Temporal attention networks for biomedical hypothesis generation
JOURNAL OF BIOMEDICAL INFORMATICS(2024)
摘要
Objectives: Hypothesis Generation (HG) is a task that aims to uncover hidden associations between disjoint scientific terms, which influences innovations in prevention, treatment, and overall public health. Several recent studies strive to use Recurrent Neural Network (RNN) to learn evolutional embeddings for HG. However, the complex spatiotemporal dependencies of term -pair relations will be difficult to depict due to the inherent recurrent structure. This paper aims to accurately model the temporal evolution of term -pair relations using only attention mechanisms, for capturing crucial information on inferring the future connectivities. Methods: This paper proposes a Temporal Attention Networks (TAN) to produce powerful spatiotemporal embeddings for Biomedical Hypothesis Generation. Specifically, we formulate HG problem as a future connectivity prediction task in a temporal attributed graph. Our TAN develops a Temporal Spatial Attention Module (TSAM) to establish temporal dependencies of node -pair (term -pair) embeddings between any two time -steps for smoothing spatiotemporal node -pair embeddings. Meanwhile, a Temporal Difference Attention Module (TDAM) is proposed to sharpen temporal differences of spatiotemporal embeddings for highlighting the historical changes of node -pair relations. As such, TAN can adaptively calibrate spatiotemporal embeddings by considering both continuity and difference of node -pair embeddings. Results: Three real -world biomedical term relationship datasets are constructed from PubMed papers. TAN significantly outperforms the best baseline with 12.03%, 4.59 and 2.34% Micro -F1 Score improvement in Immunotherapy, Virology and Neurology, respectively. Extensive experiments demonstrate that TAN can model complex spatiotemporal dependencies of term -pairs for explicitly capturing the temporal evolution of relation, significantly outperforming existing state-of-the-art methods. Conclusion: We proposed a novel TAN to learn spatiotemporal embeddings based on pure attention mechanisms for HG. TAN learns the evolution of relationships by modeling both the continuity and difference of temporal term -pair embeddings. The important spatiotemporal dependencies of term -pair relations are extracted based solely on attention mechanism for generating hypotheses.
更多查看译文
关键词
Hypothesis generation,Temporal Attention Networks,Temporal dependency,Temporal difference
AI 理解论文
溯源树
样例
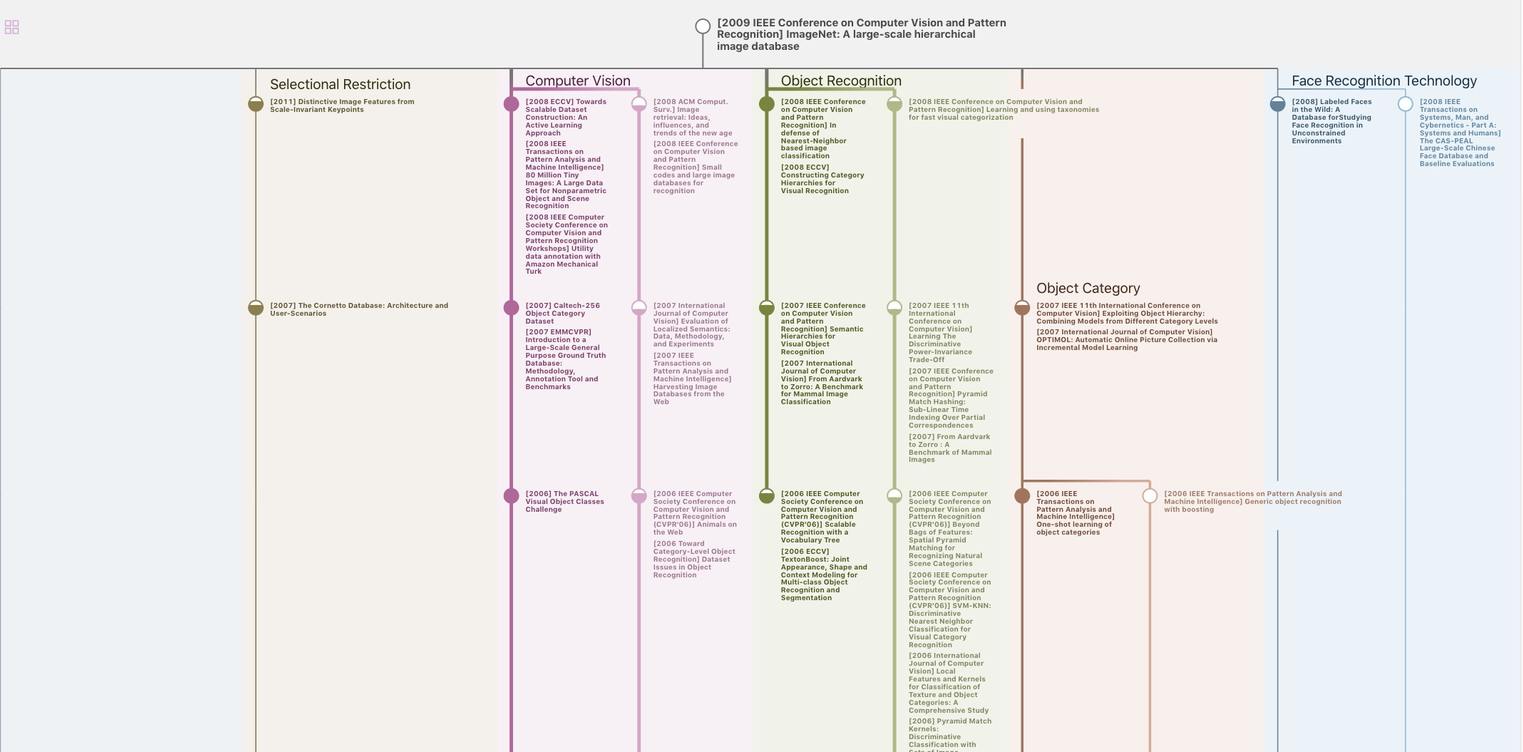
生成溯源树,研究论文发展脉络
Chat Paper
正在生成论文摘要