Unraveling the Causal Graph: Investigating Disease Etiology through Causal Structure Learning
2023 9th International Conference on Big Data and Information Analytics (BigDIA)(2023)
摘要
This paper aims to develop a robust methodology for unraveling the complex causal relationships inherent in disease dynamics, using observational data as a basis. Our primary objective is to elucidate the intricate network of causal factors influencing disease cases, with a specific focus on breast quadrant disease. To achieve this, we employ Directed Acyclic Graphs (DAGs) as our primary analytical tool. Our approach begins by constructing a fully connected graph to hypothesize potential causal links among various factors. This graph undergoes a meticulous refinement process through Conditional Independence (CI) tests, which systematically eliminates non-significant connections. The outcome is a streamlined DAG that clearly maps out both direct and indirect causal relationships relevant to disease progression. The validity and effectiveness of our model are rigorously tested using a score-based Hill-Climbing (HC) algorithm, which ensures that our causal inferences align accurately with real-world data. The culmination of this process is demonstrated through the application of our methodology to a dataset focusing on breast quadrant disease. Our findings reveal significant causal connections within this dataset, which have been corroborated by expert opinions in the field. These results not only validate our approach but also emphasize its practical utility in deciphering complex causal relationships in medical research.
更多查看译文
关键词
Disease analysis,Causal discovery,Causal graph
AI 理解论文
溯源树
样例
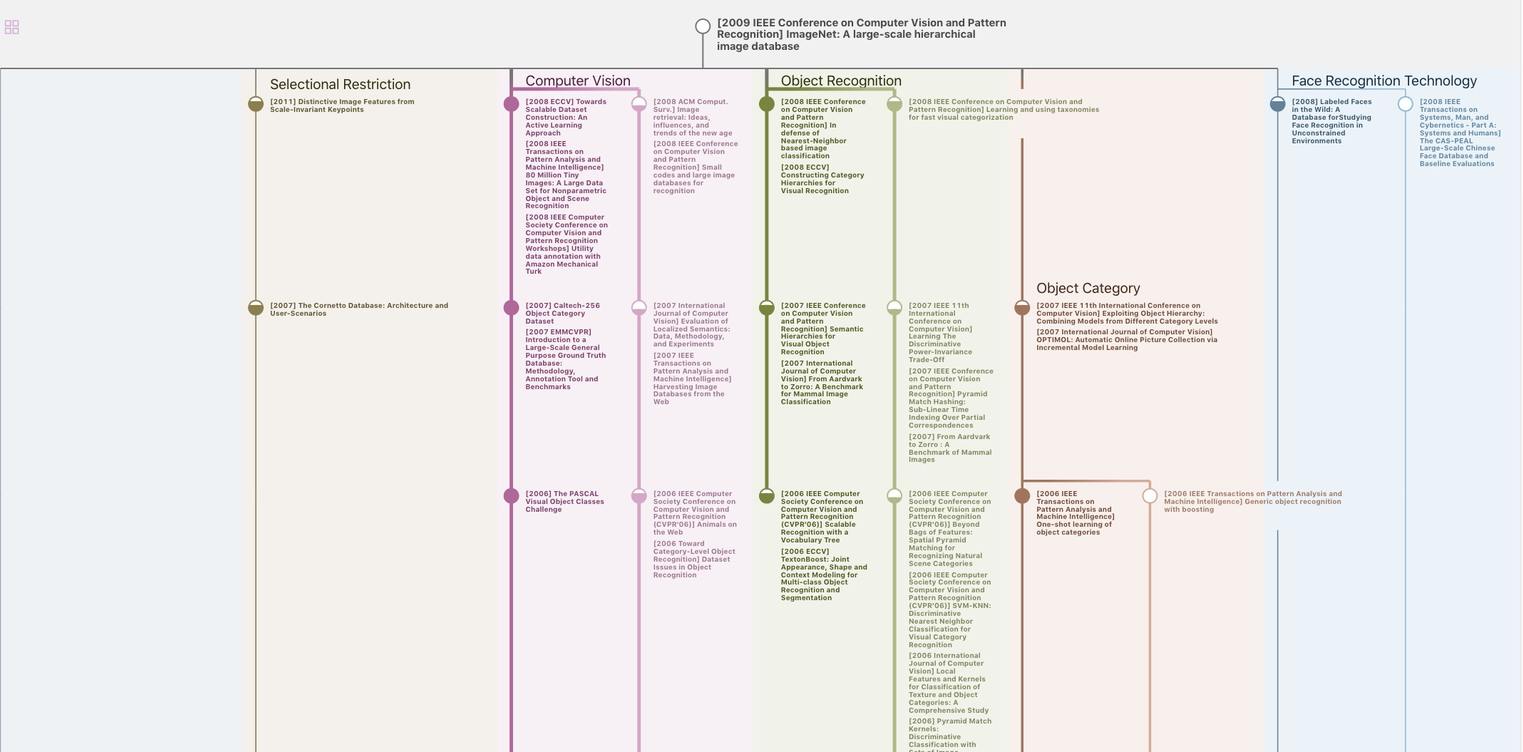
生成溯源树,研究论文发展脉络
Chat Paper
正在生成论文摘要