Optimising digital signal processor-based defect detection in smart manufacturing with lightweight convolutional neural networks
IET COLLABORATIVE INTELLIGENT MANUFACTURING(2024)
摘要
Industrial defect detection is an important part of intelligent manufacturing, and Internet of things (IoT)-based defect detection is receiving more and more attention. Although deep learning (DL) can help defect detection reduce the cost and improve the accuracy of traditional manual quality inspection, DL requires huge computational resources and is difficult to be simply deployed on IoT devices with limited computational power and memory resources. Digital signal processor (DSP) is an important IoT device with small size, high performance and low energy consumption, which has been widely used in intelligent manufacturing. In order to perform accurate defect detection on DSP, the authors proposed various optimisation strategies and then used a parallel scheme to scale the model to execute on multiple cores. The authors' method evaluated it on Northeastern University Surface Defect Dataset, Magnetic Tile Defect Dataset, Rail Surface Defect Dataset and Silk Cylinder Defect Dataset, and the experimental results showed that the authors' method obtains faster speeds without accuracy loss compared to running the same Convolutional Neural Networks model on a mainstream desktop CPU. This means that the authors' method can realise efficient and accurate defect detection on IoT devices with limited computational power and memory resources, which opens up new possibilities for future development in the field of smart manufacturing. A lightweight network model that can be deployed to digital signal processor is proposed to enable industrial defect detection for smart manufacturing.image
更多查看译文
关键词
manufacturing industries,manufacturing systems,mobile computing
AI 理解论文
溯源树
样例
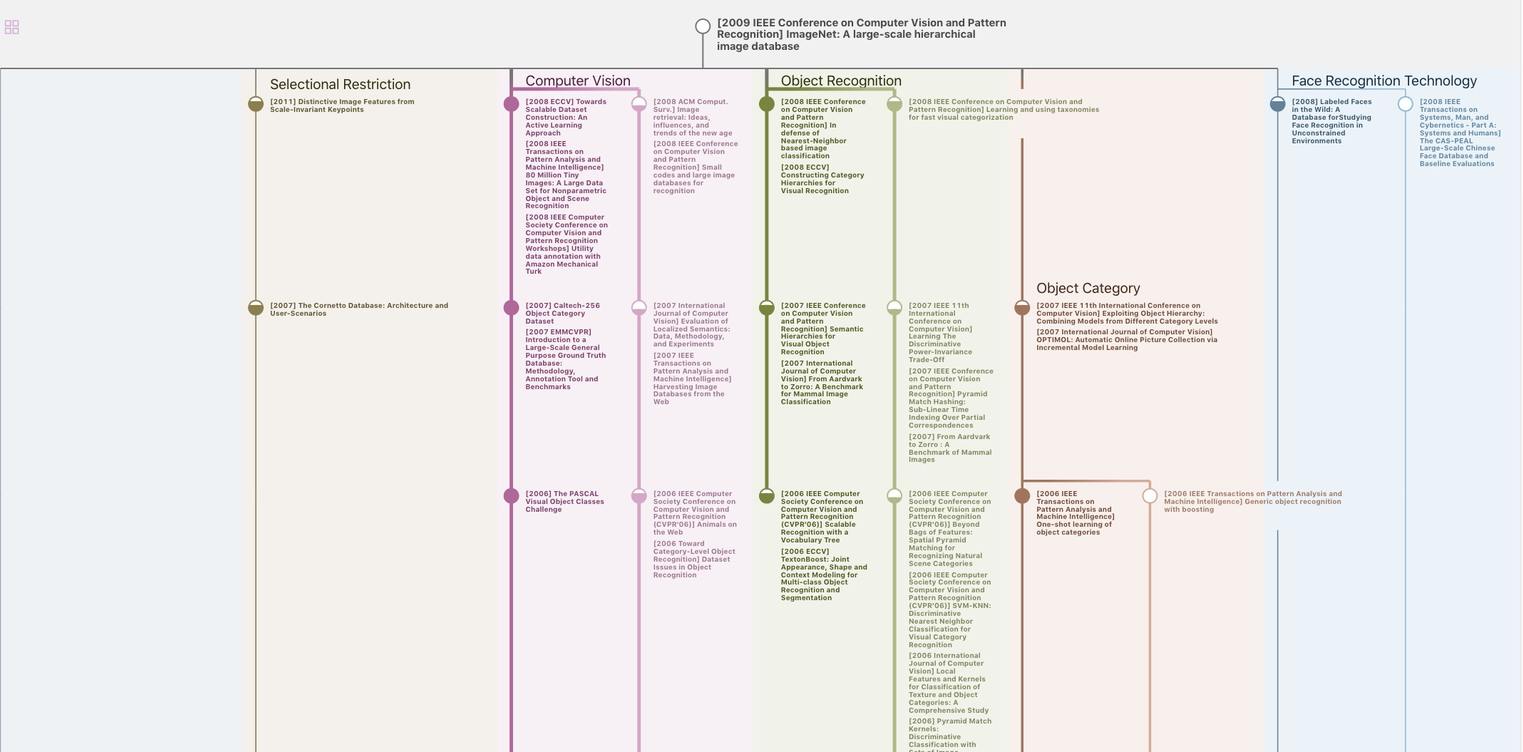
生成溯源树,研究论文发展脉络
Chat Paper
正在生成论文摘要