Adaptive partial least squares estimation addressing heteroscedasticity and multicollinearity: a Monte Carlo simulation evidence
COMMUNICATIONS IN STATISTICS-SIMULATION AND COMPUTATION(2023)
摘要
In this article, we proposed an adaptive partial least squares (APLS) estimator when a high dimensional or multivariate linear regression model has the problems of multicollinearity and heteroscedastic errors. The APLS estimator works as a dimension reduction tool which makes the design matrix orthogonalized and gives more efficient estimates when the errors are heteroscedastic. Moreover, the standard errors of the estimates are also proposed by using bootstrapping for both partial least squares and APLS. The mean squared error criterion is used for the comparison of PLS and APLS estimates by using the Monte Carlo simulations. An illustrative example from a real-life data is also presented. The results suggested that the APLS is the more reliable choice over the PLS estimator.
更多查看译文
关键词
Adaptive estimator,Bootstrap,Kernel estimate,Partial least square (PLS),Standard errors
AI 理解论文
溯源树
样例
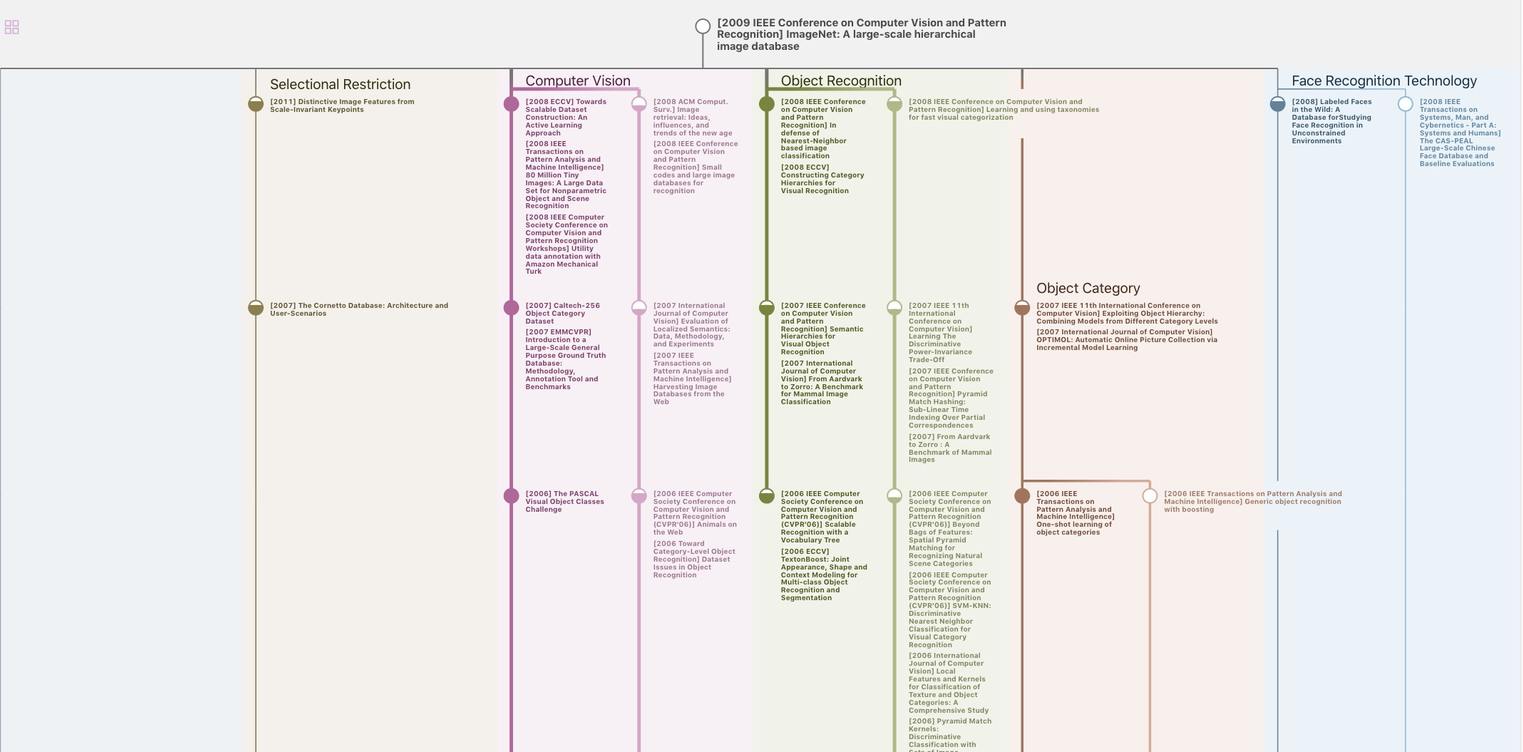
生成溯源树,研究论文发展脉络
Chat Paper
正在生成论文摘要