Bearing Remaining Useful Life Prediction with an Improved CNN-LSTM Network Using an Artificial Gorilla Troop Optimization Algorithm
PROCEEDINGS OF THE INSTITUTION OF MECHANICAL ENGINEERS PART O-JOURNAL OF RISK AND RELIABILITY(2024)
摘要
To address the problem of reliance on a priori knowledge and difficult hyperparameter selection in feature fusion. The effect of different convolutional kernel sizes and filters on feature fusion is investigated firstly, based on which an artificial Gorilla Troops Optimizer (GTO) enhanced Convolutional Long-Short Term Memory Neural Network (CNN-LSTM) method for bearing lifetime prediction is suggested. The GTO algorithm was used to optimize hyperparameters such as the convolutional kernel size of CNN-LSTM, filters and pooling layer size, batch size, number of hidden layer neurons, and rate of learning with the goal of minimizing the mean squared error of the remaining useful life (RUL) prediction. From the optimized CNN-LSTM network analyze the monitored performance degradation data, construct health indicators (HI) reflecting bearing degradation, and build the remaining bearing life prediction model. Typical life cycle data has been used for the validation of the proposed method. The results indicate that the health indicators have better trending and robustness, and leading to smaller errors in life prediction outcomes.
更多查看译文
关键词
Remaining useful life,gorilla troops optimizer,convolutional neural network,long short-term memory,health indicator
AI 理解论文
溯源树
样例
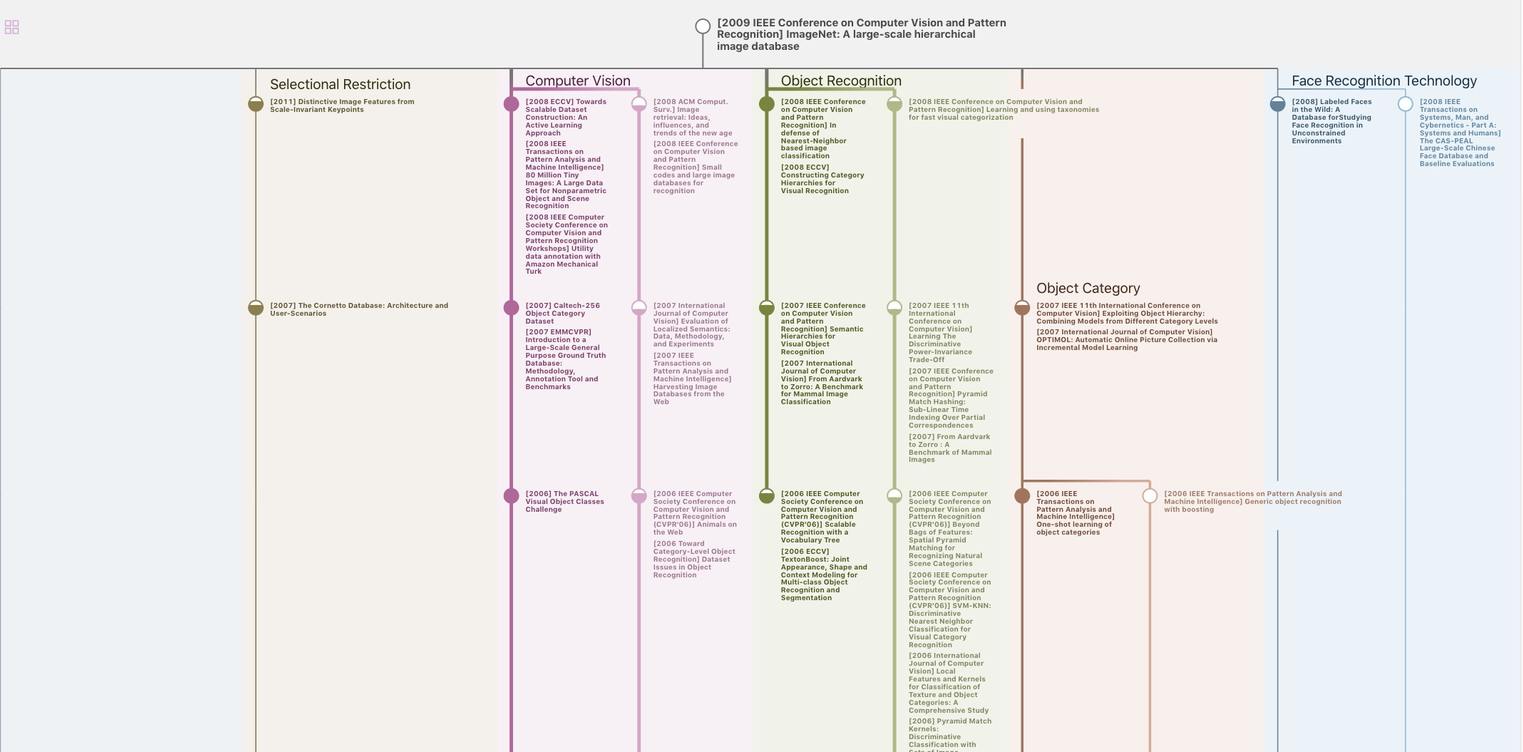
生成溯源树,研究论文发展脉络
Chat Paper
正在生成论文摘要