Global-Local Semantic Interaction Network for Salient Object Detection in Optical Remote Sensing Images with Scribble Supervision.
IEEE geoscience and remote sensing letters(2024)
摘要
Salient object detection in optical remote sensing images (RSI-SOD) is critical in remote sensing, yet it faces challenges such as dependency on intensive pixel-level annotations and limited research on low-cost, weakly supervised methods. These challenges are compounded by difficulties in handling complex backgrounds and varying salient object features with existing CNN-based methods. We propose the global-local semantic interaction network (GLSIN), a high-performance, cost-effective RSI-SOD approach based on scribble supervision. GLSIN employs an encoder-decoder framework, blending a Transformer and CNN to create a Dual Branch Encoder that effectively captures both global and local features of images. The global-local affinity block (GLAB) and Feature Shrinkage Decoder with the global-local fusion block (GLFB) are integrated to enhance feature interaction and precision in saliency map generation. Experimental results on two public datasets show that our method achieves F-beta(max), F-xi(max), F-alpha, and M scores of 86.6%, 96.5%, 91.8%, and 0.7% on the EORSSD dataset, and 90.1%, 97.2%, 91.7%, and 1.1% on the ORSSD dataset, respectively. The performance surpasses existing weakly supervised or unsupervised SOD methods and even some fully supervised models.
更多查看译文
关键词
Optical remote sensing images (RSIs),salient object detection (SOD),scribble supervision,sparse annotation,transformer
AI 理解论文
溯源树
样例
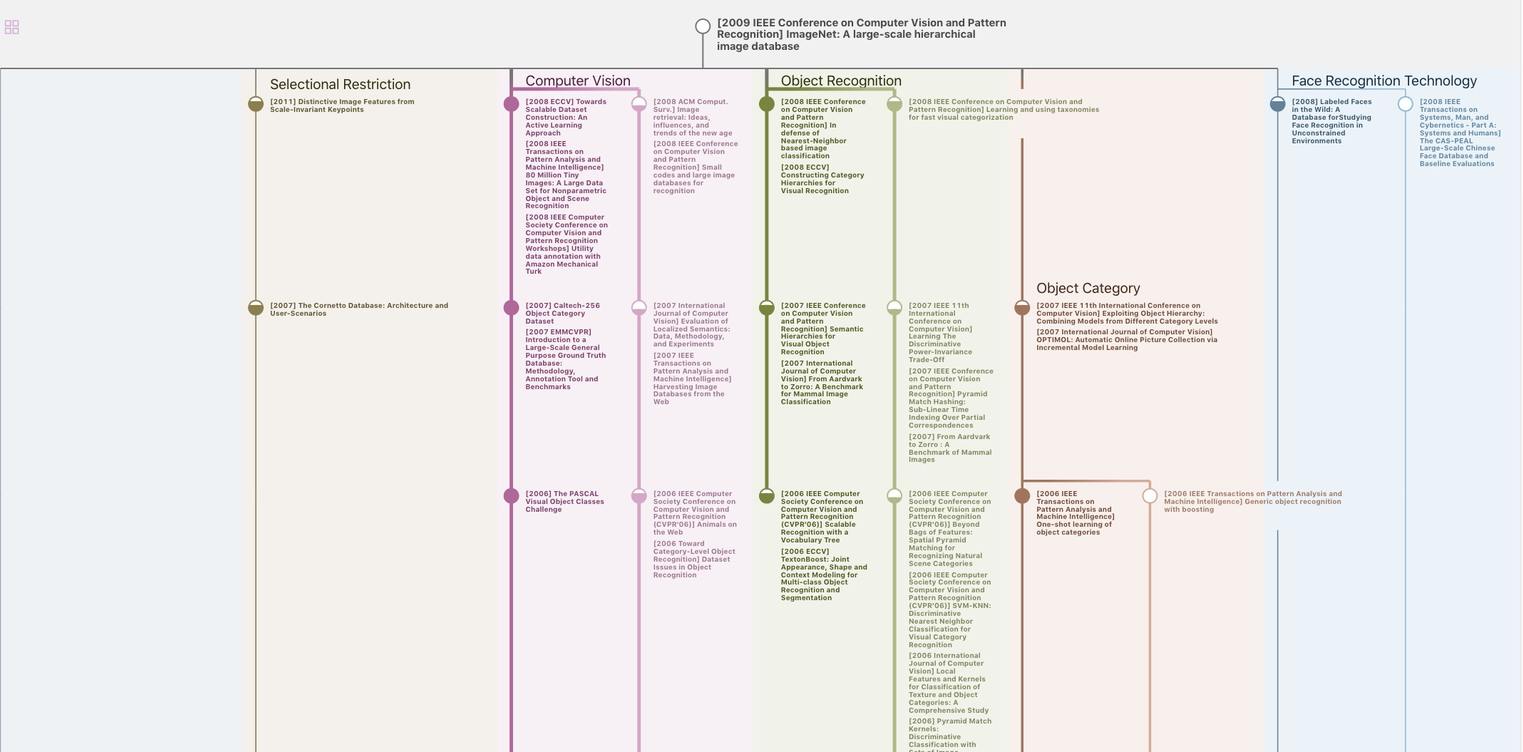
生成溯源树,研究论文发展脉络
Chat Paper
正在生成论文摘要