Real-Time Fault Tracking and Ground Motion Prediction for Large Earthquakes With HR-GNSS and Deep Learning
JOURNAL OF GEOPHYSICAL RESEARCH-SOLID EARTH(2023)
摘要
Earthquake early warning (EEW) systems aim to forecast the shaking intensity rapidly after an earthquake occurs and send warnings to affected areas before the onset of strong shaking. The system relies on rapid and accurate estimation of earthquake source parameters. However, it is known that source estimation for large ruptures in real-time is challenging, and it often leads to magnitude underestimation. In a previous study, we showed that machine learning, HR-GNSS, and realistic rupture synthetics can be used to reliably predict earthquake magnitude. This model, called Machine-Learning Assessed Rapid Geodetic Earthquake model (M-LARGE), can rapidly forecast large earthquake magnitudes with an accuracy of 99%. Here, we expand M-LARGE to predict centroid location and fault size, enabling the construction of the fault rupture extent for forecasting shaking intensity using existing ground motion models. We test our model in the Chilean Subduction Zone with thousands of simulated and five real large earthquakes. The result achieves an average warning time of 40.5 s for shaking intensity MMI4+, surpassing the 34 s obtained by a similar GNSS EEW model. Our approach addresses a critical gap in existing EEW systems for large earthquakes by demonstrating real-time fault tracking feasibility without saturation issues. This capability leads to timely and accurate ground motion forecasts and can support other methods, enhancing the overall effectiveness of EEW systems. Additionally, the ability to predict source parameters for real Chilean earthquakes implies that synthetic data, governed by our understanding of earthquake scaling, is consistent with the actual rupture processes. Earthquake Early Warning (EEW) systems can evaluate the shaking impact soon after an earthquake occurs and send alerts to areas where strong shaking has not yet arrived. However, predicting shaking intensity for large earthquakes face challenges such as magnitude underestimation and rupture complexity, leading to inaccurate prediction. In our previous study, we proposed a machine learning model, called M-LARGE, which can predict the magnitude of large earthquakes accurately and rapidly by utilizing surface deformation patterns recorded by HR-GNSS. Here, we expand the M-LARGE model to also predict the source location and fault size. This capability allows us to construct a fault, enabling forecasts of strong shaking 35-40 s before the shaking onset, longer than other similar methods. Our approach has the potential to improve the accuracy of EEW and reduce the impact of seismic hazards. Because the model is fully trained with simulated data, the successful prediction of real Chilean earthquakes indicates that our assumptions in simulating large earthquakes are sufficiently consistent with the rupture dynamics of actual events. We train a ML model that utilizes HR-GNSS data to predict source parameters and shaking intensity for large (M7.0+) earthquakesThe model yields a long median warning time of 35-40 s for MMI 4+ when tested on synthetic and real HR-GNSS recordsOur model fills a critical gap in current EEW systems for large events and can operate in parallel with other methods for better warnings
更多查看译文
关键词
earthquake early warning,deep learning,GNSS,ground motion prediction,fault tracking,rupture simulation
AI 理解论文
溯源树
样例
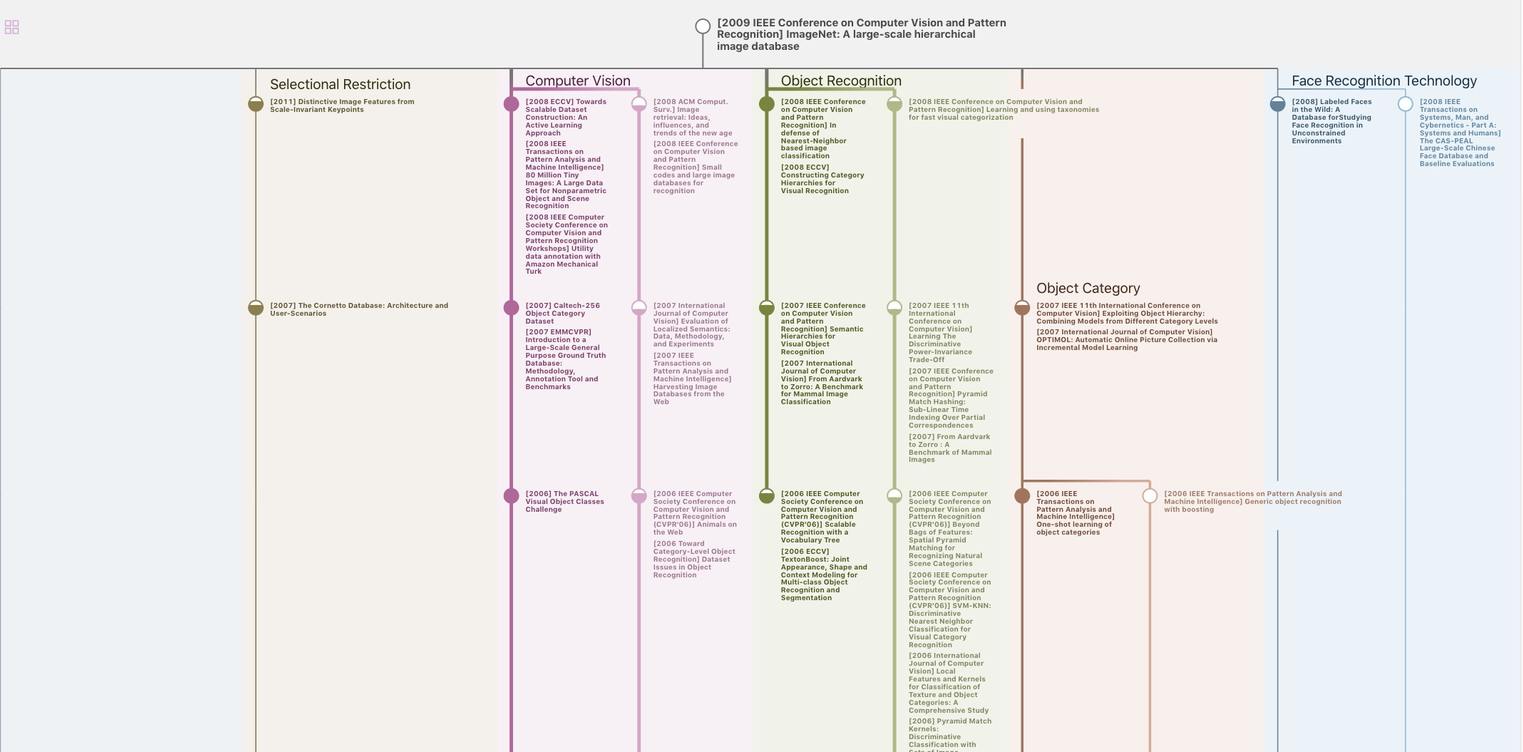
生成溯源树,研究论文发展脉络
Chat Paper
正在生成论文摘要