Bayesian Semiparametric Local Clustering of Multiple Time Series Data
TECHNOMETRICS(2023)
摘要
In multiple time series data, clustering the component profiles can identify meaningful latent groups while also detecting interesting change points in their trajectories. Conventional time series clustering methods, however, suffer the drawback of requiring the co-clustered units to have the same cluster membership throughout the entire time domain. In contrast to these "global" clustering methods, we develop a Bayesian "local" clustering method that allows the functions to flexibly change their cluster memberships over time. We design a Markov chain Monte Carlo algorithm to implement our method. We illustrate the method in several real-world datasets, where time-varying cluster memberships provide meaningful inferences about the underlying processes. These include a public health dataset to showcase the more detailed inference our method can provide over global clustering alternatives, and a temperature dataset to demonstrate our method's utility as a flexible change point detection method. Supplemental materials for this article, including R codes implementing the method, are available online.
更多查看译文
关键词
Change point detection,Hidden Markov model,Local clustering,Time series
AI 理解论文
溯源树
样例
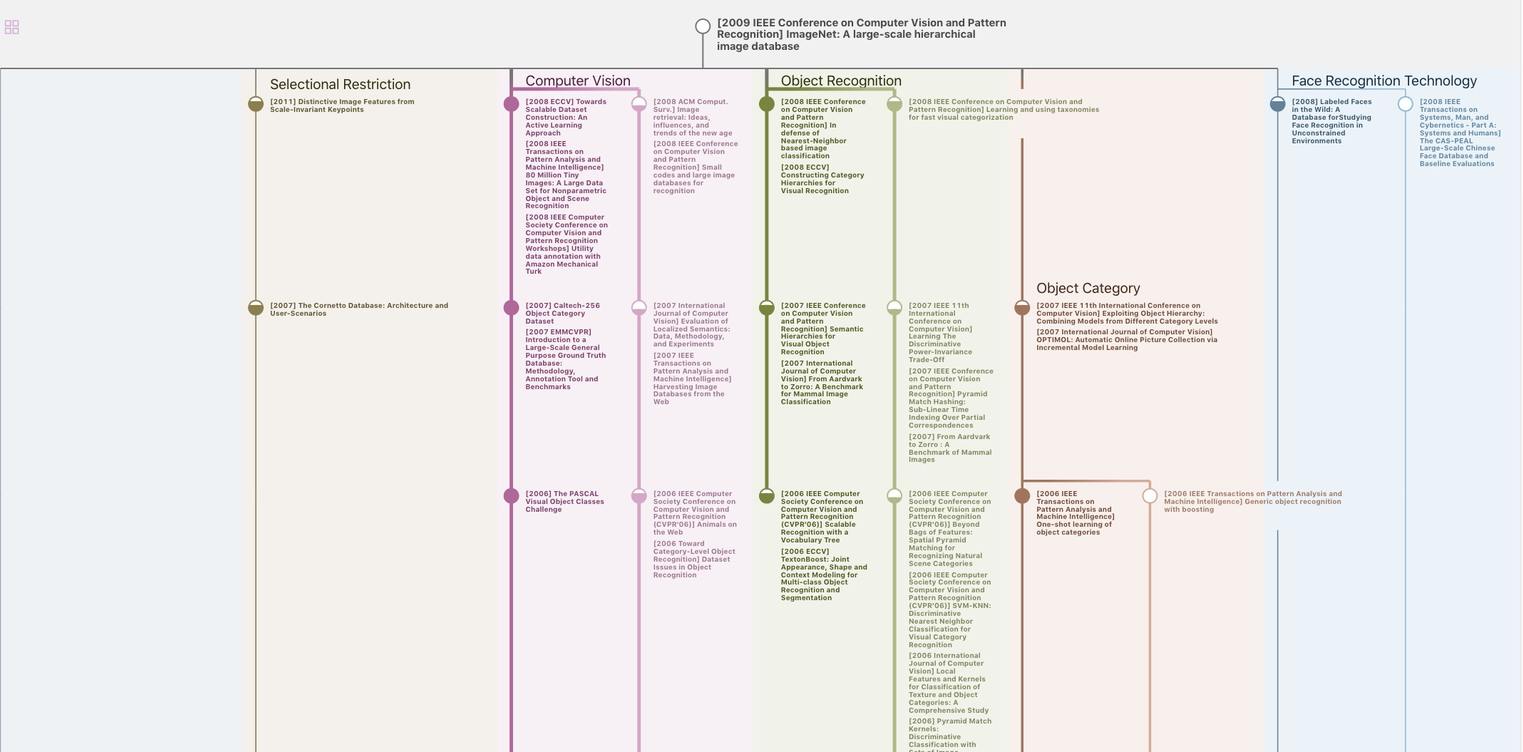
生成溯源树,研究论文发展脉络
Chat Paper
正在生成论文摘要