Online Non-stochastic Control with Partial Feedback
JOURNAL OF MACHINE LEARNING RESEARCH(2023)
摘要
Online control with non-stochastic disturbances and adversarially chosen convex cost functions, referred to as online non-stochastic control, has recently attracted increasing attention. We study online non-stochastic control with partial feedback, where learners can only access partially observed states and partially informed (bandit) costs. The problem setting arises naturally in real-world decision-making applications and strictly generalizes exceptional cases studied disparately by previous works. We propose the first online algorithm for this problem, with an Oe(T3/4) regret competing with the best policy in hindsight, where T denotes the time horizon and the Oe(center dot)-notation omits the poly-logarithmic factors in T. To further enhance the algorithms' robustness to changing environments, we then design a novel method with a two-layer structure to optimize the dynamic regret, a more challenging measure that competes with time-varying policies. Our method is based on the online ensemble framework by treating the controller above as the base learner. On top of that, we design two different meta-combiners to simultaneously handle the unknown variation of environments and the memory issue arising from the online control. We prove that the two resulting algorithms enjoy Oe(T3/4(1 + PT )1/2) and Oe(T3/4(1 + PT )1/4 +T5/6) dynamic regret respectively, where PT measures the environmental non-stationarity. Our results are further extended to unknown transition matrices. Finally, empirical studies in both synthetic linear and simulated nonlinear tasks validate our method's effectiveness, thus supporting the theoretical findings. semble, online learning with memory, bandit convex optimization
更多查看译文
关键词
online non-stochastic control,partial feedback,dynamic regret,online en-semble,online learning with memory,bandit convex optimization
AI 理解论文
溯源树
样例
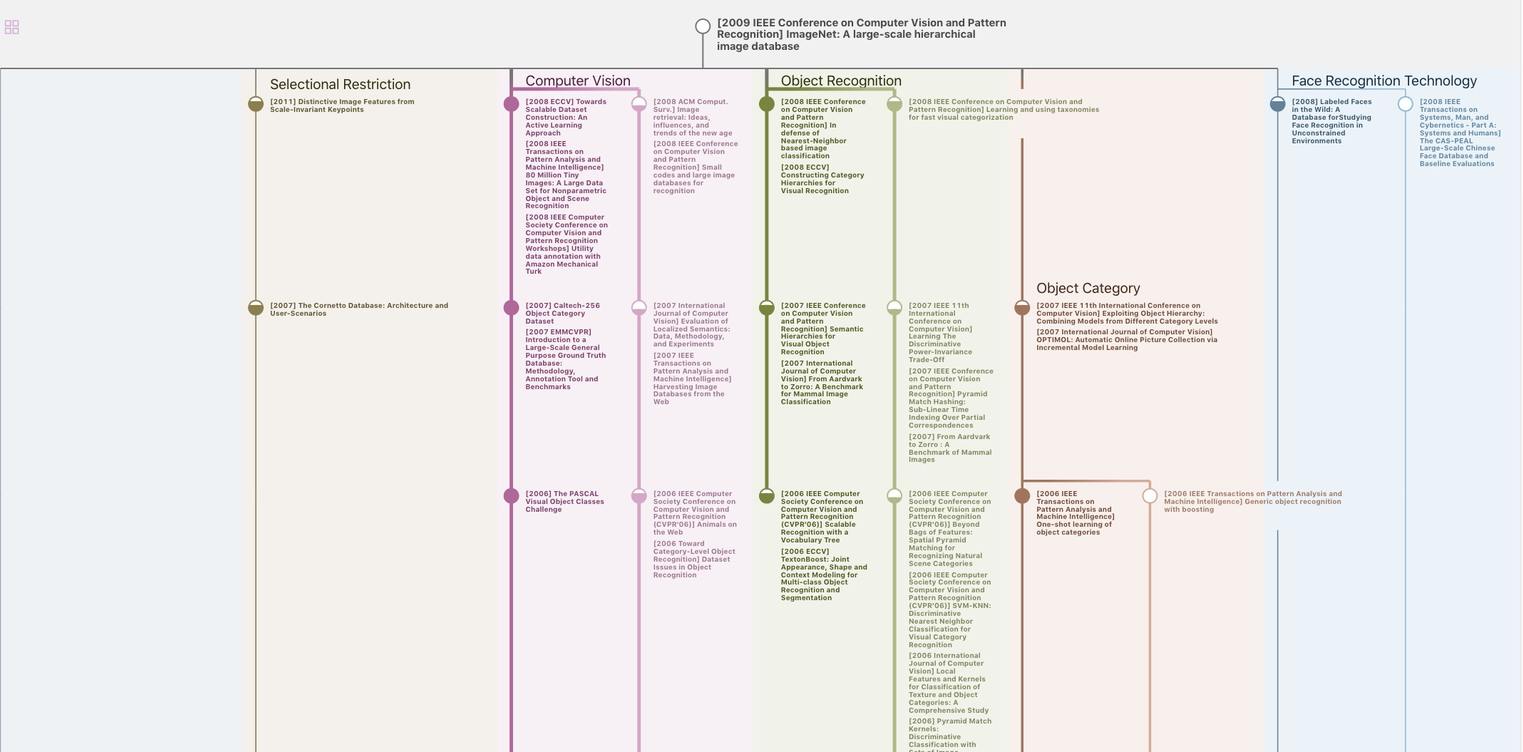
生成溯源树,研究论文发展脉络
Chat Paper
正在生成论文摘要