A Machine Learning Framework for Bearing Fault Detection in Three-Phase Induction Motors
2024 4th International Conference on Smart Grid and Renewable Energy (SGRE)(2024)
摘要
Three-phase induction motors are widely employed in industry due to their rugged performance and easy maintenance. Bearing faults in three phase induction motors are responsible for 40%-50% of unplanned shutdowns in industrial settings. Therefore, early detection of bearing faults is essential to implement preventive measures and enhance planning of maintenance strategies. This paper thus proposes a machine learning (ML) framework that consistently monitors acceleration and temperature of bearing to detect bearing faults. The results show that the ML framework using k-nearest neighbor (k-NN) and support vector machine (SVM) approaches is better than the variation-based thresholding approach, where the former method is able to detect faulty conditions with more than 99% accuracy.
更多查看译文
关键词
Bearing fault,electric motors,machine learning,fault detection,condition monitoring
AI 理解论文
溯源树
样例
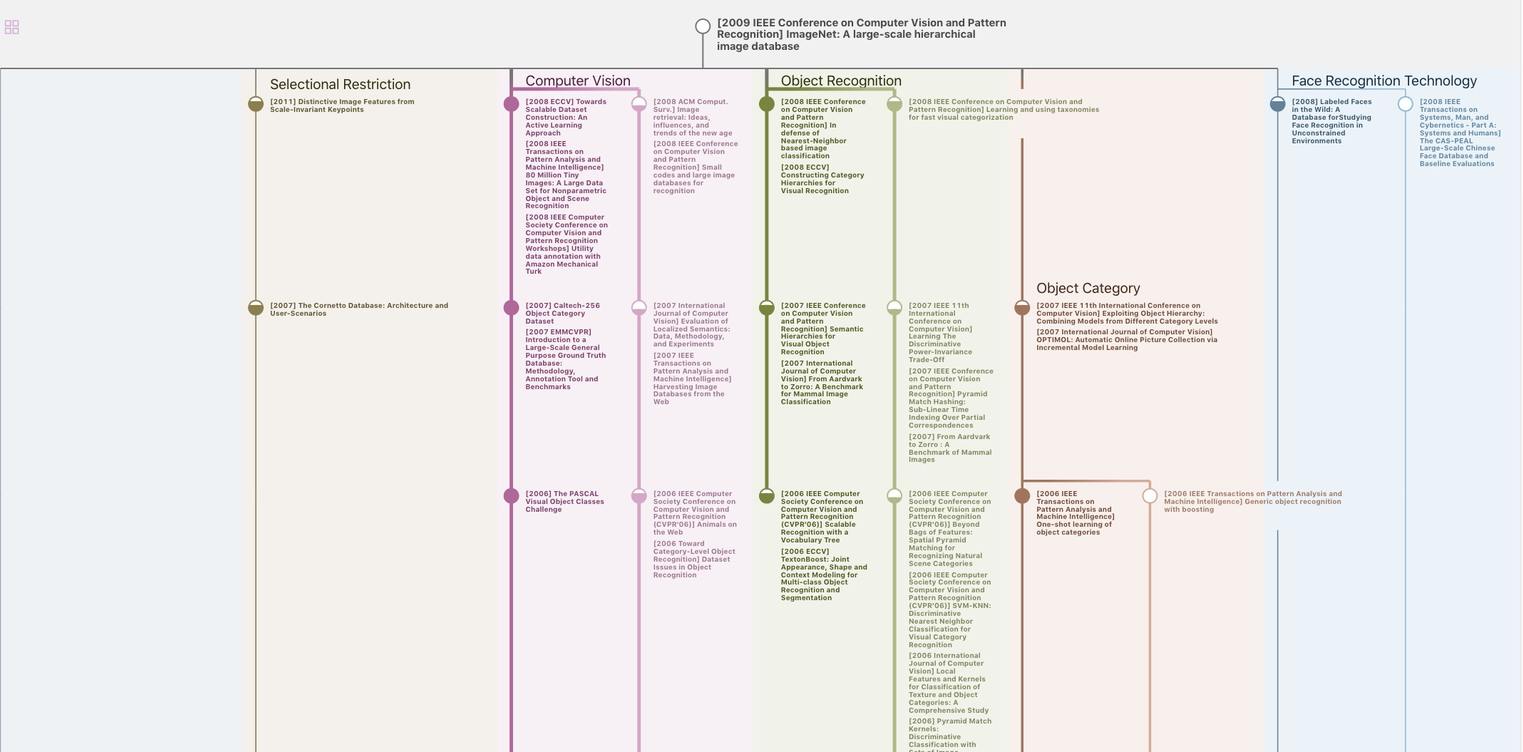
生成溯源树,研究论文发展脉络
Chat Paper
正在生成论文摘要