A brain tumour classification on the magnetic resonance images using convolutional neural network based privacy-preserving federated learning
INTERNATIONAL JOURNAL OF IMAGING SYSTEMS AND TECHNOLOGY(2024)
摘要
The healthcare industry has found it challenging to build a powerful global classification model due to the scarcity and diversity of medical data. The leading cause is privacy, which restricts data sharing among healthcare providers. Federated learning (FL) can contribute to developing classification models by protecting data privacy. This study has tested various federated techniques in a peer-to-peer setting to classify brain Magnetic Resonance Images (MRI). The authors propose various aggregation strategies for FL, including Federated Averaging (FedAvg), Quantum FL with FedAVG (QFedAvg) and Fault Tolerant FedAvg (Ft-FedAvg) and FedAvg with differential privacy (Dp-FedAvg). In each approach, a custom Convolutional Neural Network (CNN) model is applied to compute locally run nodes with different parts of the same brain MRI dataset for 10, 20 and 30 training and test rounds. A central server and CNN-based three federated clients are included in the FL-based brain tumour classification model to exchange data and combine the model weights on the server, which are sent from local devices to the server. The superiority of the performance of the proposed model is demonstrated by comparing it with traditional methods on various performance metrics. Experimental results show that in brain MRI dataset classification using FL approaches, FedAVg showed the best performance with 85.55% and 84.60% success for 10 and 20 rounds, respectively, while Ft-FedAvg showed the best performance with 85.80% success for 30 rounds for test set. Statistical results obtained from FL approaches showed that FedAvg and Ft-FedAvg have superior performance with regard to accuracy and robustness in comparison with the others.
更多查看译文
关键词
classification,deep learning,federated learning,privacy-preserving
AI 理解论文
溯源树
样例
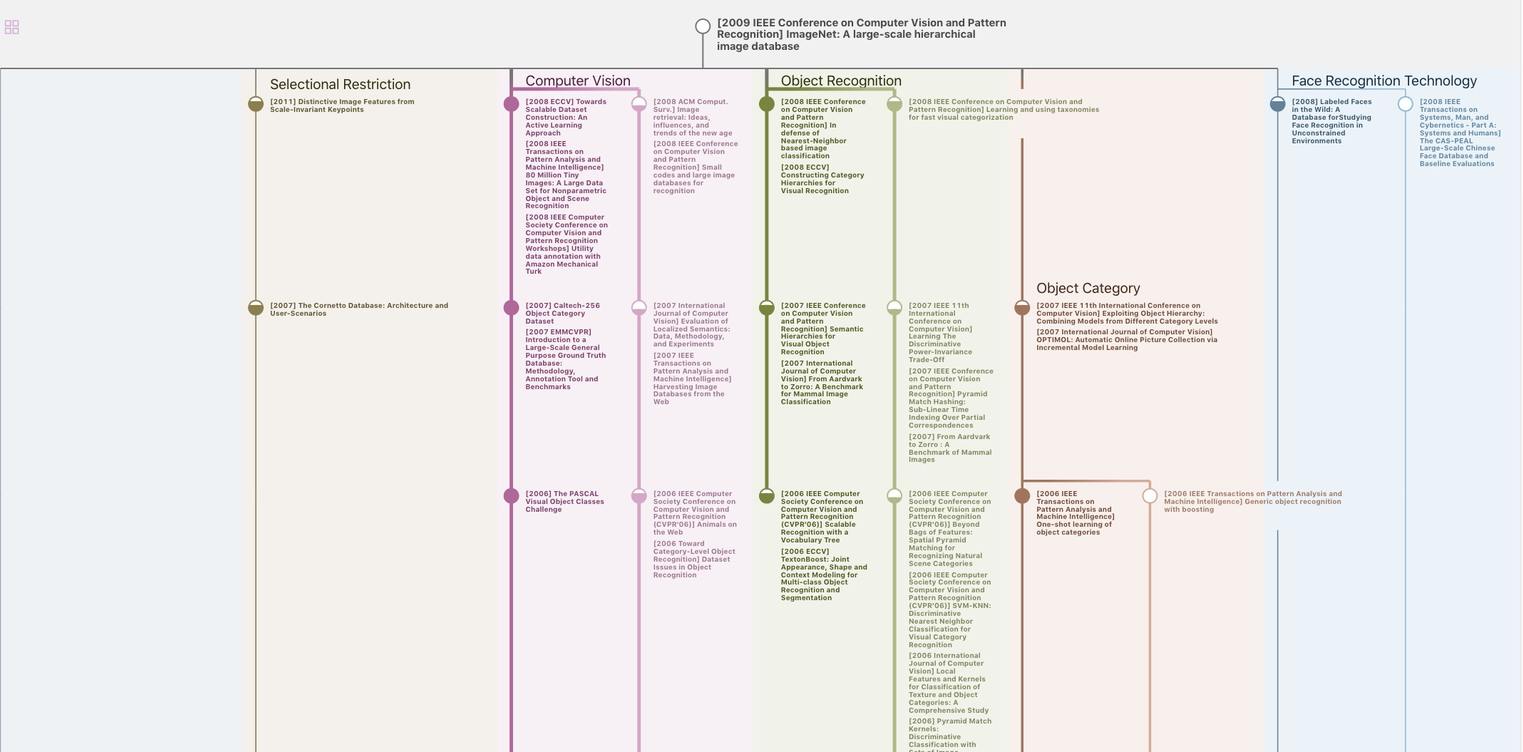
生成溯源树,研究论文发展脉络
Chat Paper
正在生成论文摘要