Simulation-based inference for reliability model selection and parameter calibration: An application to fatigue
QUALITY AND RELIABILITY ENGINEERING INTERNATIONAL(2024)
摘要
Selecting a "useful" model among a set of competing candidate models is a common problem in many disciplines and particularly in engineering applications. For a modeller, the best model fulfills a certain purpose best (e.g., the ability to accurately predict new data, good interpolation, precise estimates of lower/upper percentiles, etc.), which is typically assessed by comparing model simulations to the observed data. Approximate Bayesian computation (ABC) which is a statistical learning technique is used in this work for reliability model selection and parameter calibration using small/moderate fatigue life data sets. This is always the case in material fatigue due to the high cost and low efficiency of fatigue tests which are bottleneck problem when designing components/structures with regard to fatigue. The ABC is a likelihood-free based method which means that it needs only a generative model to simulate the data. The proposed method provides a formal rank of the competing reliability models by eliminating gradually the least likely models in a parsimonious manner. It is flexible since it offers the possibility to use different metrics to measure the discrepancy between simulated and observed data. The choice of the appropriate distance function depends essentially on the purpose of model selection. Through various examples, it has been demonstrated that the ABC method has a number of very attractive properties, which makes it especially useful in probabilistic risk assessment and reliability analysis.
更多查看译文
关键词
approximate Bayesian computation,fatigue life distributions,low percentiles,probabilistic fatigue design,reliability model selection,risk assessment,simulation-based inference
AI 理解论文
溯源树
样例
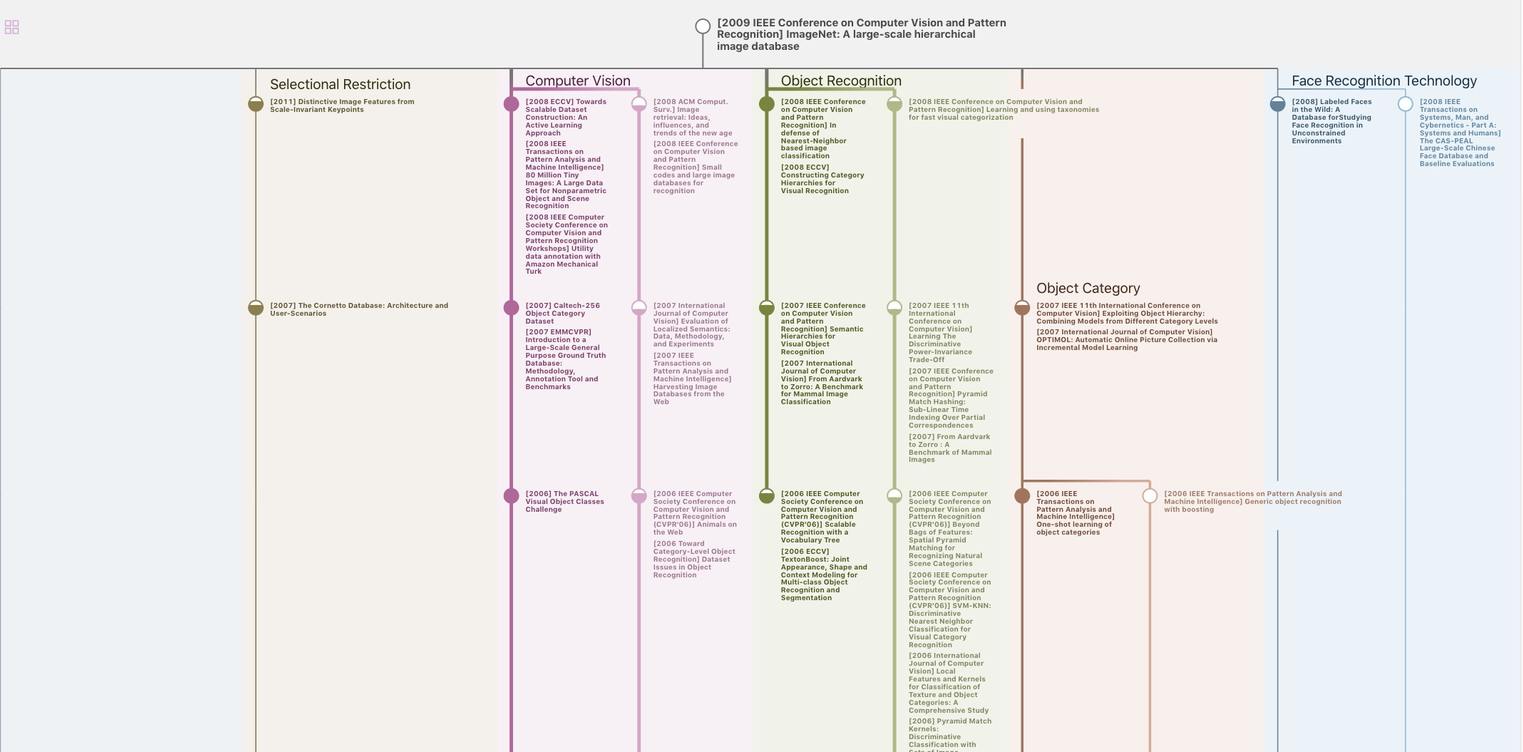
生成溯源树,研究论文发展脉络
Chat Paper
正在生成论文摘要