A receptive field transfer strategy via layer-aligned distillation learning for fault signal denoising
MEASUREMENT SCIENCE AND TECHNOLOGY(2024)
摘要
To improve fault diagnosis performance in complex noise environments, effective signal denoising techniques are necessary. However, traditional denoising methods have proven inadequate for multivariate fault signal denoising, neglecting the correlation among these signals. To this end, we propose a novel denoising module, inspired by traditional signal decomposition and reconstruction methods. Furthermore, to enhance the performance of proposed denoising module, we consider the influence of the receptive field and develop a receptive field transfer strategy using layer-aligned distillation learning. The experiments demonstrate that our approach effectively balances the denoising performance and computational load, offering a novel strategy for developing high-performance denoising networks. What's more, our strategy reduces the difficulty for fault diagnosis tasks under complex noise environments.
更多查看译文
关键词
multivariate fault signal,convolutional neural network,denoising,receptive field,distillation learning
AI 理解论文
溯源树
样例
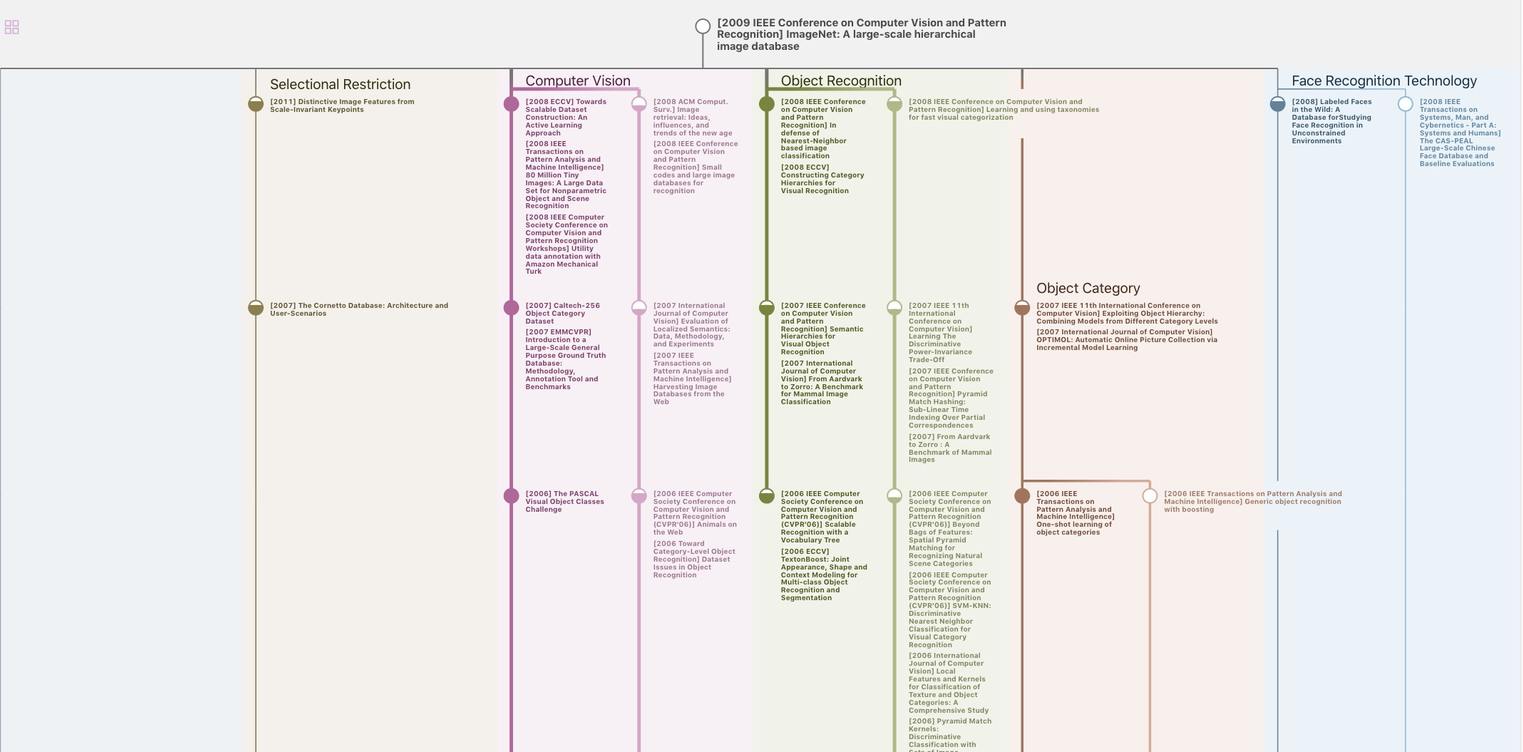
生成溯源树,研究论文发展脉络
Chat Paper
正在生成论文摘要