Length-scale effect on the hardness of metallic/ceramic multilayered composites: A machine learning prediction
SCRIPTA MATERIALIA(2024)
摘要
The length-scale effect on the hardness of Al/Si3N4 multilayered composites were investigated via combined experimental and machine learning approaches. It was found that the hardness decreases with increase of the individual layer thickness, in agreement with the classic Hall-Petch relation. However, the hardness decrease became moderate when the individual layer thickness was less than 80 nm. The individual critical layer thickness of metallic/ceramic multilayered composites for the change in deformation mechanism was obtained by machine learning methods. The prediction results indicated that the individual critical transition layer thickness was mainly determined by the metal layer, and the corresponding critical transition hardness was positively correlated with the elastic modulus of the ceramic layer. Importantly, it was confirmed that the hardness of other metallic/ceramic multilayered composite systems could be predicted by the machine learning approach, especially the optimized random forest regression (RFR) model.
更多查看译文
关键词
Machine learning,Individual critical layer thickness,Multilayered composites,Hardness prediction,Hall-Petch relation
AI 理解论文
溯源树
样例
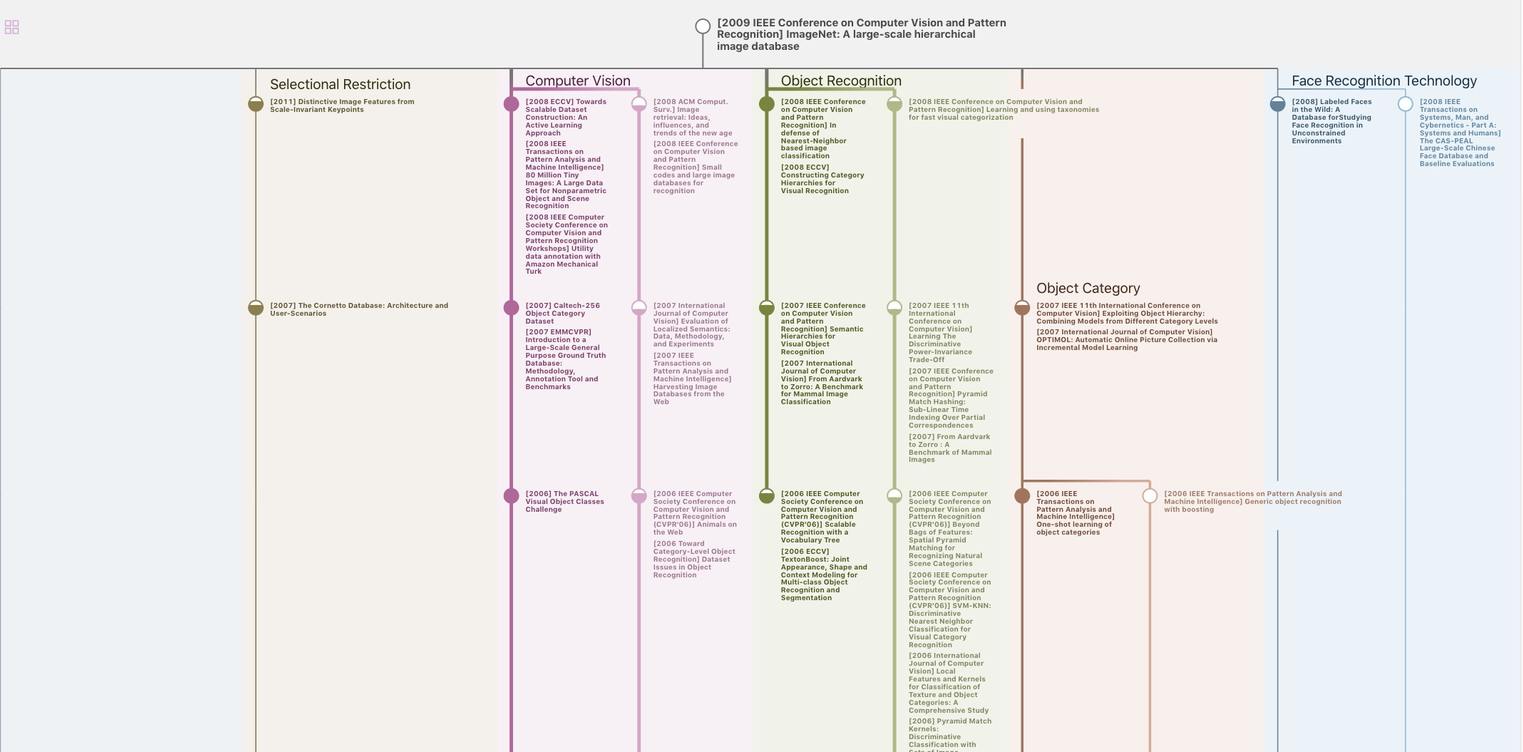
生成溯源树,研究论文发展脉络
Chat Paper
正在生成论文摘要