Investigation on the effect of wavelet mutation and opposition learning on slime mould algorithm
ENGINEERING RESEARCH EXPRESS(2024)
摘要
The study focuses on a hypothesis-based critical analysis of proposed modifications to the slime mould algorithm. The modifications being investigated are opposition learning and wavelet mutation. These modifications help the slime mould algorithm to avoid local optima for better exploration of the search space. The goal is to assess the effectiveness of these modifications in improving the performance of slime mould algorithm. Both, the basic slime mould algorithm, and the proposed variant eventually achieve the desired convergence. However, to compare the efficiency of the two algorithms, the study introduces a measurement index called the 'swiftness' of the algorithm. This index quantifies the speed at which an algorithm reaches convergence. It is calculated as the area under the convergence curve of each algorithm. The rationale behind this approach is that visual inspection alone may not be sufficient to discriminate between the algorithms based on the convergence curve. The hybrid approach, incorporating opposition learning and wavelet mutation, is evaluated statistically using the CEC 2008 benchmark function. Additionally, the study examines small and medium single-objective power dispatch optimization problems that adhere to non-convex system limitations. By assessing the performance of the modified slime mould algorithm on these different problem domains, the researchers aim to provide a comprehensive analysis of its effectiveness.
更多查看译文
关键词
optimization techniques,economic load dispatch,hypothetical signed rank test,slime mould algorithm,opposition learning,wavelet mutation
AI 理解论文
溯源树
样例
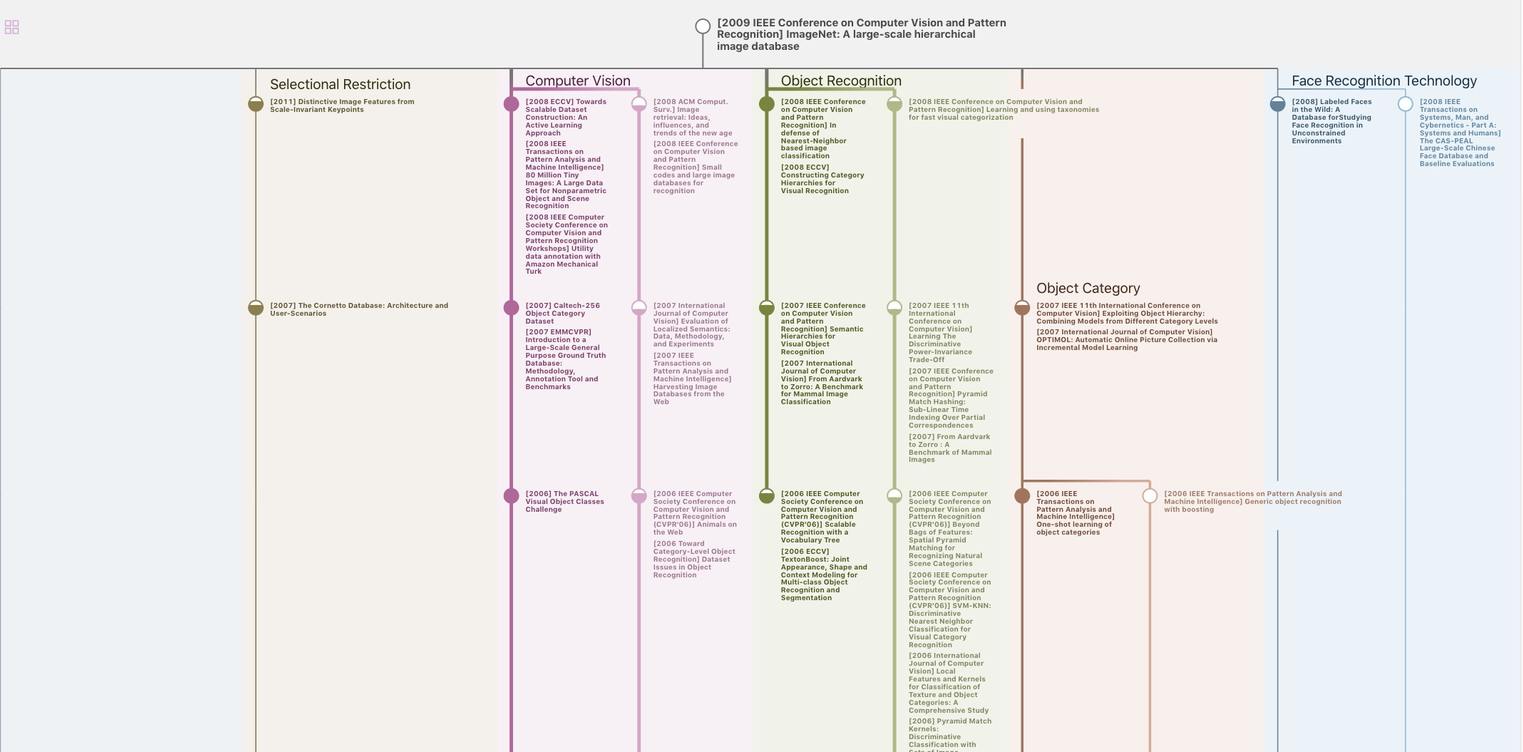
生成溯源树,研究论文发展脉络
Chat Paper
正在生成论文摘要