HG-CAD: Hierarchical Graph Learning for Material Prediction and Recommendation in Computer-Aided Design
JOURNAL OF COMPUTING AND INFORMATION SCIENCE IN ENGINEERING(2024)
摘要
To support intelligent computer-aided design (CAD), we introduce a machine learning architecture, namely HG-CAD, that recommends assembly body material through joint learning of body- and assembly-level features using a hierarchical graph representation. Specifically, we formulate the material prediction and recommendation process as a node-level classification task over a novel hierarchical graph representation of CAD models, with a low-level graph capturing the body geometry, a high-level graph representing the assembly topology, and a batch-level mask randomization enabling contextual awareness. This enables our network to aggregate geometric and topological features from both the body and assembly levels, leading to competitive performance. Qualitative and quantitative evaluation of the proposed architecture on the Fusion 360 Gallery Assembly Dataset demonstrates the feasibility of our approach, outperforming selected computer vision and human baselines while showing promise in application scenarios. The proposed HG-CAD architecture that unifies the processing, encoding, and joint learning of multi-modal CAD features indicates the potential to serve as a recommendation system for design automation and a baseline for future work.
更多查看译文
关键词
hierarchical graph learning,graph neural network,computer-aided design,material recommendation,artificial intelligence,graphics processing unit (GPU),computing for design and manufacturing
AI 理解论文
溯源树
样例
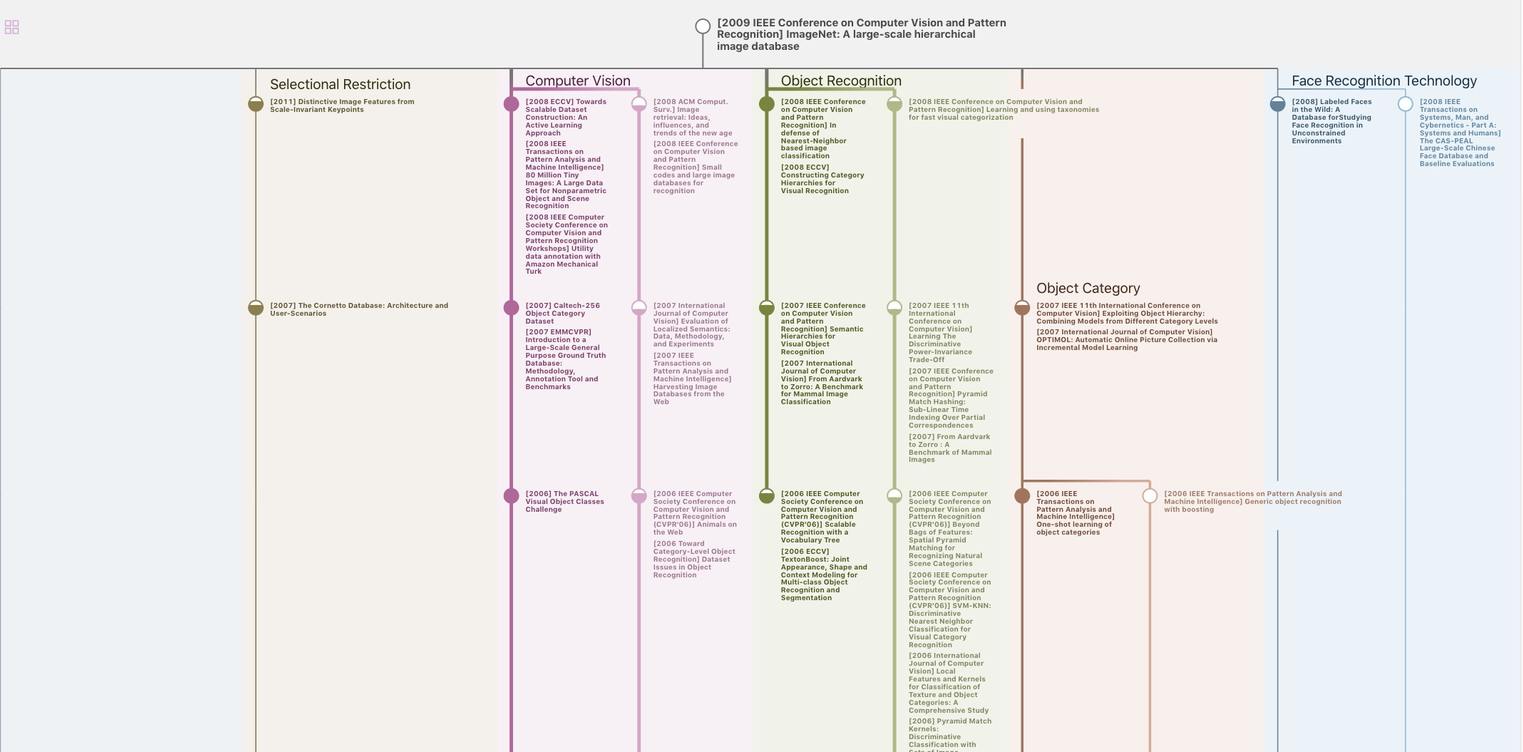
生成溯源树,研究论文发展脉络
Chat Paper
正在生成论文摘要