PointSGRADE: Sparse learning with graph representation for anomaly detection by using unstructured 3D point cloud data
IISE TRANSACTIONS(2023)
摘要
Surface anomaly detection by using 3D point cloud data has recently received significant attention. To completely measure the common free-form surfaces without loss of details, advanced 3D scanning technologies, such as 3D laser scanners, can be applied and will produce an unstructured point cloud. However, this irregular data structure poses challenges to anomaly detection, in that the existing methods based on regular data, e.g., 2D image, cannot be directly applied. This article proposes a sparse learning framework with a graph representation of the unstructured point cloud for anomaly detection (PointSGRADE). Specifically, the free-form surface is assumed to be smooth. Then, the associated point cloud can be represented as a graph. Subsequently, considering the sparse anomalies, we propose a sparse learning framework and formulate the anomaly detection problem as a penalized optimization problem, which is further solved by a computationally efficient majorization-minimization framework. Case studies demonstrate the accuracy and robustness of the proposed method. This article proposes a novel methodology for sparse anomaly detection on smooth free-form surfaces represented by unstructured point cloud, which is critical for quality inspection in manufacturing and other application areas.
更多查看译文
关键词
Anomaly detection,unstructured 3D point cloud data,graph representation,sparse learning,majorization-minimization,free-form surface,smooth surface
AI 理解论文
溯源树
样例
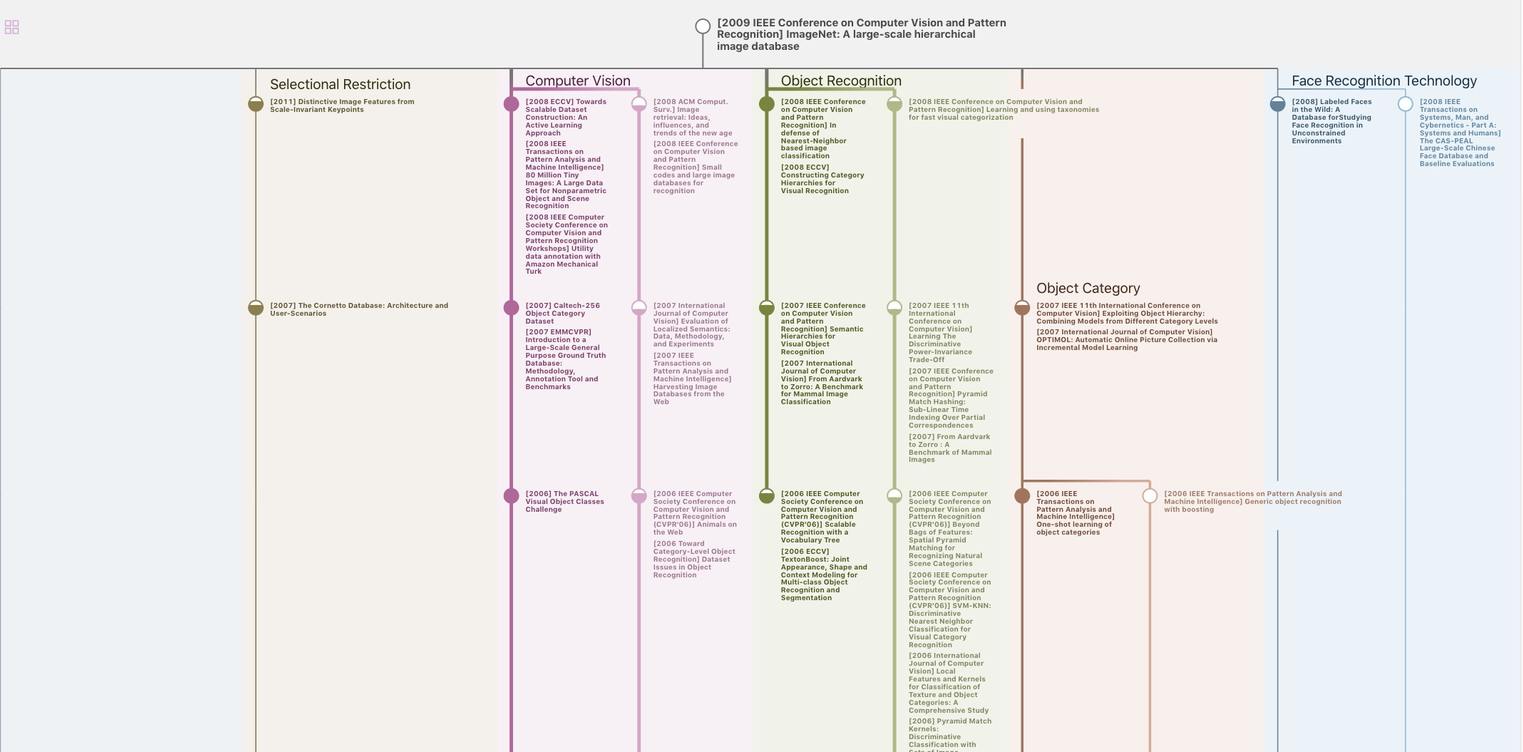
生成溯源树,研究论文发展脉络
Chat Paper
正在生成论文摘要