SVG-CNN: A shallow CNN based on VGGNet applied to intra prediction partition block in HEVC
Multimedia Tools and Applications(2024)
摘要
High Efficiency Video Coding (HEVC) offers superior compression rates, but its adoption introduces increased coding complexity due to its reliance on a recursive quad-tree for partitioning frames into varying block sizes. This quad-tree process is a central feature in upcoming video coding standards. Our paper presents a novel framework, SVG-CNN, which integrates three shallow Convolutional Neural Networks (CNNs) inspired by VGGNet. Each CNN is specifically designed for individual quad-tree levels to predict the Code Unit (CU) partition in HEVC, leading to reduced intra-frame coding time. SVG-CNN has an inherent capability for early terminations, leveraging sequential CNN feeding based on quad-tree level probabilities. This provides a mechanism to halt processes when further refinement is seemed unlikely. Enhancing the model's efficacy, we have crafted three specialized datasets, each focusing on distinct quad-tree levels and quantization parameter (QP) contexts. This allows each CNN within our framework to undergo targeted training, establishing a cutting-edge training methodology. Our study shows that performance, in terms of accuracy and F1 metrics, is highly dependent on QP settings, with lower QPs yielding better results, and higher QPs diminishing performance due to potential loss of critical features. To enhance our model, we tackled hyperparameter selection and CU split threshold determination for HEVC prediction. We utilized Grid Search Cross-Validation for the former and assessed multiple thresholds across selected videos for the latter. The model has a moderate complexity with over 328,000 parameters across 18 layers, which ensures memory efficiency. It boasts a swift prediction time of 0.05 ms and reduces HEVC encoding time by 61.64%, while slightly improving the bitrate-distortion performance by -0.24% BDBR, indicating better compression without notable PSNR loss. Significantly, our approach outperforms other CNN-based quad-tree partitioning methods that reduce HEVC coding complexity but sacrifice compression performance.
更多查看译文
关键词
HEVC,Complexity reduction,Block partitioning,Intra coding,Shallow CNN
AI 理解论文
溯源树
样例
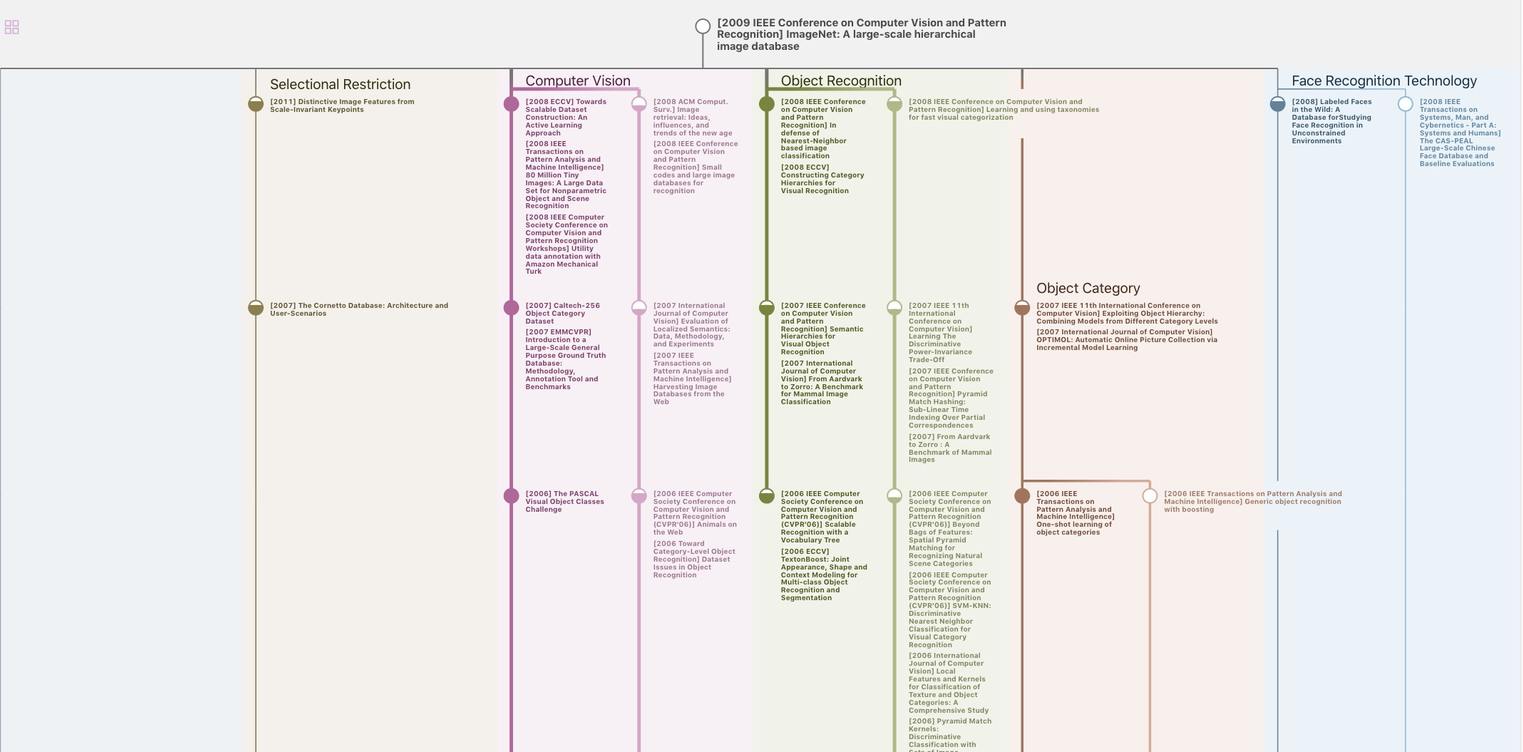
生成溯源树,研究论文发展脉络
Chat Paper
正在生成论文摘要