Annotate Less but Perform Better: Weakly Supervised Shadow Detection Via Label Augmentation
The visual computer/The visual computer(2024)
摘要
Shadow detection is essential for scene understanding and image restoration. Existing paradigms for producing shadow detection training data usually rely on densely labeling each image pixel, which will lead to a bottleneck when scaling up the number of images. To tackle this problem, by labeling shadow images with only a few strokes, this paper designs a learning framework for Weakly supervised Shadow Detection, namely WSD. Firstly, it creates two shadow detection datasets with scribble annotations, namely Scr-SBU and Scr-ISTD. Secondly, it proposes an uncertainty-guided label augmentation scheme based on graph convolutional networks, which can propagate the sparse scribble annotations to more reliable regions, and then avoid the model converging to an undesired local minima as intra-class discontinuity. Finally, it introduces a multi-task learning framework to jointly learn for shadow detection and edge detection, which encourages generated shadow maps to be comprehensive and well aligned with shadow boundaries. Experimental results on benchmark datasets demonstrate that our framework even outperforms existing semi-supervised and fully supervised shadow detectors requiring only 2% pixels to be labeled.
更多查看译文
关键词
Image segmentation,Shadow detection,Weakly supervised,Graph convolutional network,Uncertainty analysis
AI 理解论文
溯源树
样例
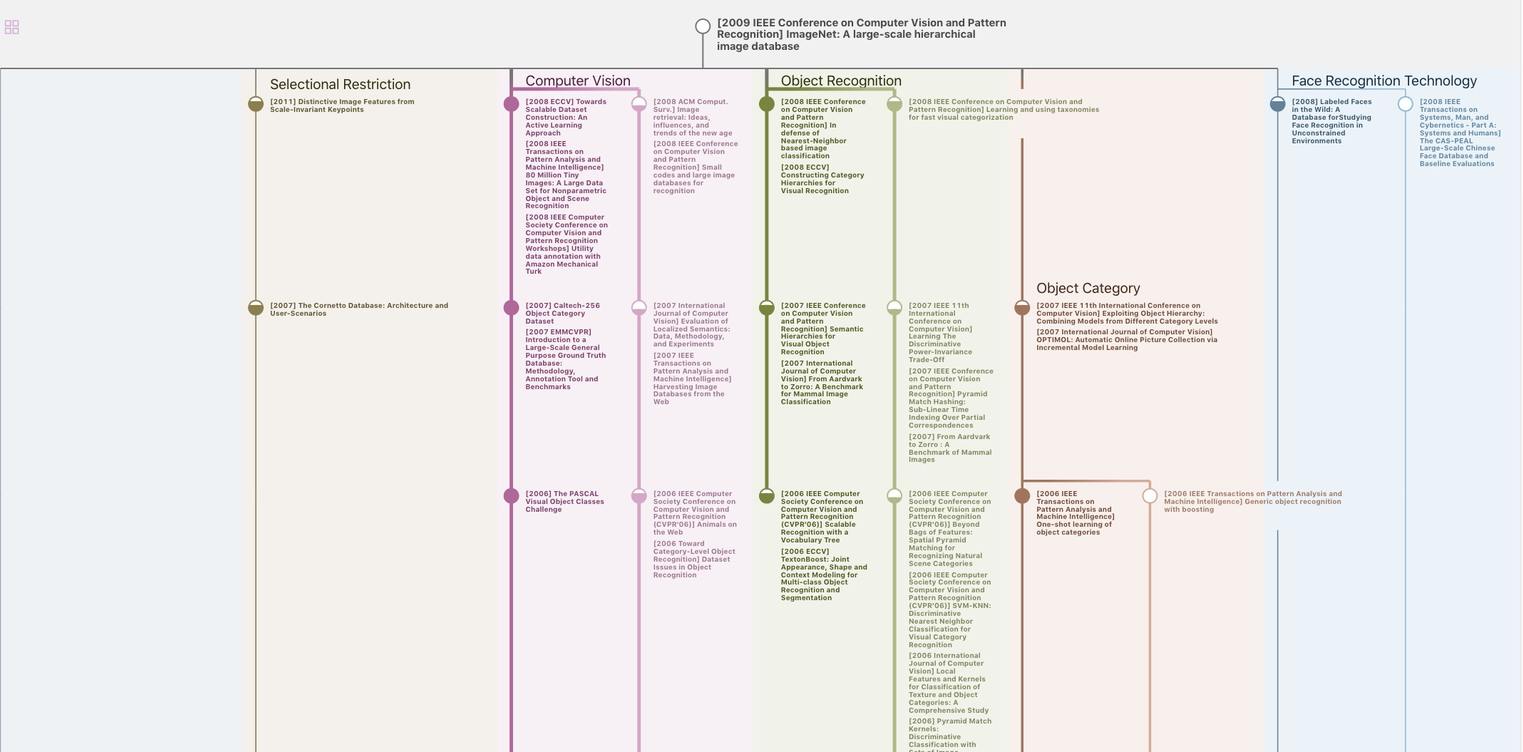
生成溯源树,研究论文发展脉络
Chat Paper
正在生成论文摘要