Bootstrap-Based Resampling Methods for Software Reliability Measurement Under Small Sample Condition
JOURNAL OF CIRCUITS SYSTEMS AND COMPUTERS(2023)
摘要
Highly reliable software systems rarely fail during tests because they are usually designed with fault-tolerant mechanisms and tested comprehensively. It is usually difficult to obtain sufficient failure data to carry out reliability measurements by using traditional software reliability models. These models are typically based on probabilistic statistics, and the measurement accuracy cannot be guaranteed with insufficient failure data. We propose a nonparametric bootstrap (NBP) resampling method and six parametric bootstrap (PB) resampling methods to construct software reliability models for small sample conditions based on commonly used models, i.e., the Jelinski-Moranda (J-M), Goel-Okumoto (G-O), Musa-Okumoto (M-O), Schneidewind, Duane and Littlewood-Verrall models. The bootstrap is a statistical procedure that resamples a single dataset to create many simulated samples. Our experimental results on fourteen failure datasets collected from industry and academia show that the proposed models improve by 10.2-18.0% failure time prediction accuracy, 24.7-30.7% curve fitting accuracy, and 7.7-42.9% reliability measurement accuracy compared with the original models. Furthermore, our approaches achieve 58.3-91.1% better failure time prediction accuracy in the case of small sample conditions compared to state-of-the-art machine learning and neural network-based methods. Overall, our approaches can perform more accurate reliability measurements than the original models even in scenarios with limited failure data.
更多查看译文
关键词
Software reliability,failure time prediction,small sample,bootstrap,resampling
AI 理解论文
溯源树
样例
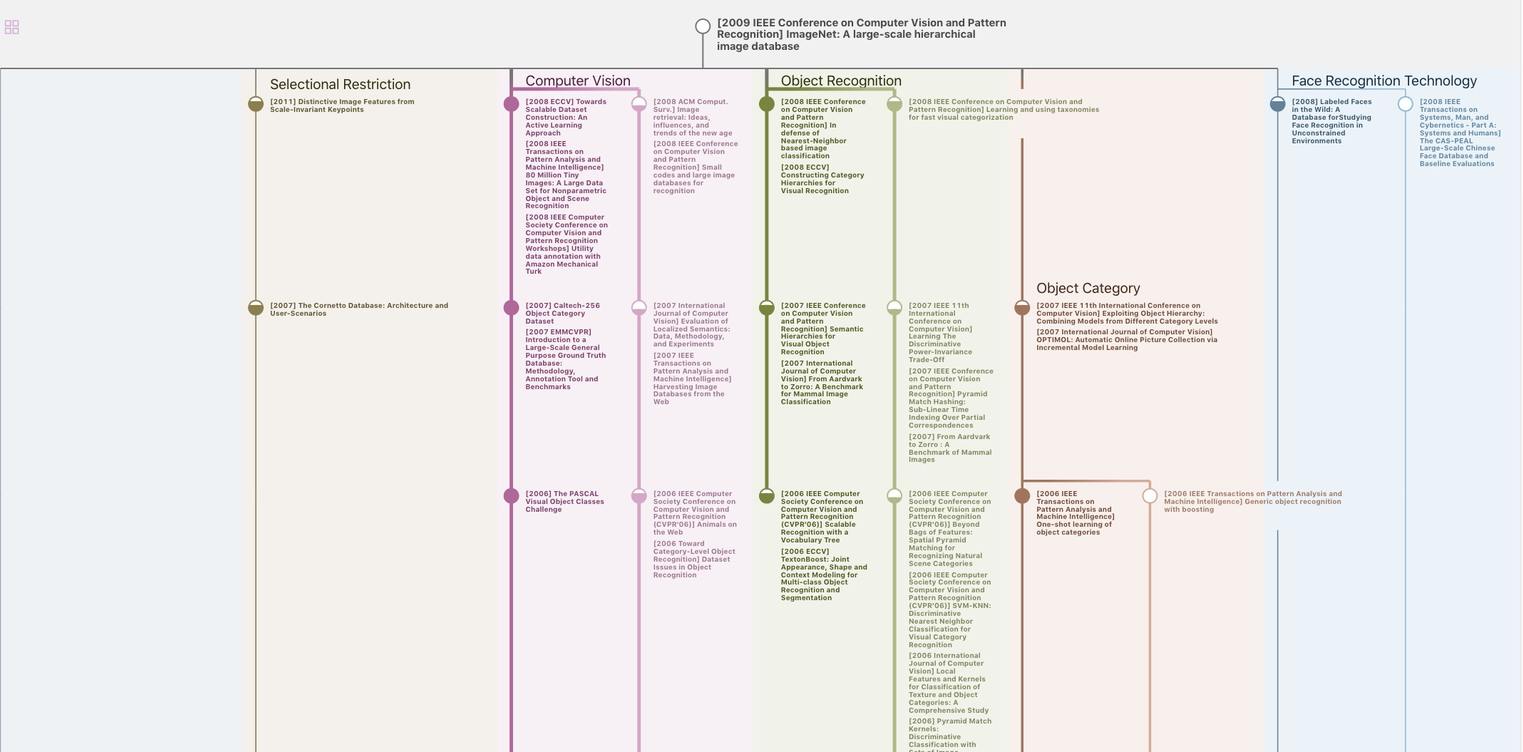
生成溯源树,研究论文发展脉络
Chat Paper
正在生成论文摘要