Optimizing Activation Functions and Hidden Neurons in Backpropagation Neural Networks for Real-Time NOx Concentration Prediction
ENERGY SOURCES PART A-RECOVERY UTILIZATION AND ENVIRONMENTAL EFFECTS(2024)
摘要
The Backpropagation (BP) neural network model is optimized in this research to predict NOx concentrations under dynamic conditions. Various activation functions (elliotsig, logsig, poslin, radbas, satlin, satlins, and tansig) were explored, and hidden neuron counts were adjusted to determine the optimal configuration for achieving maximal prediction accuracy. Out of the 8791 dataset entries, 7033 were used for training, and 1758 were used for testing. The model was evaluated using four primary metrics: Mean Absolute Error (MAE), Root Mean Square Error (RMSE), the coefficient of determination (R2), and Model Efficiency (ME). It was observed that activation functions had a significant impact on the computational time of the BP model. Satlins, poslin, and satlin functions operated swiftly, with a running time of approximately 11 ms for an ANN model with 100 neurons. Due to the computational complexity of logsig, tansig, and radbas, their computation time was more than three times longer compared to the previous functions. Overall, this research illustrates the effectiveness of the BP neural network in predicting NOx concentrations when optimized with the appropriate architecture. It highlights the elliotsig function for its combination of accuracy and efficiency. During a 10 ms operating time of the microcontroller, the combination of elliotsig function with 68 hidden neurons resulted in the highest R2 and ME values, achieving 0.9934 and 0.9861, respectively. Furthermore, this configuration demonstrated exceptional accuracy, with the lowest RMSE and MAE values recorded at 45.4654 and 23.2959, respectively.
更多查看译文
关键词
Activation functions,backpropagation (BP) neural network,Dynamic conditions,Emission monitoring and control,NOx concentrations prediction
AI 理解论文
溯源树
样例
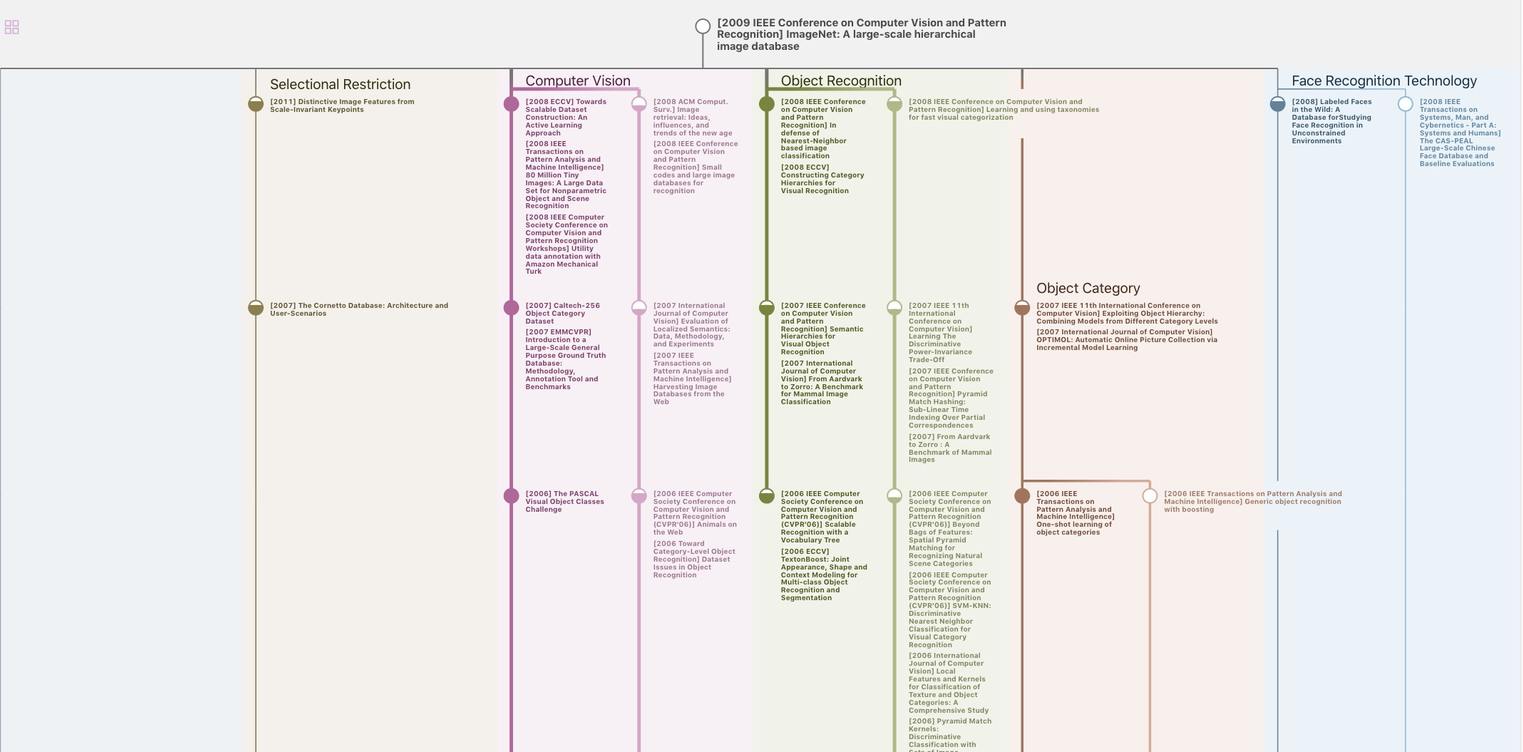
生成溯源树,研究论文发展脉络
Chat Paper
正在生成论文摘要