Silver Nanowire Networks with Moisture-Enhanced Learning Ability
ACS APPLIED MATERIALS & INTERFACES(2024)
摘要
The human brain possesses a remarkable ability to memorize information with the assistance of a specific external environment. Therefore, mimicking the human brain's environment-enhanced learning abilities in artificial electronic devices is essential but remains a considerable challenge. Here, a network of Ag nanowires with a moisture-enhanced learning ability, which can mimic long-term potentiation (LTP) synaptic plasticity at an ultralow operating voltage as low as 0.01 V, is presented. To realize a moisture-enhanced learning ability and to adjust the aggregations of Ag ions, we introduced a thin polyvinylpyrrolidone (PVP) coating layer with moisture-sensitive properties to the surfaces of the Ag nanowires of Ag ions. That Ag nanowire network was shown to exhibit, in response to the humidity of its operating environment, different learning speeds during the LTP process. In high-humidity environments, the synaptic plasticity was significantly strengthened with a higher learning speed compared with that in relatively low-humidity environments. Based on experimental and simulation results, we attribute this enhancement to the higher electric mobility of the Ag ions in the water-absorbed PVP layer. Finally, we demonstrated by simulation that the moisture-enhanced synaptic plasticity enabled the device to adjust connection weights and delivery modes based on various input patterns. The recognition rate of a handwritten data set reached 94.5% with fewer epochs in a high-humidity environment. This work shows the feasibility of building our electronic device to achieve artificial adaptive learning abilities.
更多查看译文
关键词
moisture-enhanced learning ability,Ag nanowire network,long-term potentiation,Ag nanofilament,electricmobility
AI 理解论文
溯源树
样例
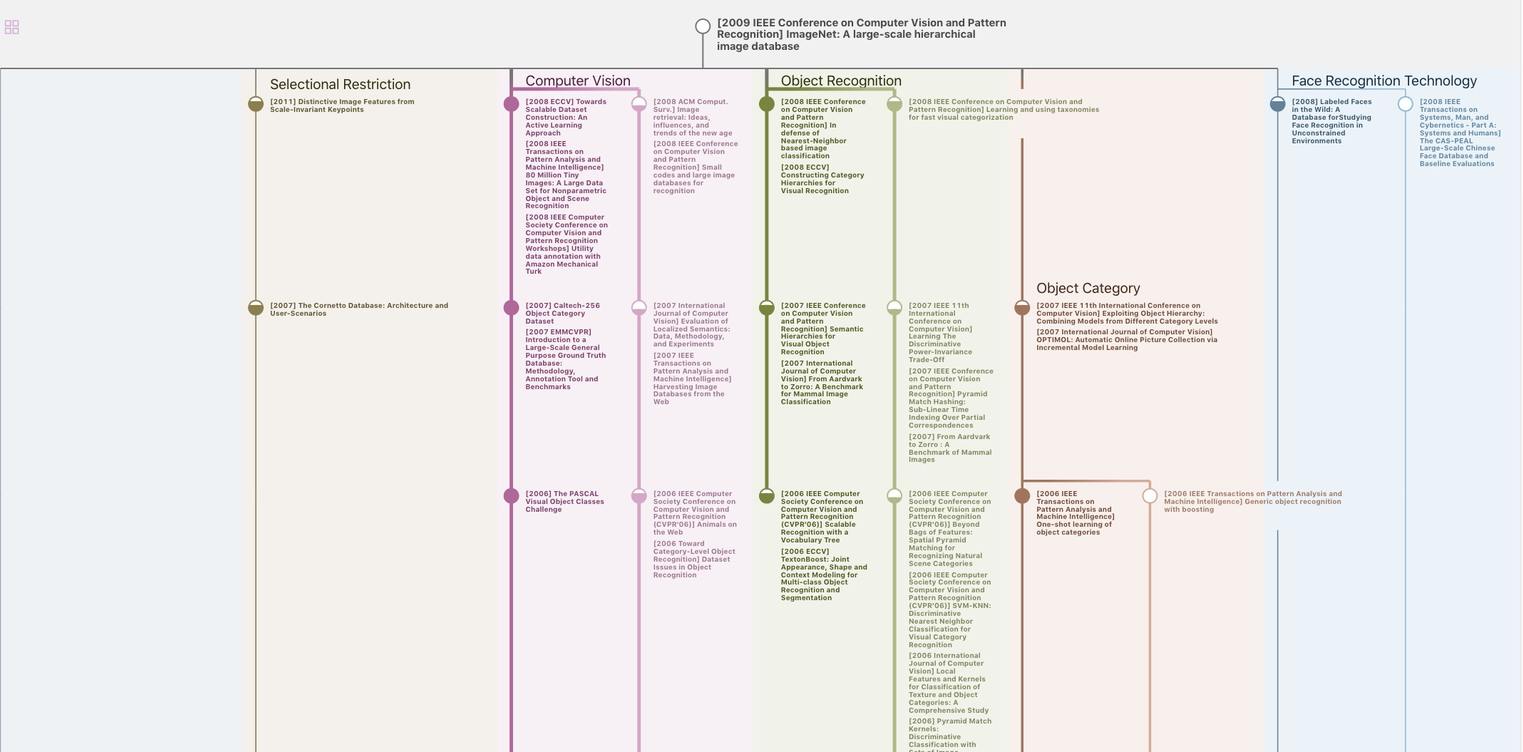
生成溯源树,研究论文发展脉络
Chat Paper
正在生成论文摘要