Next-Generation Autonomous Vehicle Navigation: A DDPG Reinforcement Learning Approach to Dynamic HD Map Caching.
International Conference on Communication Systems and Networks(2024)
摘要
This work presents a solution to the challenges of real-time access and processing of High-Definition maps (HD-maps) in vehicular networks with limited bandwidth and high latency. Leveraging the Deep Deterministic Policy Gradient (DDPG) algorithm, the proposed approach dynamically caches relevant map tiles in Roadside Units (RSUs). By intelligently determining which map tiles to cache, considering factors like popularity, recency, and anticipated demand, RSUs can serve a significantly portion of map requests from vehicles. Empirical results demonstrate the superiority of proposed DDPG-based HD map caching over traditional methods leading to improved map retrieval times and alleviating network congestion.
更多查看译文
关键词
Autonomous Vehicles,Network Congestion,Vehicular Networks,Roadside Units,Learning Algorithms,Deep Neural Network,Time Series Data,State Space,Mapping Data,Actor Network,Data Retrieval,Time Stamp,Target Network,Intelligent Transportation Systems,Critic Network,Continuous Action Space,Content Caching,Cache Size,Frequent Requests,Cache Hit,Caching Scheme,Edge Caching,Cache Misses,High-dimensional State Space,Map Information,Item Content,State Representation,Neural Network,Time Series
AI 理解论文
溯源树
样例
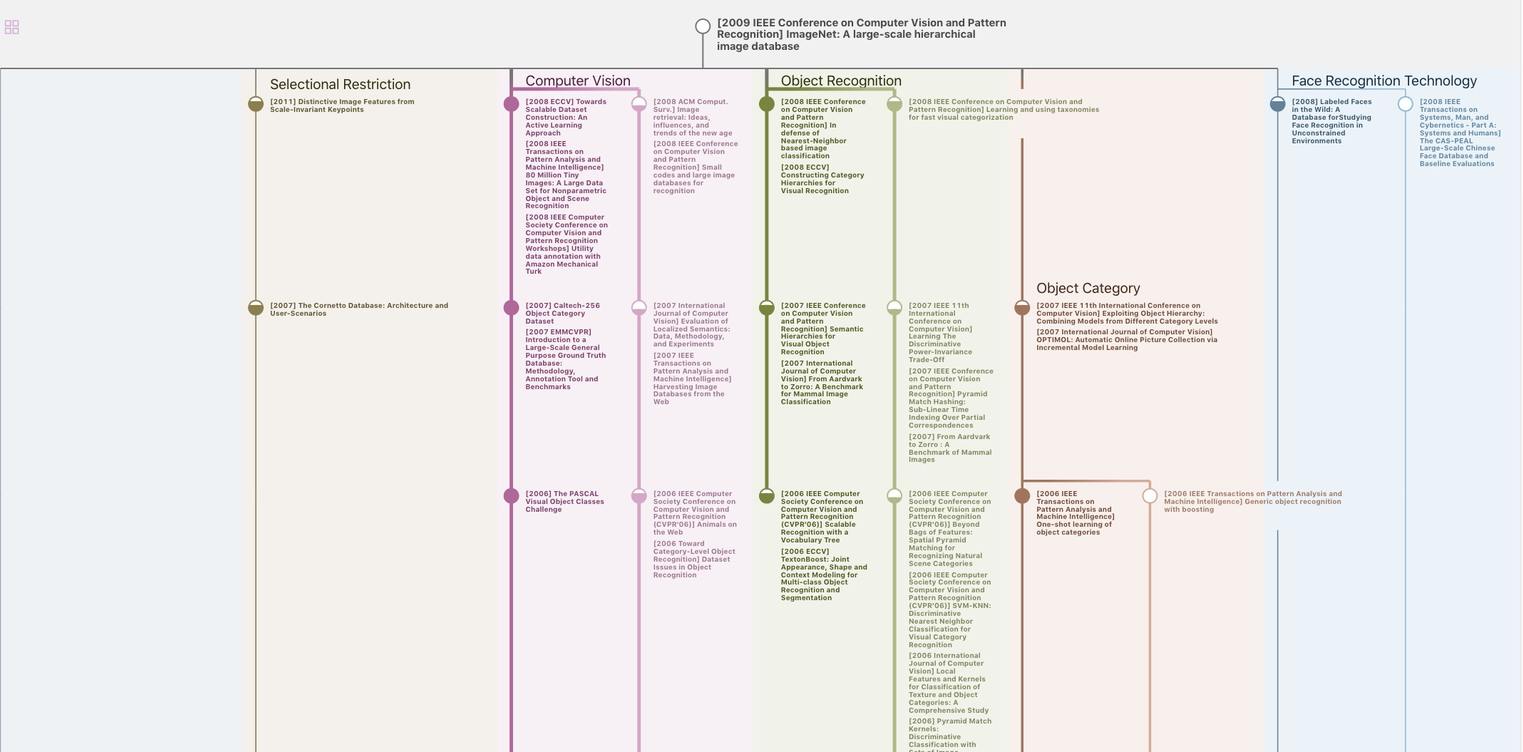
生成溯源树,研究论文发展脉络
Chat Paper
正在生成论文摘要