Prediction Equilibrium for Dynamic Network Flows
JOURNAL OF MACHINE LEARNING RESEARCH(2023)
摘要
We study a dynamic traffic assignment model, where agents base their instantaneous rout-ing decisions on real-time delay predictions. We formulate a mathematically concise model and define dynamic prediction equilibrium (DPE) in which no agent can at any point dur-ing their journey improve their predicted travel time by switching to a different route. We demonstrate the versatility of our framework by showing that it subsumes the well-known full information and instantaneous information models, in addition to admitting further realistic predictors as special cases. We then proceed to derive properties of the predictors that ensure a dynamic prediction equilibrium exists. Additionally, we define epsilon-approximate DPE wherein no agent can improve their predicted travel time by more than epsilon and pro -vide further conditions of the predictors under which such an approximate equilibrium can be computed. Finally, we complement our theoretical analysis by an experimental study, in which we systematically compare the induced average travel times of different predic-tors, including two machine-learning based models trained on data gained from previously computed approximate equilibrium flows, both on synthetic and real world road networks.
更多查看译文
关键词
Dynamic Traffic Assignment,Dynamic Flow,Prediction Equilibrium,Machine-Learning,Regression Predictors
AI 理解论文
溯源树
样例
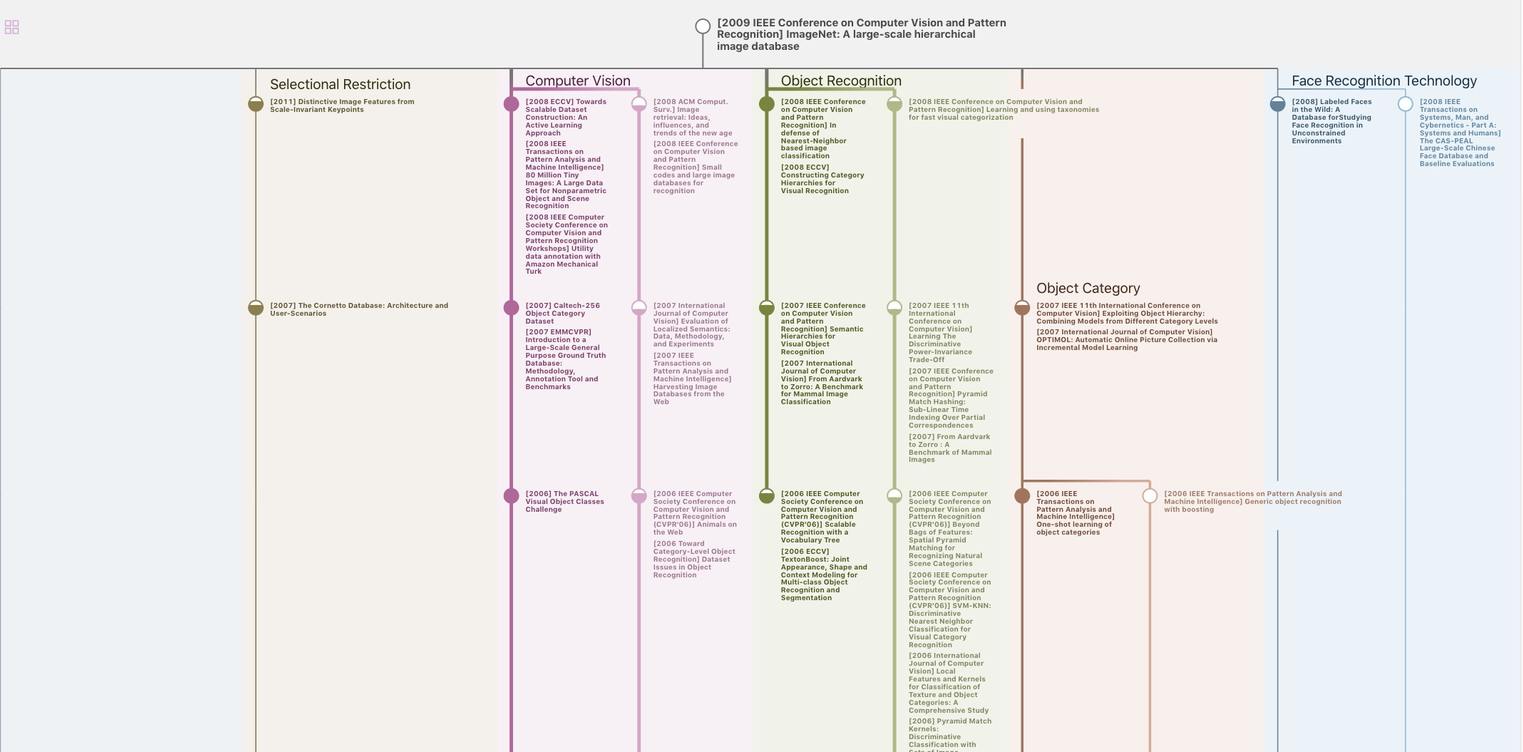
生成溯源树,研究论文发展脉络
Chat Paper
正在生成论文摘要