RoeNet: Predicting discontinuity of hyperbolic systems from continuous data
INTERNATIONAL JOURNAL FOR NUMERICAL METHODS IN ENGINEERING(2024)
摘要
Predicting future discontinuous phenomena that are unobservable from training data sets has long been a challenging problem in scientific machine learning. We introduce a novel paradigm to predict the emergence and evolution of various discontinuities of hyperbolic partial differential equations (PDEs) based on given training data over a short window with limited discontinuity information. Our method is inspired by the classical Roe solver [P. L. Roe, J Comput Phys., vol. 43, 1981], a basic tool for simulating various hyperbolic PDEs in computational physics. By carefully designing the computing primitives, the data flow, and the novel pseudoinverse processing module, we enable our data-driven predictor to satisfy all the essential mathematical criteria of a Roe solver and hence deliver accurate predictions of hyperbolic PDEs. We demonstrate through various examples that our data-driven Roe predictor outperforms original human-designed Roe solver and deep neural networks with weak priors in terms of accuracy and robustness.
更多查看译文
关键词
conservation law,machine learning,physics-informed neural network,Roe solve
AI 理解论文
溯源树
样例
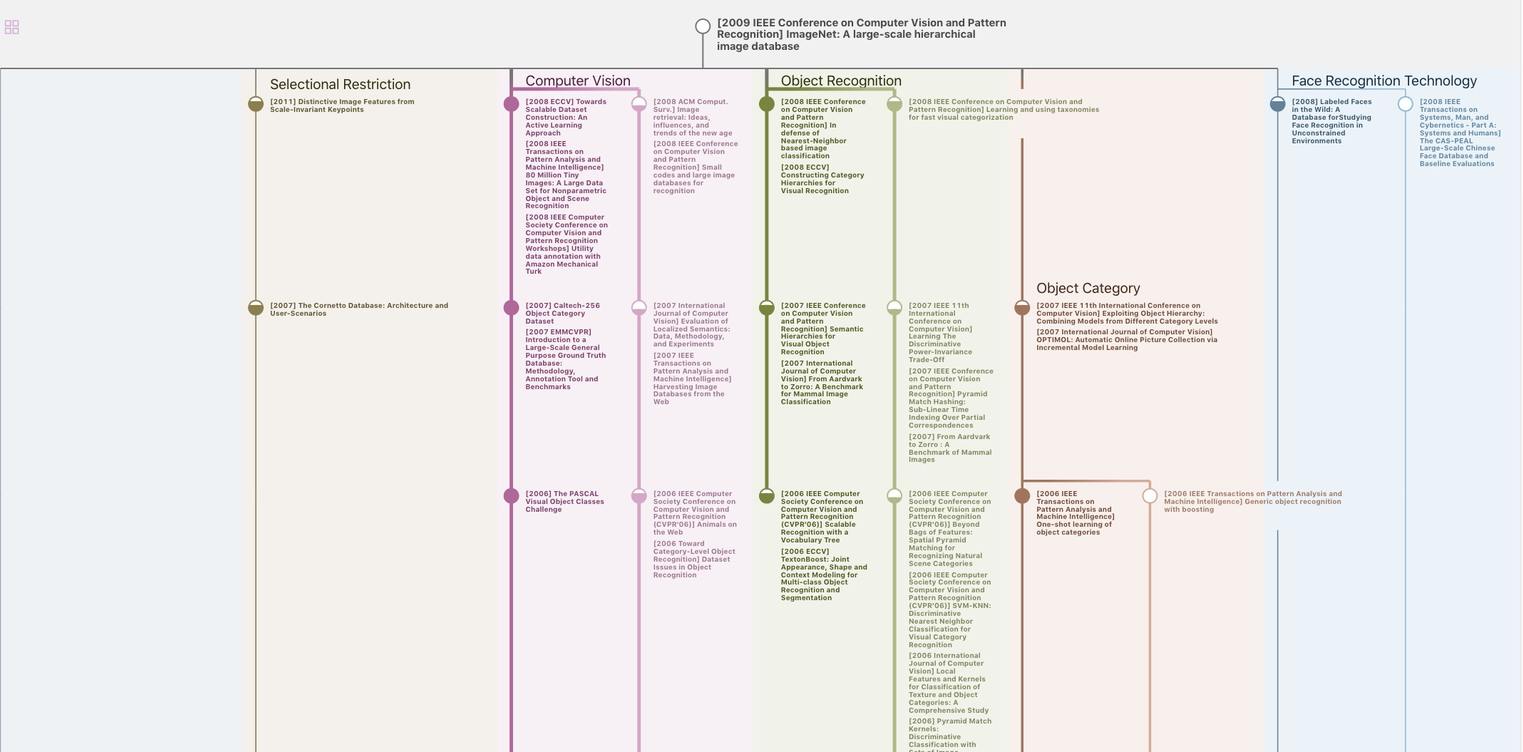
生成溯源树,研究论文发展脉络
Chat Paper
正在生成论文摘要