Dimension Reduction for Frchet Regression
JOURNAL OF THE AMERICAN STATISTICAL ASSOCIATION(2023)
摘要
With the rapid development of data collection techniques, complex data objects that are not in the Euclidean space are frequently encountered in new statistical applications. Frechet regression model (Petersen and Muller) provides a promising framework for regression analysis with metric space-valued responses. In this article, we introduce a flexible sufficient dimension reduction (SDR) method for Frechet regression to achieve two purposes: to mitigate the curse of dimensionality caused by high-dimensional predictors, and to provide a visual inspection tool for Frechet regression. Our approach is flexible enough to turn any existing SDR method for Euclidean (X, Y) into one for Euclidean X and metric space-valued Y. The basic idea is to first map the metric space-valued random object Y to a real-valued random variable f(Y) using a class of functions, and then perform classical SDR to the transformed data. If the class of functions is sufficiently rich, then we are guaranteed to uncover the Frechet SDR space. We showed that such a class, which we call an ensemble, can be generated by a universal kernel (cc-universal kernel). We established the consistency and asymptotic convergence rate of the proposed methods. The finite-sample performance of the proposed methods is illustrated through simulation studies for several commonly encountered metric spaces that include Wasserstein space, the space of symmetric positive definite matrices, and the sphere. We illustrated the data visualization aspect of our method by the human mortality distribution data from the United Nations Databases. Supplementary materials for this article are available online.
更多查看译文
关键词
Ensembled sufficient dimension reduction,Inverse regression,Statistical objects,Universal kernel,Wasserstein space
AI 理解论文
溯源树
样例
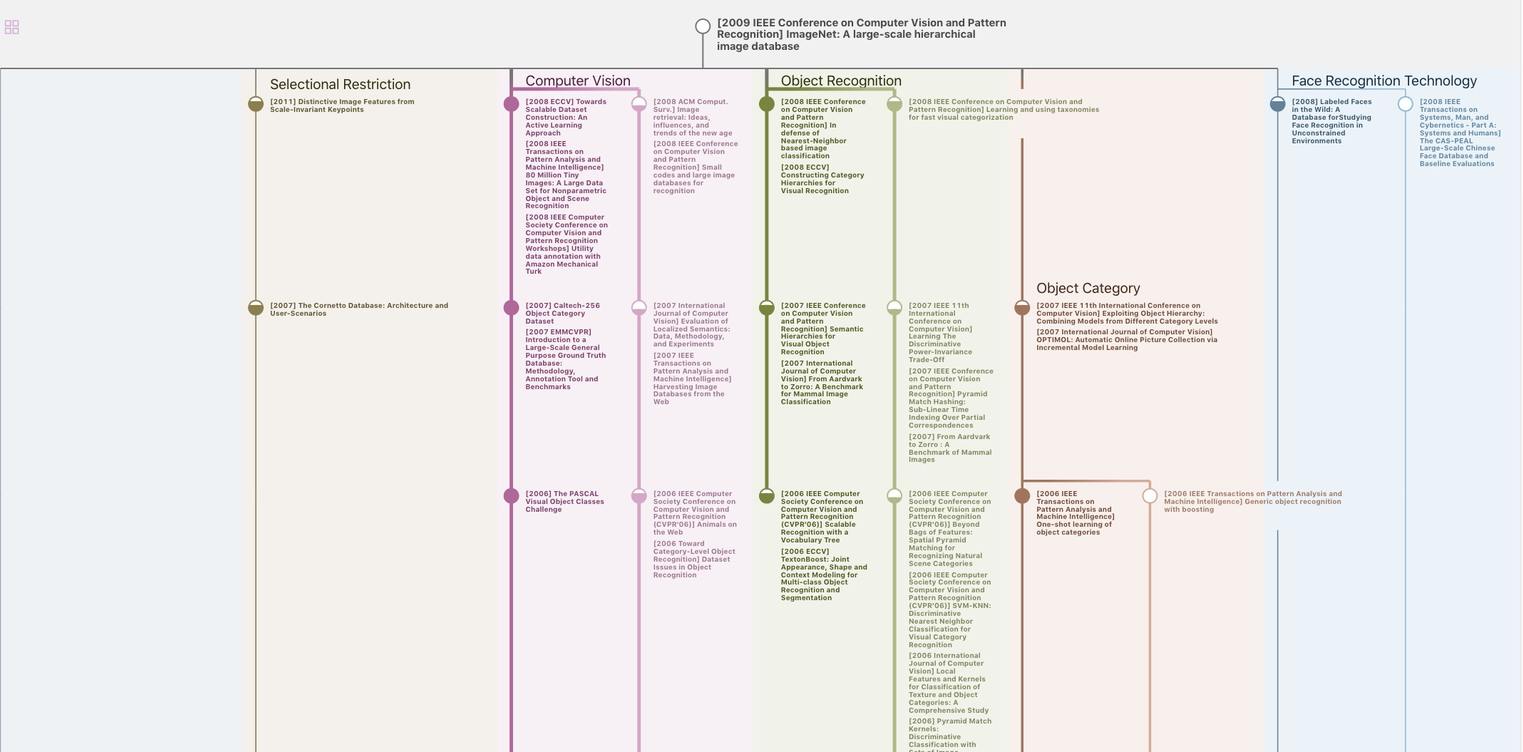
生成溯源树,研究论文发展脉络
Chat Paper
正在生成论文摘要