Two-step semiparametric empirical likelihood inference from capture–recapture data with missing covariates
TEST(2024)
摘要
Missing covariates are not uncommon in capture–recapture studies. When covariate information is missing at random in capture–recapture data, an empirical full likelihood method has been demonstrated to outperform conditional-likelihood-based methods in abundance estimation. However, the fully observed covariates must be discrete, and the method is not directly applicable to continuous-time capture–recapture data. Based on the Binomial and Poisson regression models, we propose a two-step semiparametric empirical likelihood approach for abundance estimation in the presence of missing covariates, regardless of whether the fully observed covariates are discrete or continuous. We show that the maximum semiparametric empirical likelihood estimators for the underlying parameters and the abundance are asymptotically normal, and more efficient than the counterpart for a completely known non-missingness probability. After scaling, the empirical likelihood ratio test statistic for abundance follows a limiting chi-square distribution with one degree of freedom. The proposed approach is further extended to one-inflated count regression models, and a score-like test is constructed to assess whether one-inflation exists among the number of captures. Our simulation shows that, compared with the previous method, the proposed method not only performs better in correcting bias, but also has a more accurate coverage in the presence of fully observed continuous covariates, although there may be a slight efficiency loss when the fully observed covariates are only discrete. The performance of the new method is illustrated by analyses of the yellow-bellied prinia data and the rana pretiosa data.
更多查看译文
关键词
Capture–recapture data,Population size,Semiparametric empirical likelihood,Missing at random
AI 理解论文
溯源树
样例
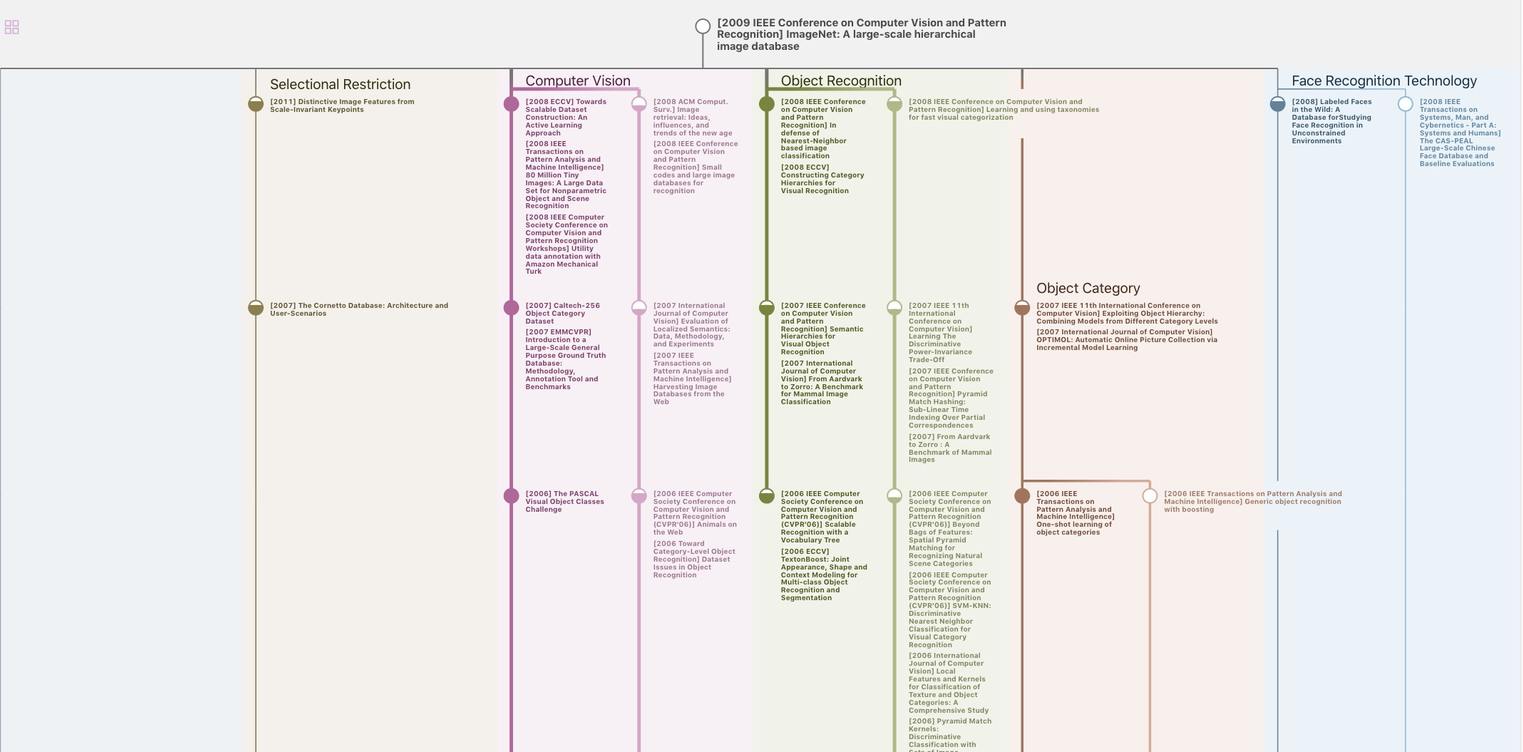
生成溯源树,研究论文发展脉络
Chat Paper
正在生成论文摘要