18F-FDG PET/CT-based deep learning radiomics predicts 5-years disease-free survival after failure to achieve pathologic complete response to neoadjuvant chemotherapy in breast cancer
EJNMMI RESEARCH(2023)
摘要
Background This study aimed to assess whether a combined model incorporating radiomic and depth features extracted from PET/CT can predict disease-free survival (DFS) in patients who failed to achieve pathologic complete response (pCR) after neoadjuvant chemotherapy.Results This study retrospectively included one hundred and five non-pCR patients. After a median follow-up of 71 months, 15 and 7 patients experienced recurrence and death, respectively. The primary tumor volume underwent feature extraction, yielding a total of 3644 radiomic features and 4096 depth features. The modeling procedure employed Cox regression for feature selection and utilized Cox proportional-hazards models to make predictions on DFS. Time-dependent receiver operating characteristic (ROC) curves and the area under the ROC curve (AUC) were utilized to evaluate and compare the predictive performance of different models. 2 clinical features (RCB, cT), 4 radiomic features, and 7 depth features were significant predictors of DFS and were included to develop models. The integrated model incorporating RCB, cT, and radiomic and depth features extracted from PET/CT images exhibited the highest accuracy for predicting 5-year DFS in the training (AUC 0.943) and the validation cohort (AUC 0.938).Conclusion The integrated model combining radiomic and depth features extracted from PET/CT images can accurately predict 5-year DFS in non-pCR patients. It can help identify patients with a high risk of recurrence and strengthen adjuvant therapy to improve survival.
更多查看译文
关键词
Breast cancer,Deep learning,Radiomics,PET/CT,Neoadjuvant chemotherapy
AI 理解论文
溯源树
样例
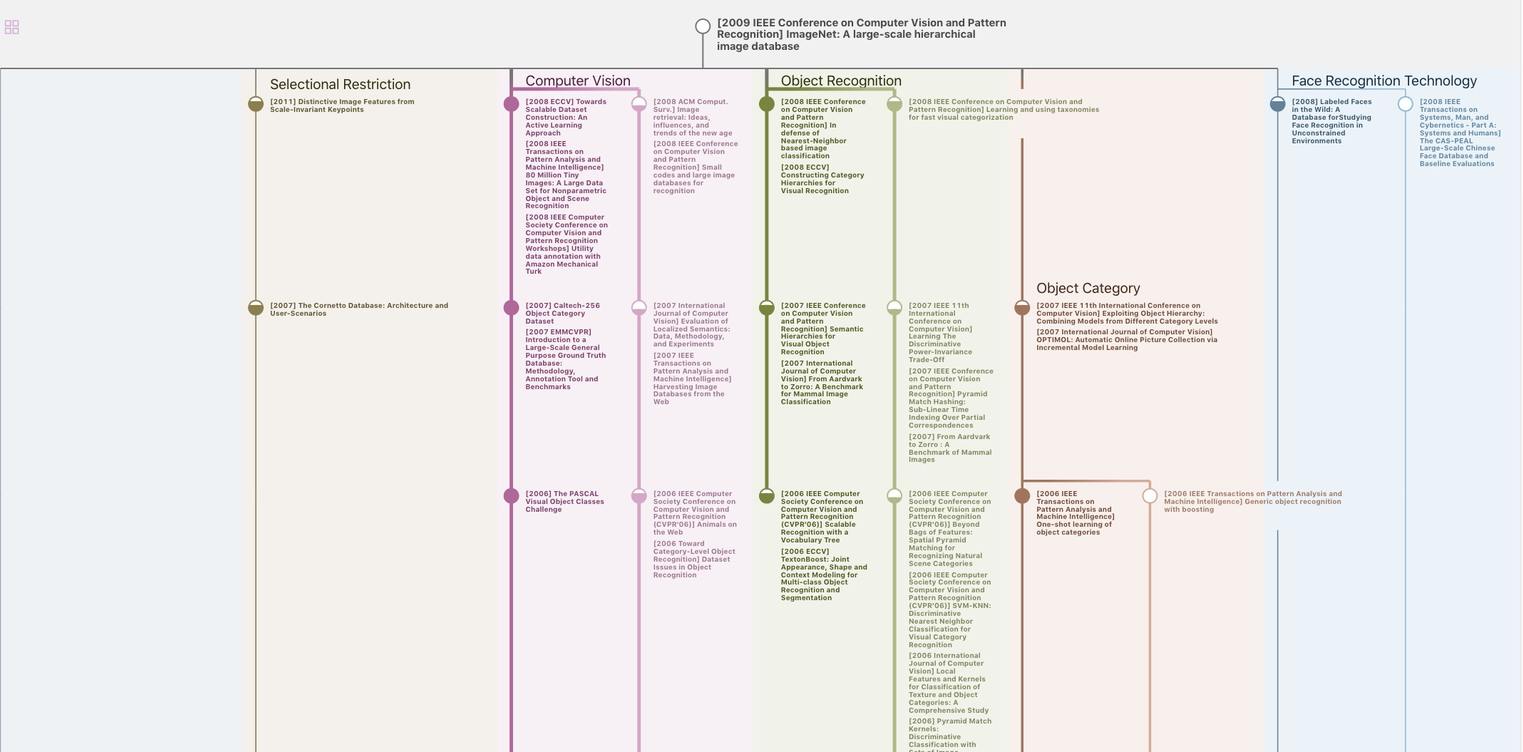
生成溯源树,研究论文发展脉络
Chat Paper
正在生成论文摘要