Clustering spatial functional data using a geographically weighted Dirichlet process
CANADIAN JOURNAL OF STATISTICS-REVUE CANADIENNE DE STATISTIQUE(2024)
摘要
We propose a Bayesian nonparametric clustering approach to study the spatial heterogeneity effect for functional data observed at spatially correlated locations. We consider a geographically weighted Chinese restaurant process equipped with a conditional autoregressive prior to capture fully the spatial correlation of function curves. To sample efficiently from our model, we customize a prior called Quadratic Gamma, which ensures conjugacy. We design a Markov chain Monte Carlo algorithm to infer simultaneously the posterior distributions of the number of groups and the grouping configurations. The superior numerical performance of the proposed method over competing methods is demonstrated using simulated examples and a U.S. annual precipitation study. Dans ce travail, les auteurs presentent une approche de regroupement non parametrique bayesienne pour examiner l'heterogeneite spatiale dans des donnees fonctionnelles observees a des sites correles. Leur methode s'appuie sur un processus du restaurant chinois pondere geographiquement, complete par un a priori autoregressif conditionnel, pour capturer la correlation spatiale des courbes fonctionnelles. Afin d'echantillonner efficacement dans ce cadre, ils introduisent un a priori Gamma quadratique qui garantit la propriete de conjugaison. Ils proposent egalement un algorithme de Monte-Carlo par chaines de Markov pour estimer les lois a posteriori du nombre de groupes et de leurs configurations. A travers des simulations et une etude sur les precipitations annuelles aux etats-Unis, ils demontrent que la performance de leur methode est souvent superieure a celle d'approches alternatives.
更多查看译文
关键词
Bayesian nonparametric method,functional data,geographical weights,Markov chain Monte Carlo,spatial clustering
AI 理解论文
溯源树
样例
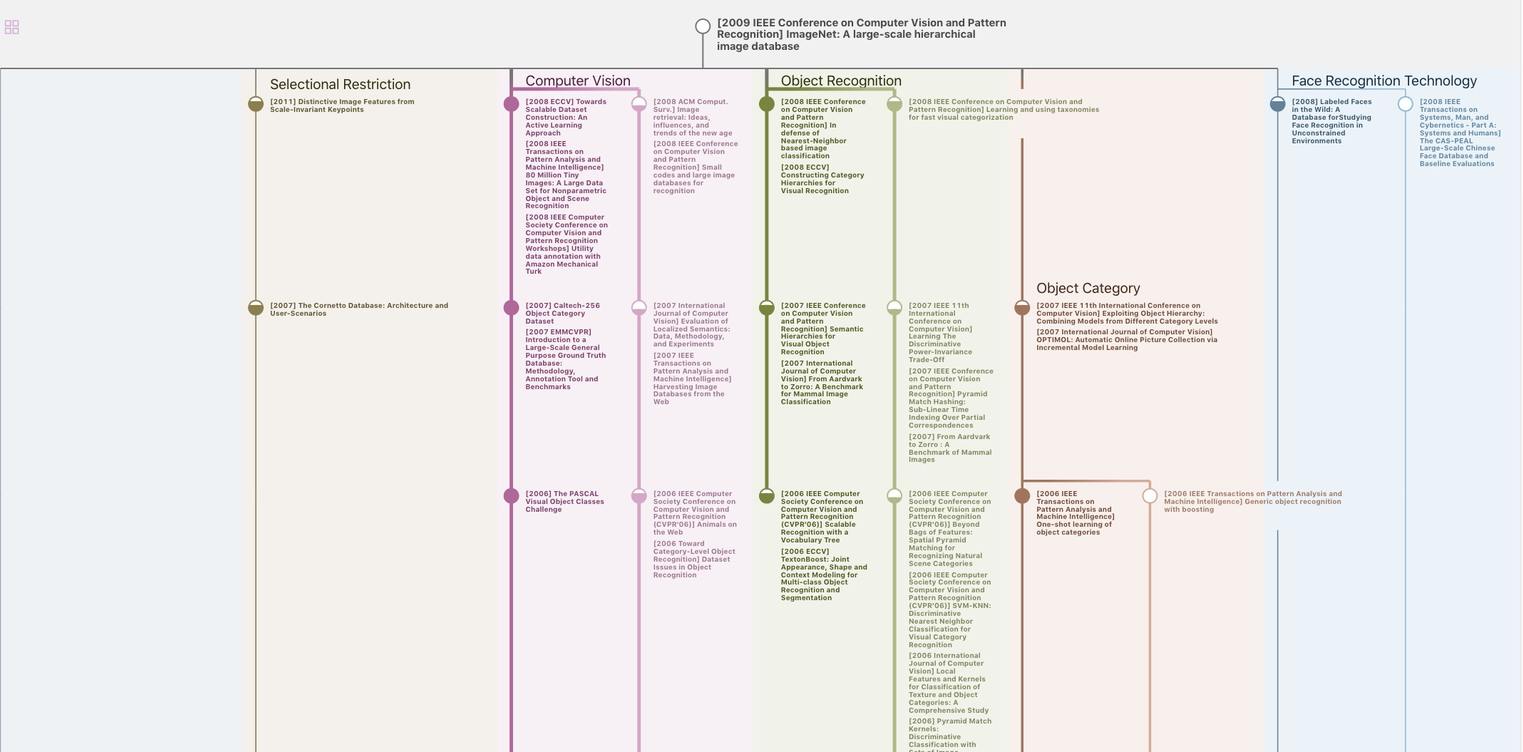
生成溯源树,研究论文发展脉络
Chat Paper
正在生成论文摘要