A Two-Stage CNN-LSTM Model-Based Transient Stability Assessment for Power System
2024 4th International Conference on Smart Grid and Renewable Energy (SGRE)(2024)
摘要
Transient Stability Assessment (TSA) has gained increased attention as a preventative measure against grid black-outs. This paper proposes an efficient two-stage Deep Learning (DL)-based TSA strategy to provide critical insights to operators for timely emergency control action. The proposed DL-based TSA scheme uses a Convolutional Neural Network-Long Short-Term Memory (CNN-LSTM) model in the first stage. A K-Nearest Neighbors (KNN) model is employed in the second stage. The CNN model recognizes the spatial patterns, while the LSTM model efficiently tracks temporal dependencies. To further enhance the efficacy of the approach and reduce the computational burden associated with time-domain simulation-based TSA without sacrificing performance, the Minimum Redundancy Maximum Relevance (MRMR) algorithm is adopted for feature ranking and dimensionality reduction. Furthermore, Bayesian optimization is implemented to fine-tune the hyperparameters of the CNN-LSTM within a predefined search space. The in-depth analysis and simulations are conducted using PowerWorld simulator. The performance of the two-stage CNN-LSTM-KNN scheme is evaluated on the IEEE 39-bus system and validated to be of high accuracy.
更多查看译文
关键词
Convolutional Neural Network (CNN),deep learning,Long Short-Term Memory (LSTM),power system dynamics,transient stability assessment
AI 理解论文
溯源树
样例
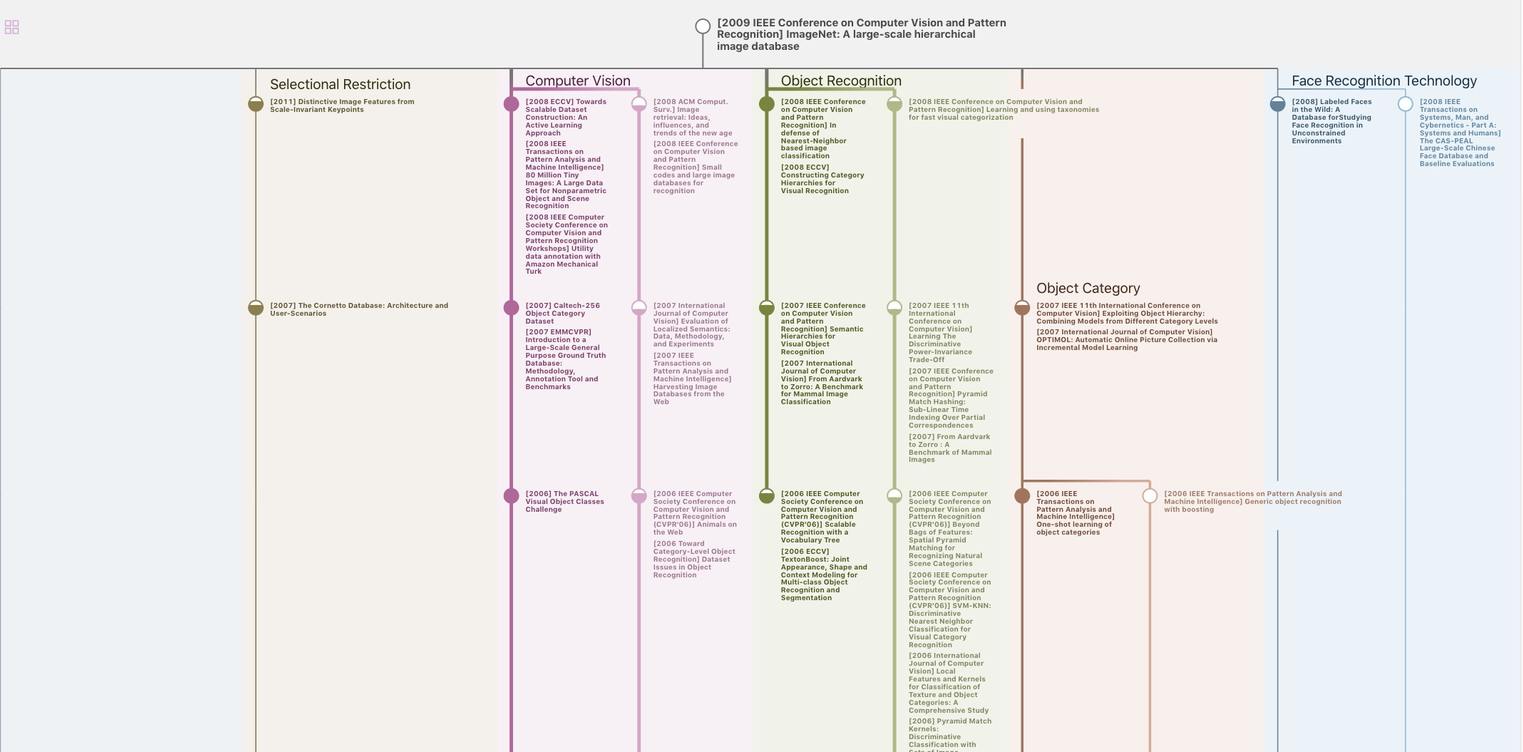
生成溯源树,研究论文发展脉络
Chat Paper
正在生成论文摘要