Large-scale LOD1 Building Extraction from a Textured 3D Mesh of a Scene
IMAGE AND SIGNAL PROCESSING FOR REMOTE SENSING XXIX(2023)
摘要
We propose a novel deep learning approach which performs building semantic segmentation of large-scale textured 3D meshes, followed by a polygonal extraction of footprints and heights. Extracting accurate individual building structures poses a challenge due to the complexity and the variety of architecture and urban designs, where a single overhead image is not enough. Integrating elevation data from a 3D mesh allows to better distinguish individual buildings in three-dimensional space. Another advantage is to avoid occlusion issues in the case of oblique imagery, where tall buildings mask smaller buildings behind them in the case of non-nadir images (especially problematic in urban areas). The proposed method transforms the input data from a 3D textured mesh to a true orthorectified RGB image by rendering both the color information and the depth information from a virtual camera looking straight down. Depth information is then converted to a normalized DSM (nDSM) by subtracting the Copernicus GDEM v3 30-meter digital elevation model (DEM). Viewing the 3D textured mesh as a 4-band raster image (RGB + nDSM) allows us to use a very efficient fully-convolutional neural network based on the U-net architecture for processing large-scale areas. The proposed method was evaluated on three urban areas in Brazil, America, and France. It allows a fourfold improvement in productivity for cartography of buildings in complex urban areas.
更多查看译文
关键词
textured mesh,semantic segmentation,building footprint,normalized DSM,Deep Learning
AI 理解论文
溯源树
样例
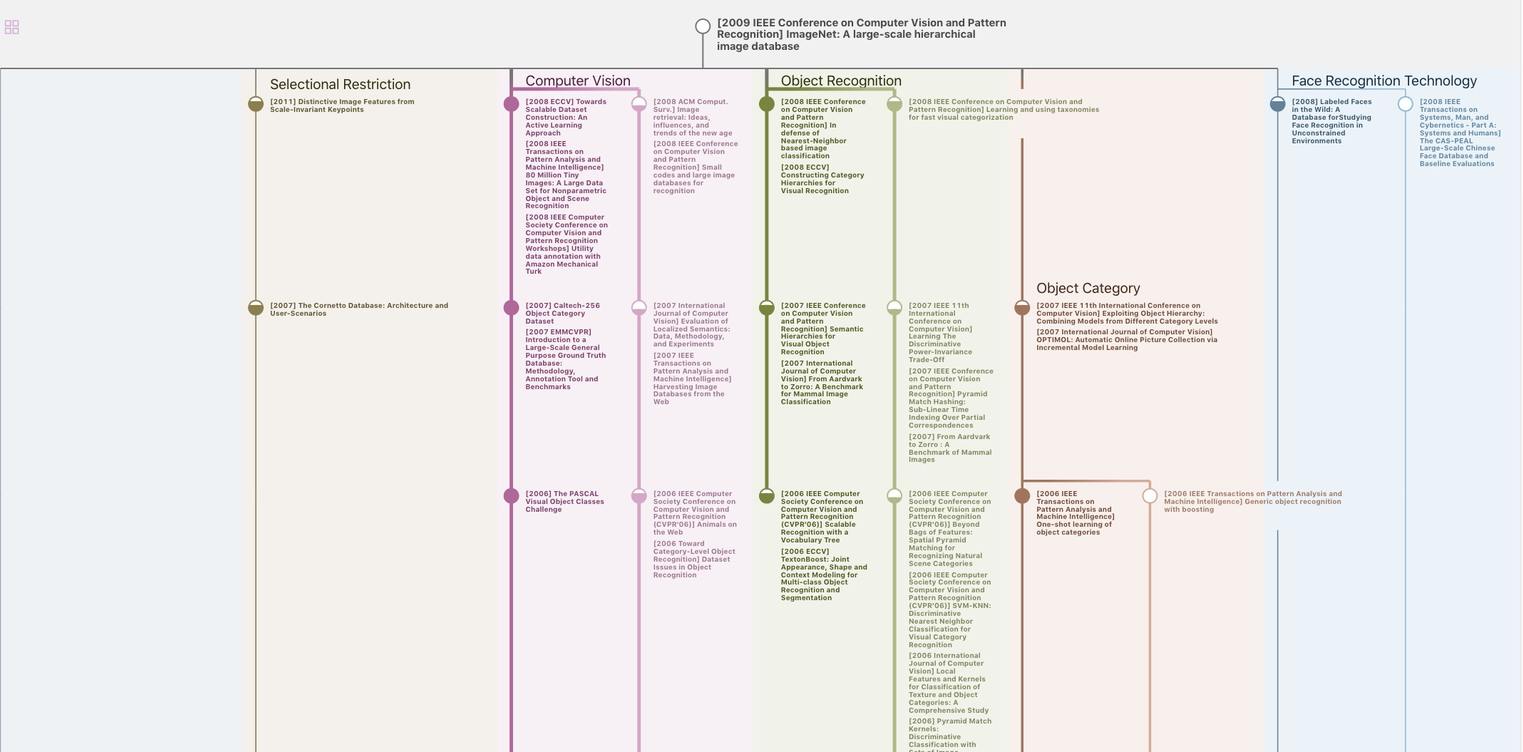
生成溯源树,研究论文发展脉络
Chat Paper
正在生成论文摘要