An acoustic emission onset time determination method based on Transformer
STRUCTURAL HEALTH MONITORING-AN INTERNATIONAL JOURNAL(2024)
摘要
Acoustic emission (AE) technology, as the main method of non-destructive testing technology, has been widely used in structural health monitoring (known as SHM) in the fields of machinery and civil engineering. Locating the failure source is an important application of SHM, and accurately identifying the moment when the AE signal first reaches the sensor (which is called onset time) is of vital importance. Deep learning model has been widely used in onset time determination of AE signals in recent years due to its powerful feature extraction ability. However, as one of the most popular models, Transformer has not been further studied in such field and its effectiveness remains to be proven. In this paper, a novel AE onset time determination method based on Transformer is proposed. Firstly, a preprocessing method based on segmentation-concatenation is applied to divide original data into several connected small segments, while the integrating labeling method is applied on small label segments. Secondly, the preprocessed data and labels are substituted into the Transformer model for training. Finally, for the sequence processed by the Transformer model, the first-time index that reaches the maximum value is obtained as the determination result. Based on the Hsu-Nielson source AE data, the feasibility and performance of this method are analyzed and compared with several commonly used methods: Akaike information criterion (AIC), short/long term average combined with AIC (STA/LTA-AIC), floating threshold (FT) and 1D-CNN-AIC method. The results show that the proposed method is significantly better than AIC, STA/LTA-AIC and FT. Moreover, the determination efficiency is greatly improved while the performance of the proposed method is close to that of 1D-CNN-AIC. Meanwhile, the method has robust performance especially in low signal-to-noise ratio scenario. In practical applications with small-scale data, the proposed method is of relatively high reference as well as application value.
更多查看译文
关键词
Onset time,acoustic emission,Transformer,low SNR
AI 理解论文
溯源树
样例
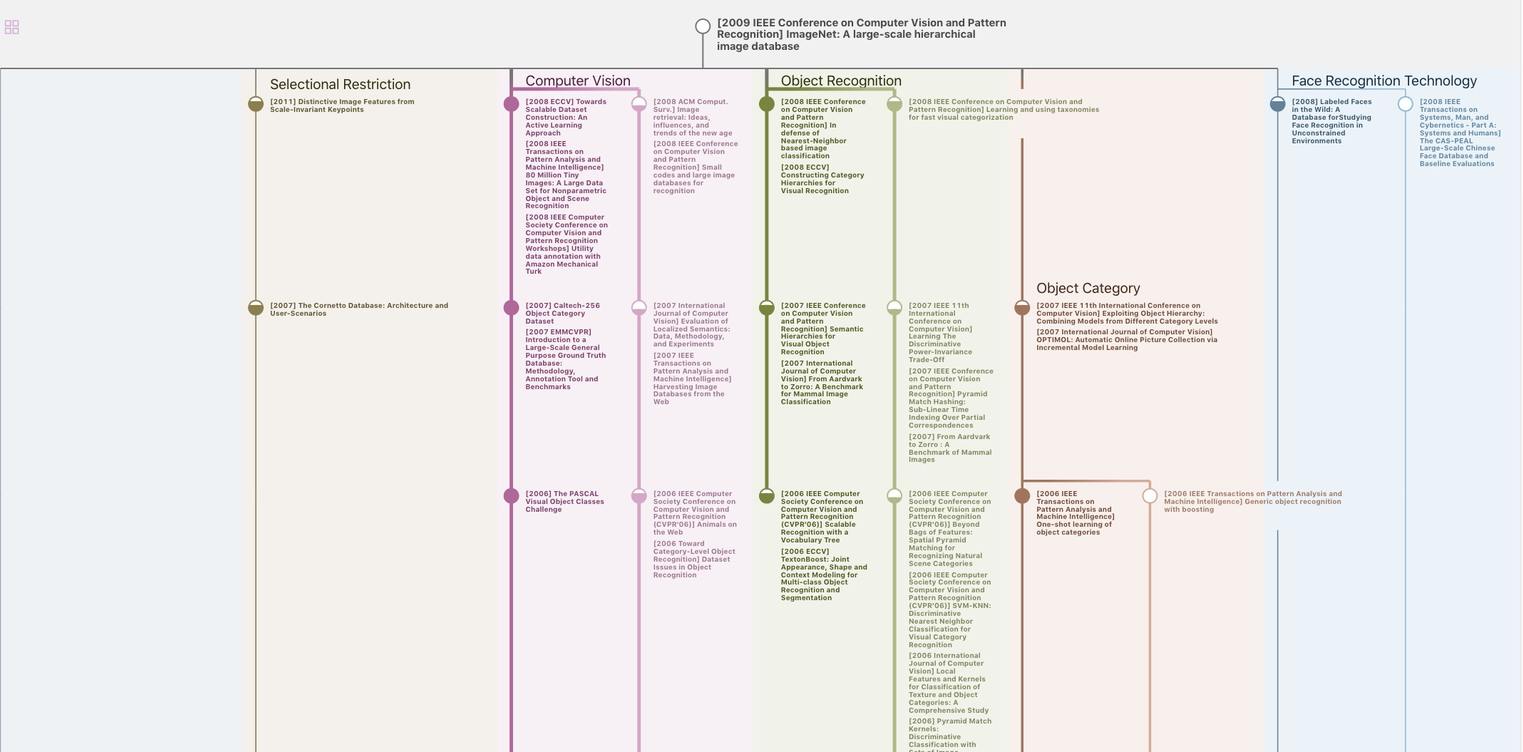
生成溯源树,研究论文发展脉络
Chat Paper
正在生成论文摘要