Privacy-Preserving Vertical Federated KNN Feature Imputation Method
Electronics(2024)
摘要
Federated learning stands as a pivotal component in the construction of data infrastructure. It significantly fortifies the safety and reliability of data circulation links, facilitating credible sharing and openness among diverse subjects. The presence of missing data poses a pervasive and challenging issue in the implementation of federated learning. Current research on imputation missing values predominantly concentrates on centralized methods and horizontal federation scenarios. However, there is a notable absence of exploration in the context of vertical federated application scenarios. In this paper, the problem of missing imputation in vertical federated learning is investigated and a novel vertical federated k-nearest neighbors (KNN) imputation method is proposed. Extensive experiments are conducted using publicly available data sets to compare existing imputation methods, the results demonstrate the effectiveness and progress of our approach.
更多查看译文
关键词
federated learning,missing data,vertical federated imputation,k-nearest neighbors
AI 理解论文
溯源树
样例
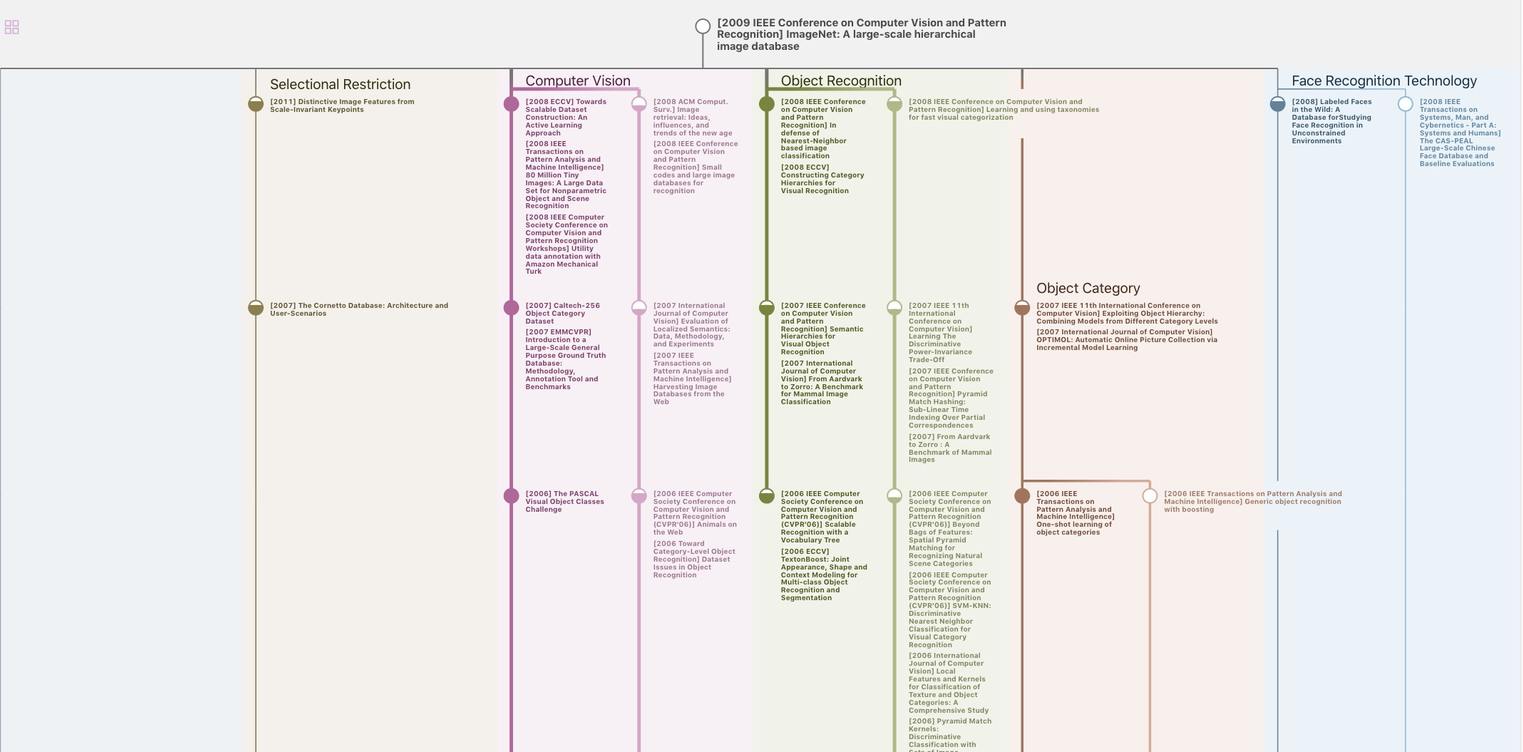
生成溯源树,研究论文发展脉络
Chat Paper
正在生成论文摘要