CLCC-FS(OBWOA): an efficient hybrid evolutionary algorithm for motor imagery electroencephalograph classification
Multimedia Tools and Applications(2024)
摘要
A brain-computer interface (BCI) based on an electroencephalograph (EEG) establishes a new channel of communication between the human brain and a computer. Redundant, noisy, and irrelevant channels lead to high computational costs and poor classification accuracy. Therefore, an effective feature selection technique for determining the optimal number of channels can improve BCI’s performance. However, existing meta-heuristic algorithms are prone to get trapped in local optimum due to high dimensional dataset. Thus, to reduce dimension, solve inter subject variation and choose an optimal subset of channels, a novel framework called Component Loading followed by Clustering and Classification (CLCC) is proposed in this paper. This novel framework is further divided into two experiment configurations-CLCC with Feature Selection (CLCC-FS) and CLCC without Feature Selection (CLCC-WFS). All these frameworks have been implemented on a motor imagery (MI) EEG dataset of 10 subjects in order to choose the best subset of channels. Further, seven different classifiers have been employed to assess the performance. Experimental outcomes show that on comparing various feature selection techniques, our proposed algorithm i.e., CLCC-FS Opposition-Based Whale Optimization Algorithm (CLCC-FS(OBWOA)) performed substantially better than the other feature selection techniques. We demonstrate that the proposed algorithm is able to achieve 99.6% accuracy by using only few channels and can improve the practicality of the BCI system by reducing the computation cost.
更多查看译文
关键词
Brain computer interface,Feature selection,Electroencephalography,Computer vision,Hybrid intelligent systems,Machine learning,Artificial intelligence
AI 理解论文
溯源树
样例
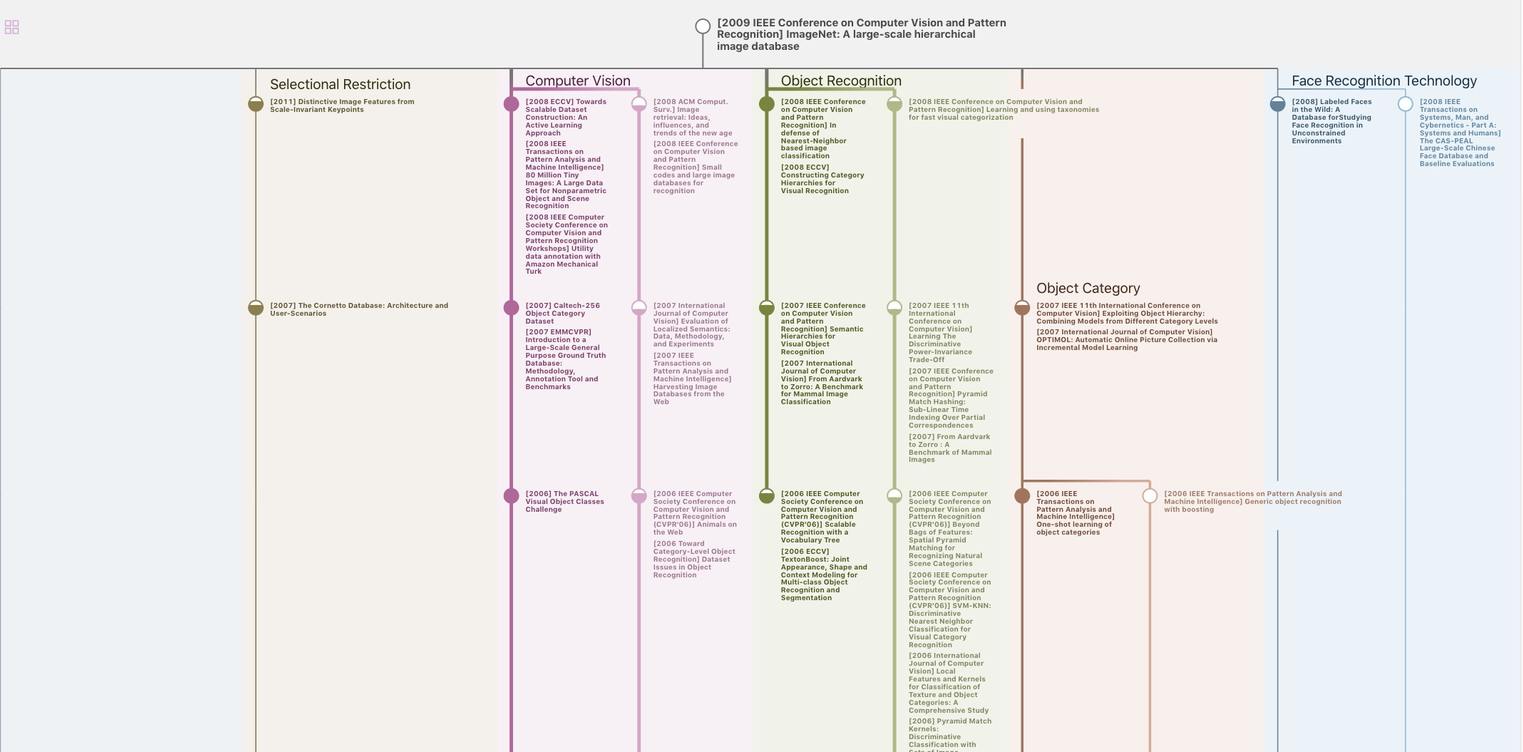
生成溯源树,研究论文发展脉络
Chat Paper
正在生成论文摘要