Cost-Aware Hierarchical Federated Learning for Smart Healthcare.
International Conference on Communication Systems and Networks(2024)
摘要
Federated Learning (FL) enables local model training on devices while collaboratively updating a global model on a server, ensuring user data privacy by keeping it on the device and sharing only model updates. Although it has been widely employed in healthcare to address privacy concerns, still deployment faces challenges due to variations in device data and system capacities. Moreover, the substantial distance between a global cloud server and users leads to significant energy consumption during the exchange of local model weights and parameter aggregation. Therefore, this paper proposes a method for minimizing energy and time consumed during transmission and computation of health data generated by Wireless Body Area Networks (WBANs) along with loss function using hierarchical FL. Through extensive evaluation, the proposed approach showcases an 11.13% reduction in total costs, encompassing latency, energy, and loss function, compared to the traditional Federated Averaging (FedAvg) algorithm. Moreover, the proposed model attains an accuracy of 95.85%, surpassing the 94.77% and 94.53% achieved by FedAvg and Federated Clustering (FedCluster), respectively.
更多查看译文
关键词
Federated learning,Healthcare,WBAN,Energy,Loss function
AI 理解论文
溯源树
样例
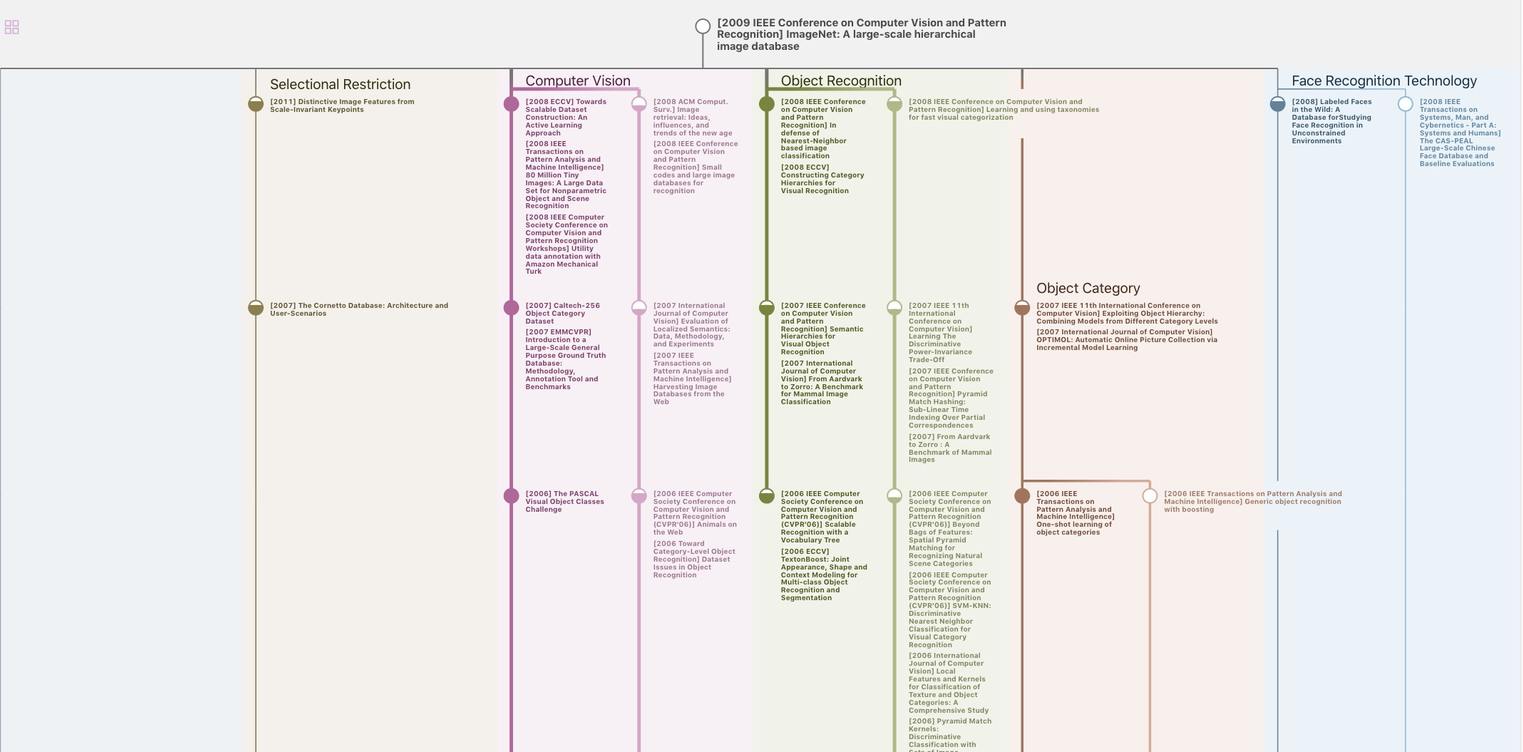
生成溯源树,研究论文发展脉络
Chat Paper
正在生成论文摘要