Hierarchical spiking neural network auditory feature based dry-type transformer fault diagnosis using convolutional neural network
MEASUREMENT SCIENCE AND TECHNOLOGY(2024)
摘要
Dry-type transformer fault diagnosis (DTTFD) presents a significant challenge because of its complex internal structure and sensitivity to noise. To address this challenge, we propose a DTTFD method that combines hierarchical spike neural network auditory features (HSNNAF) with convolutional neural networks (CNN). By leveraging the hierarchical structure of the central auditory system and sequential nonlinear feature extraction to compute the HSNNAF, we enhanced the relevant clues of transformer faults while removing non-fault source noise. Subsequently, the obtained HSNNAF were fed into a CNN for fault classification. The proposed method demonstrated high accuracy in DTTFD, with a diagnostic accuracy of 99.52%. Even at a signal-to-noise ratio of 0 dB, the diagnostic accuracy remains as high as 95.88%. These results indicate that the method can accurately diagnose faults in dry-type transformers while exhibiting excellent noise resistance capabilities.
更多查看译文
关键词
dry-type transformers,fault diagnosis,auditory feature,auditory model,convolutional neural network
AI 理解论文
溯源树
样例
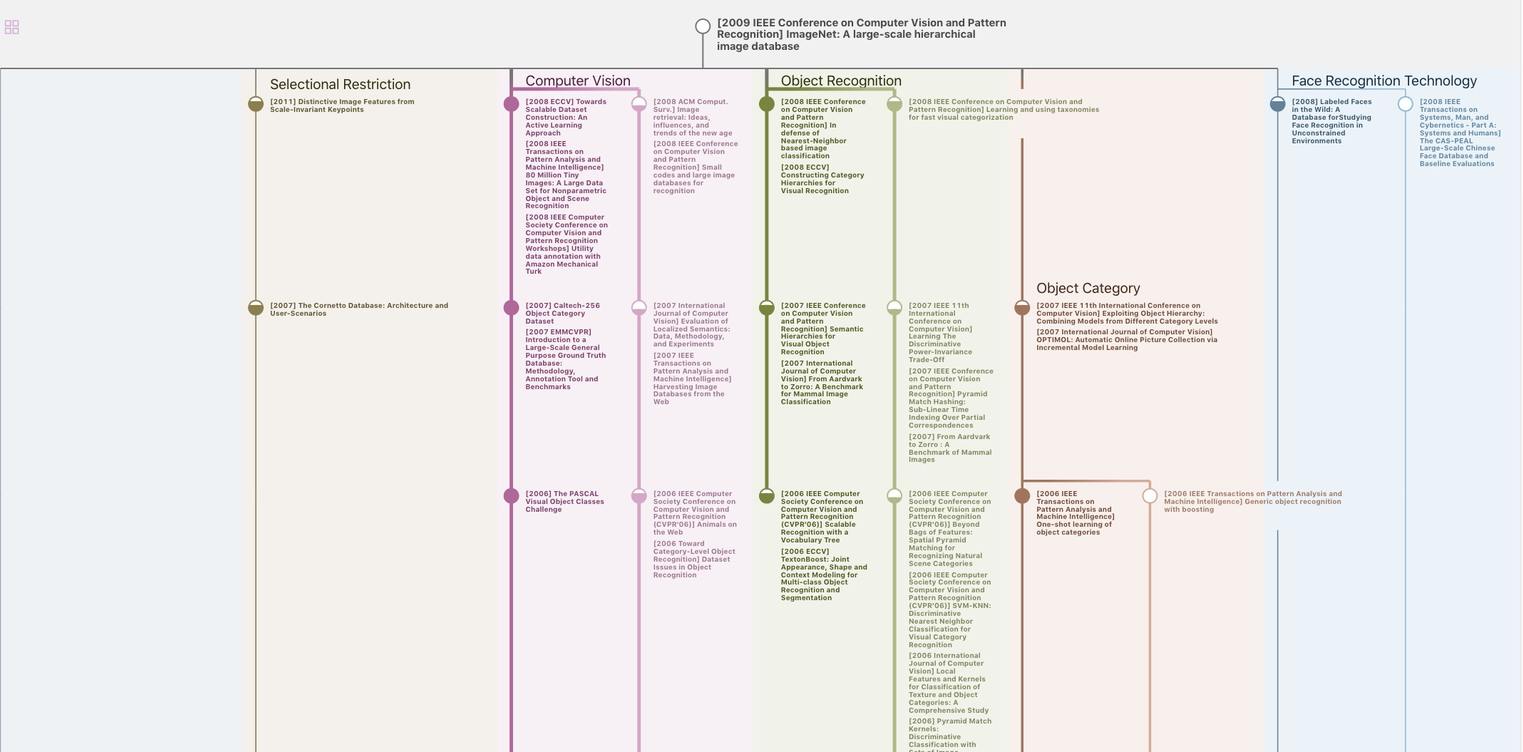
生成溯源树,研究论文发展脉络
Chat Paper
正在生成论文摘要