Exploratory image data analysis for quality improvement hypothesis generation
QUALITY ENGINEERING(2023)
摘要
Images can provide critical information for quality engineering. Exploratory image data analysis (EIDA) is proposed here as a special case of EDA (exploratory data analysis) for quality improvement problems with image data. The EIDA method aims to obtain useful information from the image data to identify hypotheses for additional exploration relating to key inputs or outputs. The proposed four steps of EIDA are: (1) image processing, (2) image-derived quantitative data analysis and display, (3) salient feature (pattern) identification, and (4) salient feature (pattern) interpretation. Three examples illustrate the methods for identifying and prioritizing issues for quality improvement, identifying key input variables for future study, identifying outliers, and formulating causal hypotheses.
更多查看译文
关键词
body-in-white,computer vision,exploratory data analysis,graphical data analysis,image processing,pattern discovery,pipeline inspection,quality improvement,welding
AI 理解论文
溯源树
样例
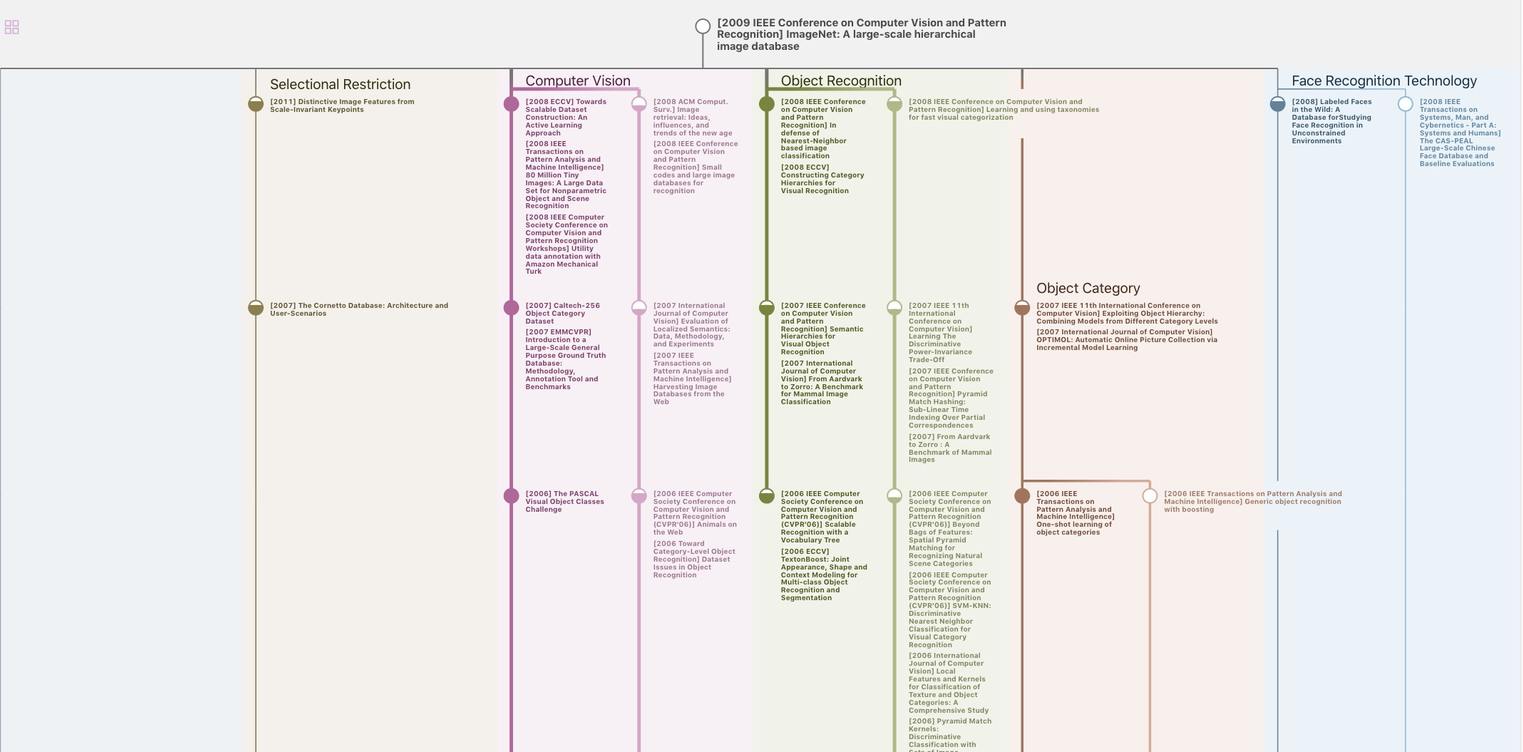
生成溯源树,研究论文发展脉络
Chat Paper
正在生成论文摘要