Fuel consumption cost prediction model for ro-ro carriers: a machine learning-based application
MARITIME POLICY & MANAGEMENT(2024)
摘要
Shipping companies are facing rising fuel consumption costs and pressure to reduce emissions, so reducing logistics consumption costs and addressing stakeholders' environmental concerns have become urgent tasks. This study aims to develop a ship fuel cost prediction model using statistical and machine learning methods. We analyzed a dataset from a large PCTC shipping company in South Korea containing 16,189 observations for the period from 2012 to 2021. Fuel consumption costs was predicted using features such as date, ship size, route, distance, speed, sea day, port call day, and duration. We used the CatBoost algorithm for deep learning and evaluated the effectiveness of 18 methods. The CatBoost algorithm has an R2 value of 0.976, which is better than other methods. In addition, the variables of distance, sea days, speed, duration, and port days had a significant effect on the prediction results. We determined this effect using Shapley's additive interpretation (SHAP). This study can help PCTC companies efficiently estimate fuel consumption costs and meet environmental requirements, thereby improving operational efficiency and promoting sustainable practices in the shipping industry. We also enrich the existing body of knowledge by providing insights on predictive modeling techniques for fuel consumption costs in maritime logistics.
更多查看译文
关键词
Maritime transportation,ship fuel consumption costs,logistics data mining,machine learning,fuel consumption costs prediction,deep learning
AI 理解论文
溯源树
样例
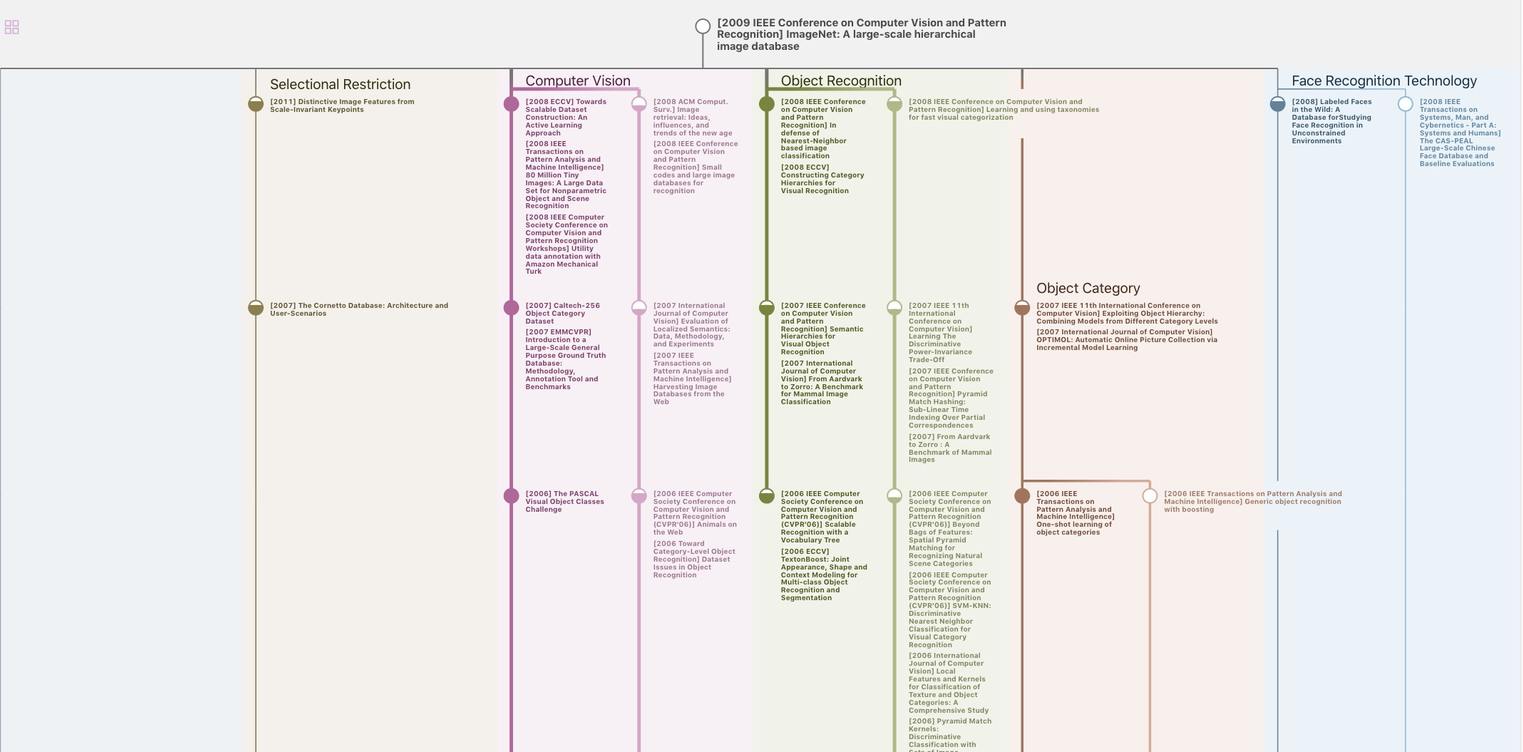
生成溯源树,研究论文发展脉络
Chat Paper
正在生成论文摘要