GRDL: A New Global Reservoir Area-Storage-Depth Data Set Derived Through Deep Learning-Based Bathymetry Reconstruction
WATER RESOURCES RESEARCH(2024)
摘要
Reservoirs play a critical role in the global water cycle by regulating the flow of water from the environment into human systems. Accurate estimation of the area-storage-depth relationships for global reservoirs is essential for effective hydrological modeling and reservoir storage monitoring. Bathymetry reconstruction presents a promising approach to derive this information. Current bathymetry methods either rely on simple approximations or are constrained by dependence on altimetry data or field survey data. To overcome these limitations, this study presents a pioneering approach involving training a deep learning model to reconstruct bathymetry and establish precise area-storage-depth relationships. We trained the deep learning model with approximately 160,000 simulated reservoirs derived from Shuttle Radar Topography Mission (SRTM) and fine-tuned the model based on hundreds of reservoirs with bathymetry data. By employing the trained model and SRTM, the bathymetry of 7,250 reservoirs in the Global Reservoir and Dam Database were subsequently reconstructed. The method is validated against comprehensive reference data sets, including 54 test reservoirs with bathymetry data, 118 satellite altimetry-based reservoirs, and 68 LiDAR-based reservoirs. The reconstructed bathymetry achieves a mean absolute error of 7.87 m and a mean error of +2.05 m for the test reservoirs. The validation against satellite altimetry and LiDAR-based references significantly outperforms previous geometric approximation techniques, with median normalized root mean square error (NRMSE) values of 20.6% for area-storage and 22.1% for area-level curves. Additionally, the reservoir storage variations are estimated with precision, outperforming previous methods. The proposed deep learning-based approach presents a robust solution for accurate reservoir bathymetry estimation and establishes more reliable area-storage-depth relationships for reservoirs worldwide. Reservoirs are large bodies of water used to store and manage our water supply. Knowing how much water they can hold and at what depth is crucial for our understanding of water management and the environment. Our study explored the application of machine learning to better estimate how much water is in a reservoir at any given depth. Traditional methods have shown limitations, prompting us to develop a machine learning model for more precise estimations. Using our new approach, we were able to more accurately measure the depth and storage of over 7,000 reservoirs worldwide. Our results were more precise than older methods when we tested them against well-known data sets. This new machine learning-based method can provide better information about water storage in reservoirs, which is important for managing water resources and understanding the environment. We employed deep learning to reconstruct bathymetry for 7,250 global reservoirs, refining area-storage-depth relationshipsValidation against various data sources achieved a mean absolute error of 7.87 mThe method realized a 20.6% normalized root mean square error (NRMSE) for area-storage, surpassing prior methods
更多查看译文
关键词
deep learning,reservoir bathymetry,area-storage-depth,global reservoir
AI 理解论文
溯源树
样例
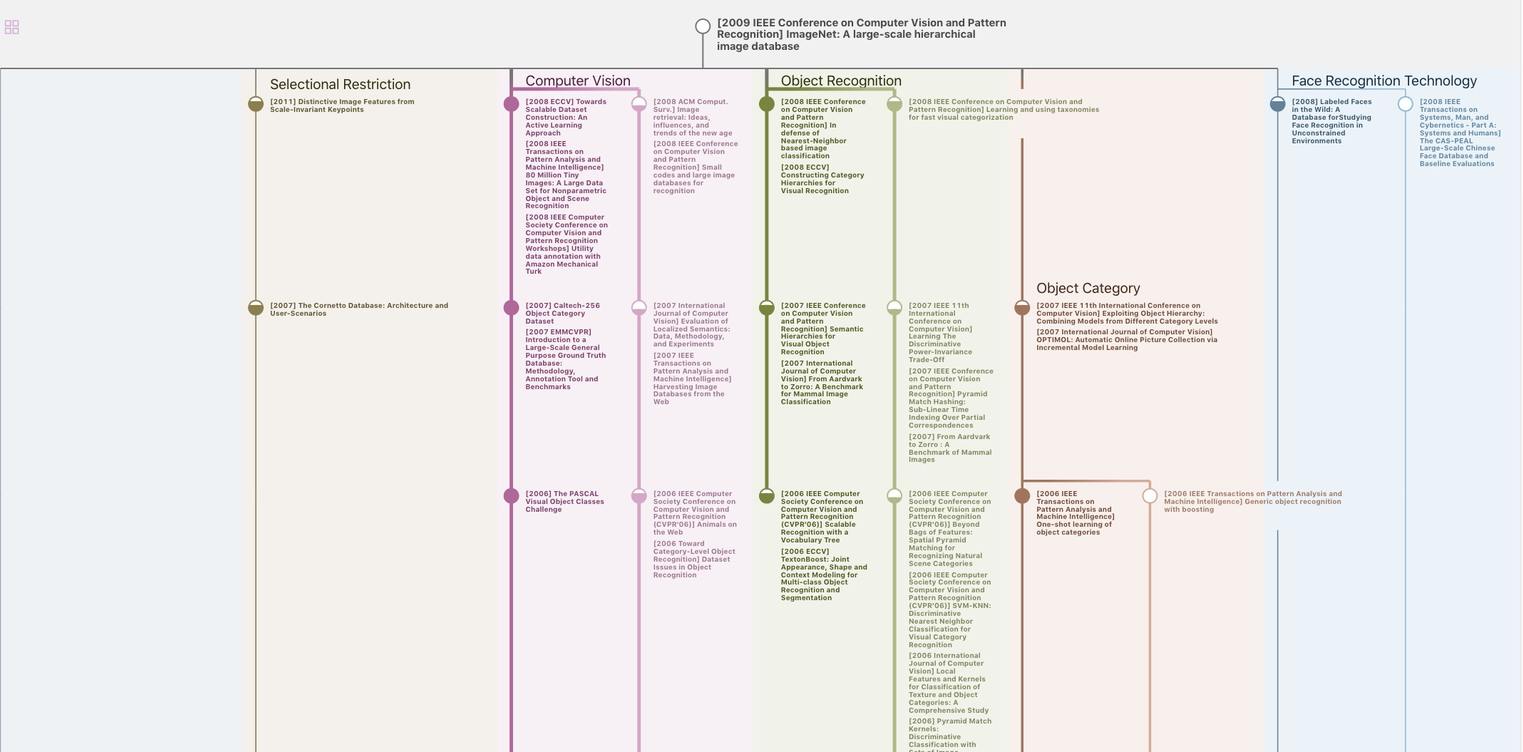
生成溯源树,研究论文发展脉络
Chat Paper
正在生成论文摘要