The potential of SAR data for detecting deforestation in mountainous and sparse forested areas: the case of Northern Portugal
MICROWAVE REMOTE SENSING: DATA PROCESSING AND APPLICATIONS II(2023)
摘要
Change detection in highly dynamic areas, such as vegetated areas, is necessary to improve the thematic accuracy of national land use/cover map production. Sentinel-1 data have proved its potential for change detection. However, its suitability in mountainous and sparse forested areas is not well known. Here, we propose a straightforward clear-cut detection method, using monthly backscatter and coherence composites in both polarizations. The monthly clear-cut masks are estimated using a multivariate alteration detection (MAD) algorithm, using the previous and following composite, and a threshold value corresponding to the 98th percentile. The method was applied to a test site in northern Portugal, mainly characterized by the presence of dense eucalyptus forest, in a mountainous area. A Sentinel-1 time series from February to October 2018 was considered. Results showed that the monthly clear- cut estimation is not only highly influenced by rainfall events, with F1-score values less than 0.11, for the rainy season, but also by terrain-induced geometric distortions and foliage characteristics of the eucalyptus stands. The highest accuracy metrics were obtained for June, with an F1-score value of 0.45, which was still considered unfavorable. Inaccurate monthly estimations are also affected by clear-cut events that occur over more than one month, with the sum of all masks producing results with F1scores less than 0.41. When applied to another region, located southwest of the former site and near the coastline, but characterized by smoother slopes and scattered and sparse forested stands, the method retrieved equivalent recall, but increased precision of around 0.78 (false alarms reduction), resulting in higher F1-score values (0.56).
更多查看译文
关键词
change detection,forest,SAR data,multivariate alteration detection (MAD)
AI 理解论文
溯源树
样例
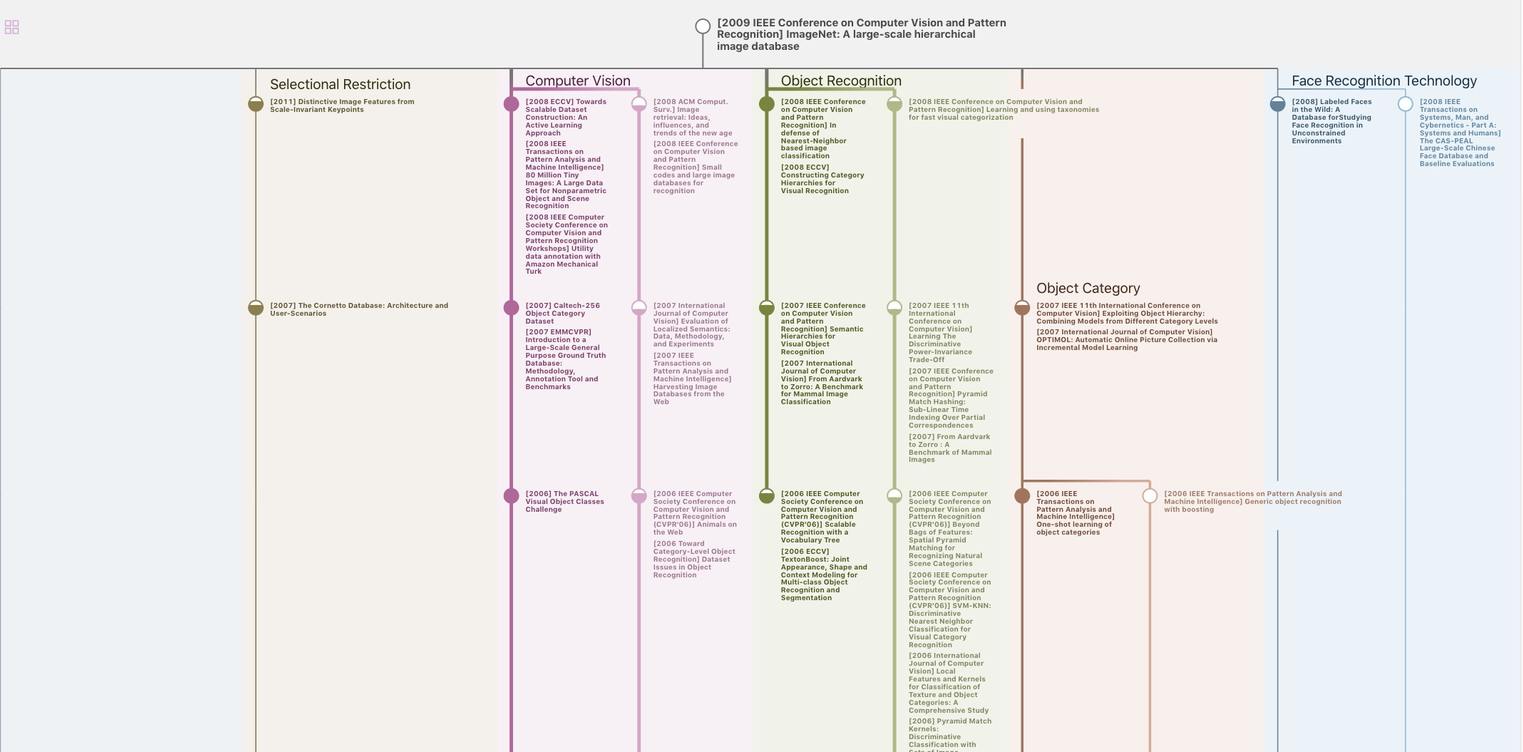
生成溯源树,研究论文发展脉络
Chat Paper
正在生成论文摘要