Guidelines for appropriate use of BirdNET scores and other detector outputs
Journal of Ornithology(2024)
摘要
Machine learning tools capable of identifying animals by sound have proliferated, making the challenge of interpreting their outputs much more prevalent. These tools, like their predecessors, quantify prediction uncertainty with scores that tend to resemble probabilities but are actually unitless scores that are (generally) positively related to prediction accuracy in species-specific ways. BirdNET is one such tool, a freely available animal sound identification algorithm capable of identifying > 6,000 species, most of them birds. We describe two ways in which BirdNET “confidence scores”—and the output scores of other detector tools—can be used appropriately to interpret BirdNET results (reviewing them down to a user-defined threshold or converting them to probabilities), and provide a step-by-step tutorial to follow these suggestions. These suggestions are complementary to common performance metrics like precision, recall, and receiver operating characteristic. BirdNET can be a powerful tool for acoustic-based biodiversity research, but its utility depends on the careful use and interpretation of its outputs.
更多查看译文
关键词
Machine learning,Passive acoustic monitoring,Bioacoustics,Detector
AI 理解论文
溯源树
样例
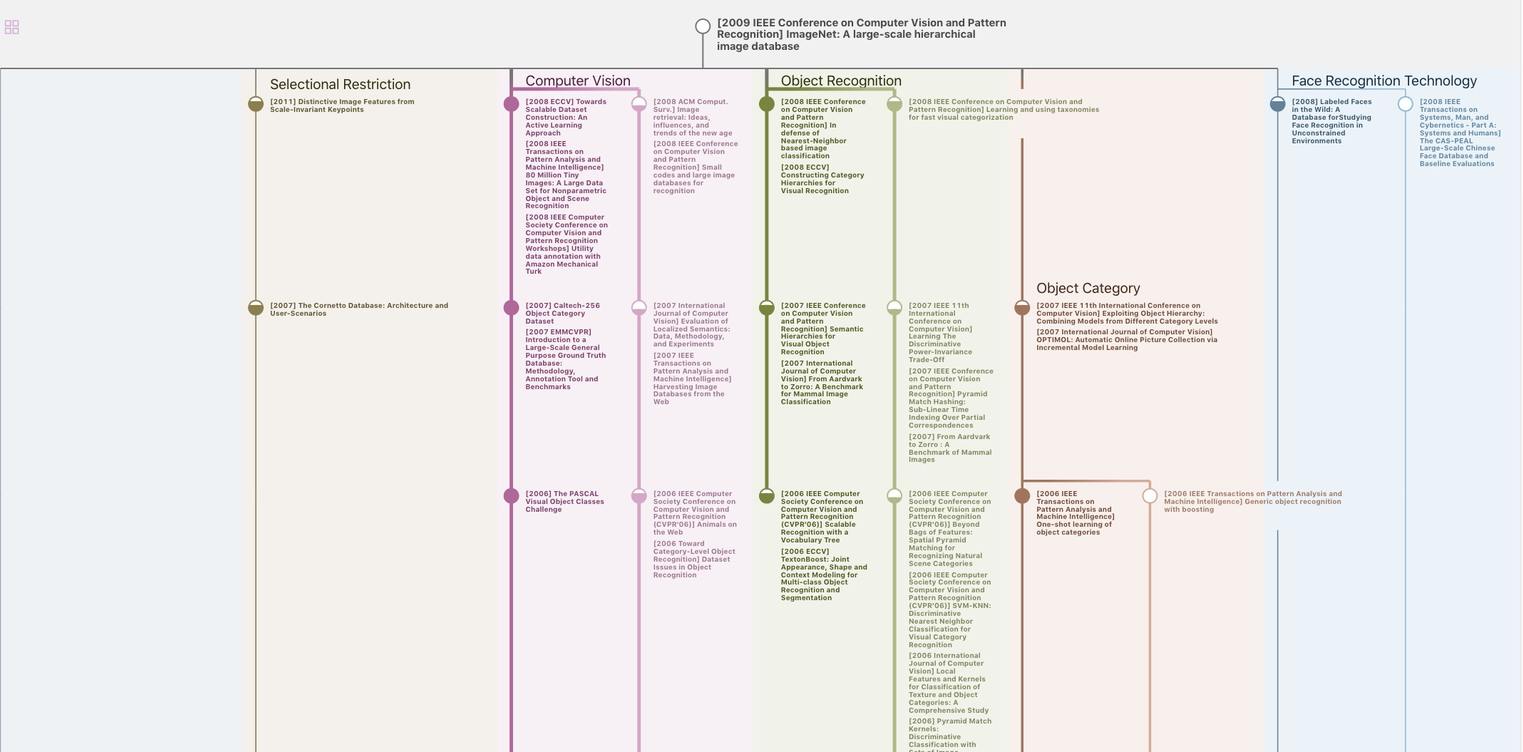
生成溯源树,研究论文发展脉络
Chat Paper
正在生成论文摘要