Enhanced Atrous Extractor and Self-Dynamic Gate Network for Superpixel Segmentation
APPLIED SCIENCES-BASEL(2023)
摘要
A superpixel is a group of pixels with similar low-level and mid-level properties, which can be seen as a basic unit in the pre-processing of remote sensing images. Therefore, superpixel segmentation can reduce the computation cost largely. However, all the deep-learning-based methods still suffer from the under-segmentation and low compactness problem of remote sensing images. To fix the problem, we propose EAGNet, an enhanced atrous extractor and self-dynamic gate network. The enhanced atrous extractor is used to extract the multi-scale superpixel feature with contextual information. The multi-scale superpixel feature with contextual information can solve the low compactness effectively. The self-dynamic gate network introduces the gating and dynamic mechanisms to inject detailed information, which solves the under-segmentation effectively. Massive experiments have shown that our EAGNet can achieve the state-of-the-art performance between k-means and deep-learning-based methods. Our methods achieved 97.61 in ASA and 18.85 in CO on the BSDS500. Furthermore, we also conduct the experiment on the remote sensing dataset to show the generalization of our EAGNet in remote sensing fields.
更多查看译文
关键词
superpixel segmentation,gating mechanism,multi-scale superpixel feature
AI 理解论文
溯源树
样例
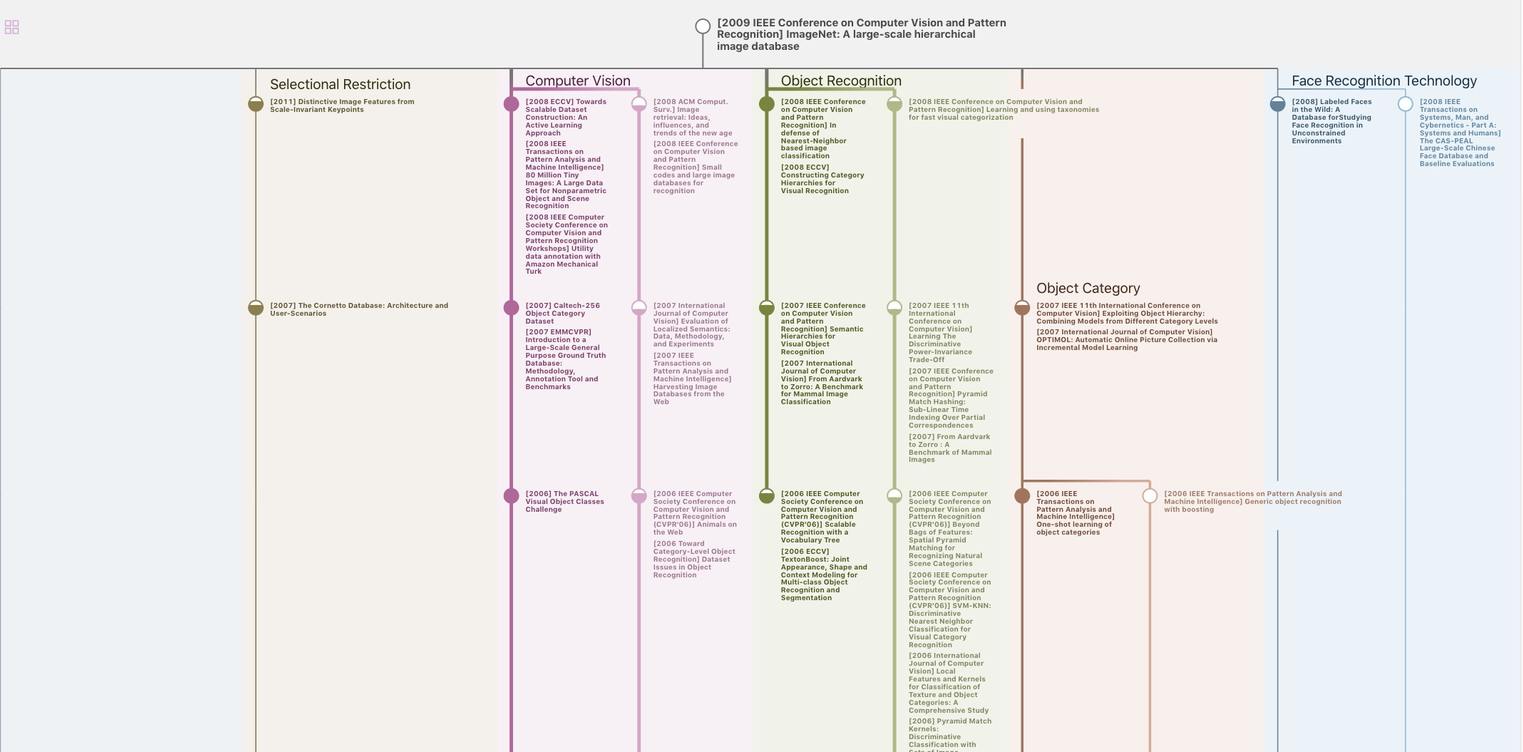
生成溯源树,研究论文发展脉络
Chat Paper
正在生成论文摘要