A Nature-Inspired Method to Mine Top-k Multi-Level High-Utility Itemsets
CYBERNETICS AND SYSTEMS(2023)
关键词
Cross-entropy,data mining,high-utility itemset mining,multi-level abstract database,top-k multi-level high-utility itemset
AI 理解论文
溯源树
样例
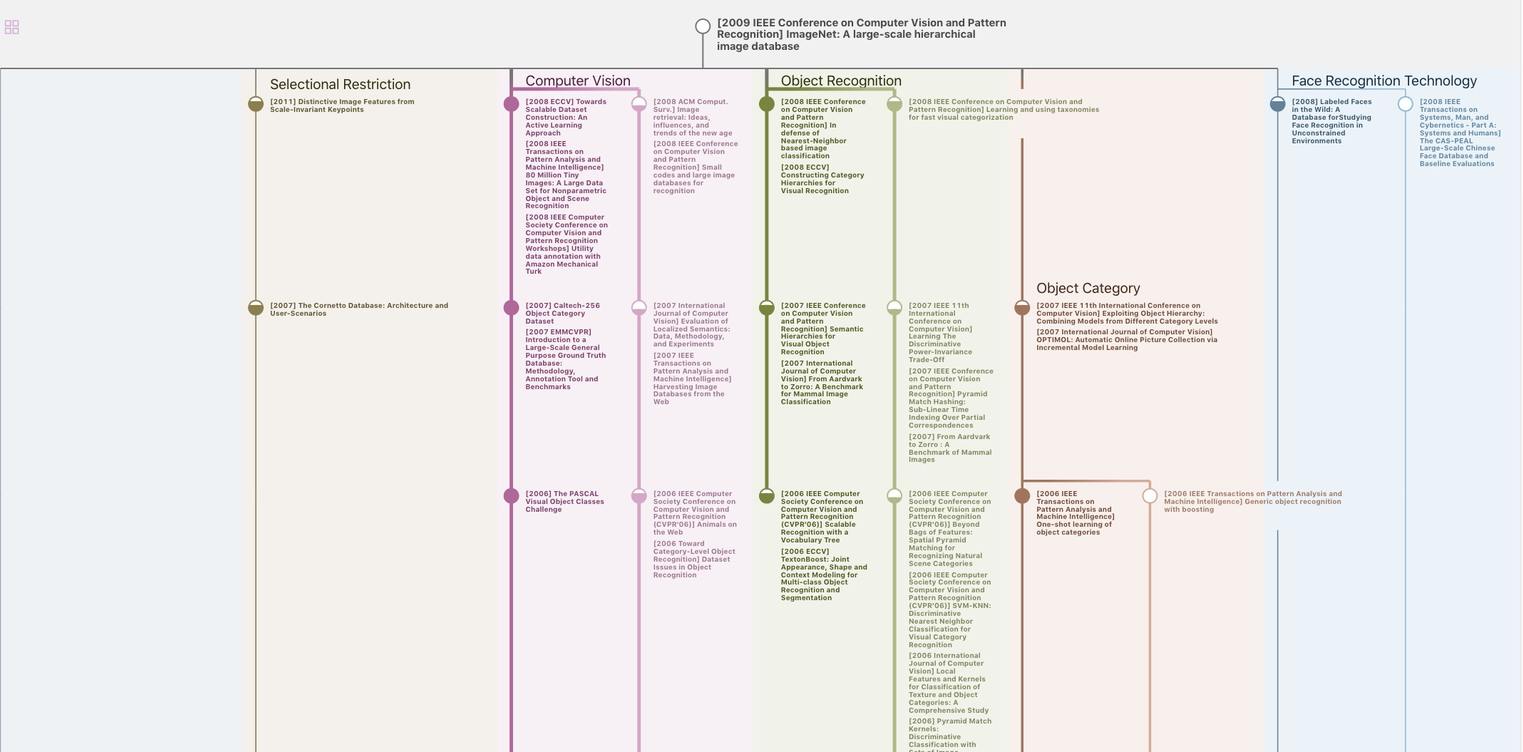
生成溯源树,研究论文发展脉络
Chat Paper
正在生成论文摘要