PKD-Net: Distillation of prior knowledge for image completion by multi-level semantic attention
CONCURRENCY AND COMPUTATION-PRACTICE & EXPERIENCE(2024)
摘要
Prior knowledge plays a crucial role in image completion. Although almost all of the existing image completion methods use prior knowledge to complete the image to be repaired from different perspectives, the learning and modeling of the prior knowledge is still a challenging problem. In order to address this issue, we propose a novel prior knowledge distillation framework (PKD-Net) which could distill prior knowledge of structure and style from multiple semantic space and generates not only plausible content but also consistent style with surrounding image area. Our PKD-Net replaces the skip connection in the vanilla U-Net with a semantic shift attention module. The semantic shift attention module takes features from encoder layer and those from decoder layer as input pairs to output shifted features which take into account the long-range dependency of encoder layer features and corresponding decoder layer features from the perspective of local structure and style. Semantic shift attention module models the global interdependencies in local spatial structures (patches centered at each position) and style (appearance texture) dimensions respectively, which could implement distillation of prior knowledge from two aspects: structure and style. Experiments on multiple datasets including faces (CelebA, CelebA-HQ) and natural images (ImageNet, Places2, Paris Street View) demonstrate that our proposed approach generates higher quality completion results than existing ones.
更多查看译文
关键词
deep learning,image completion,knowledge distillation,semantic attention
AI 理解论文
溯源树
样例
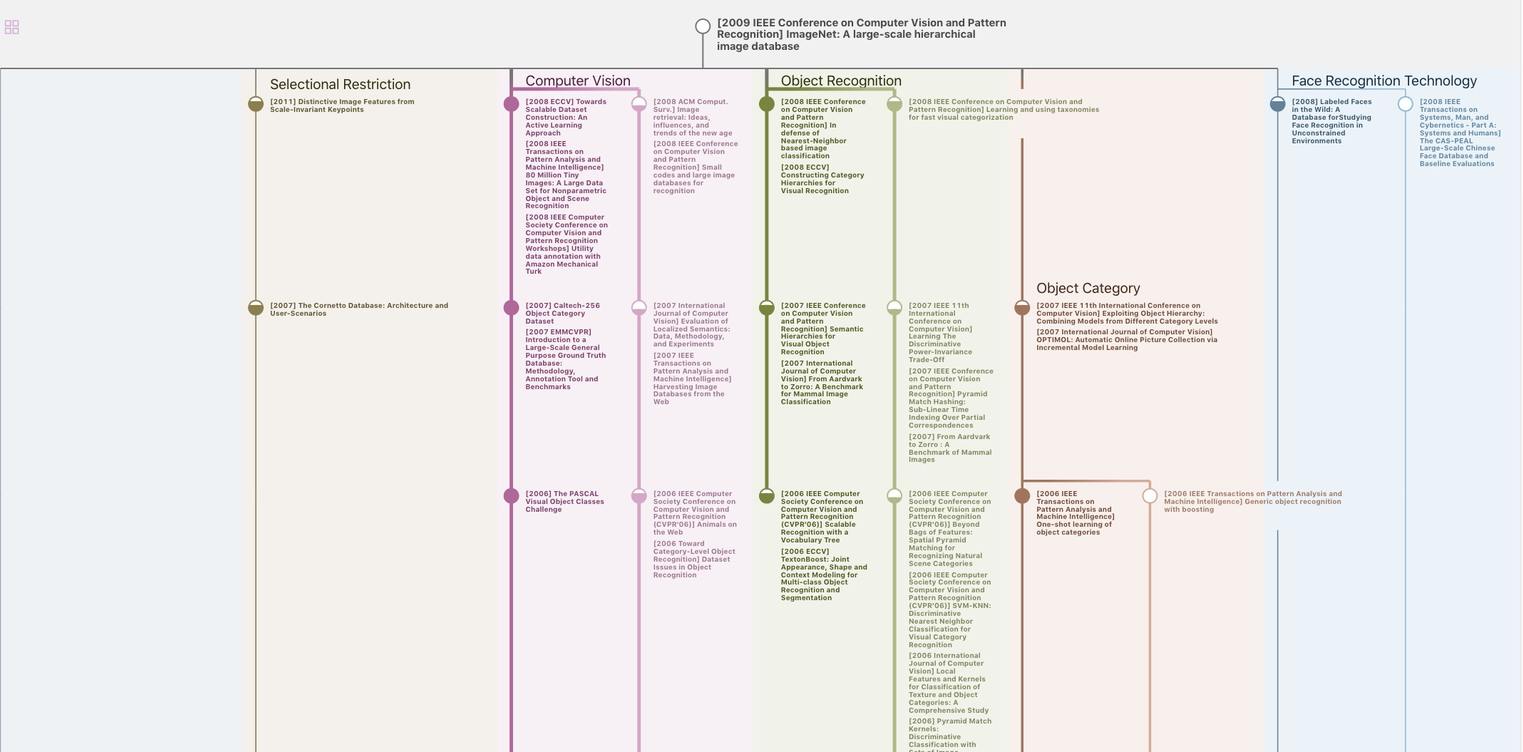
生成溯源树,研究论文发展脉络
Chat Paper
正在生成论文摘要