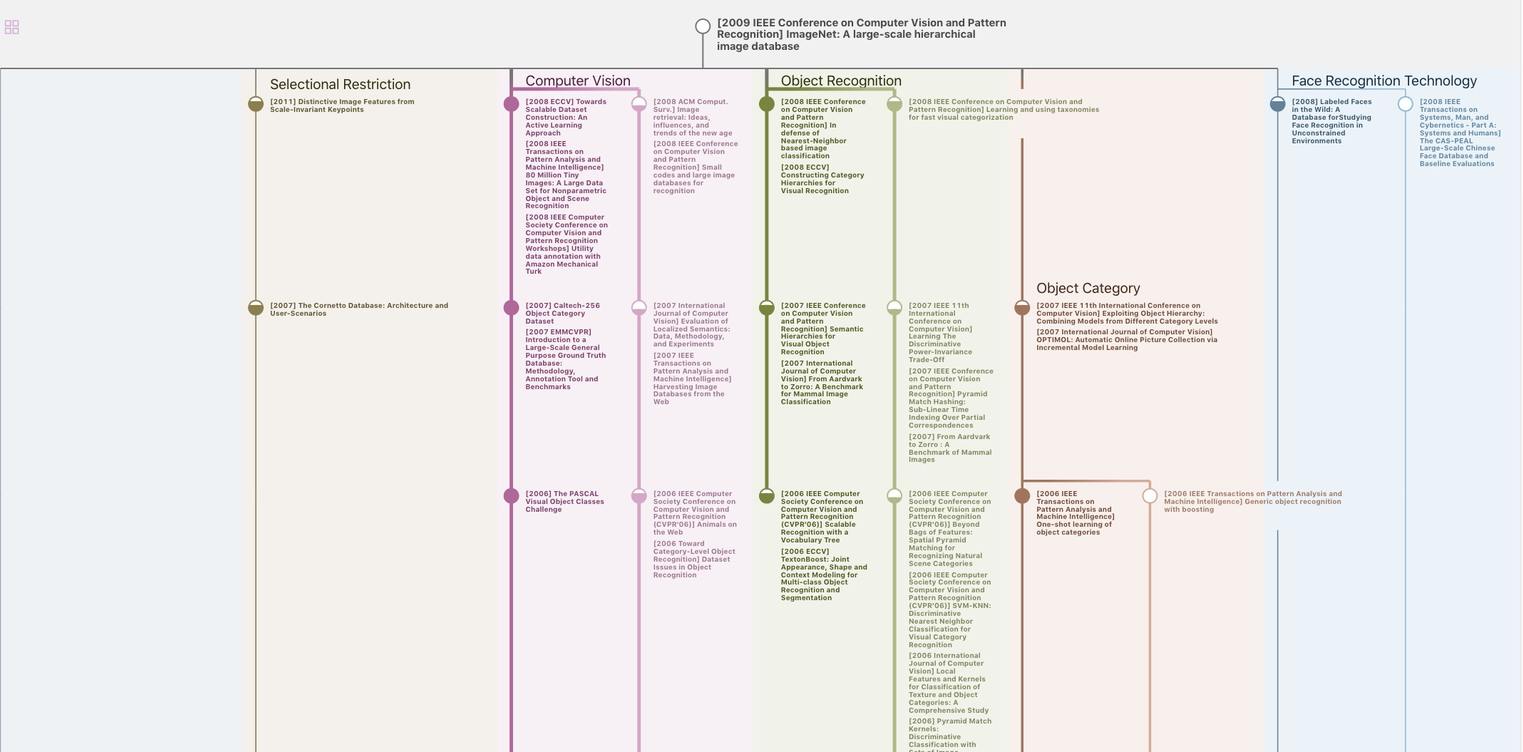
生成溯源树,研究论文发展脉络
Chat Paper
正在生成论文摘要