Let's Learn Step by Step: Enhancing In-Context Learning Ability with Curriculum Learning
CoRR(2024)
关键词
Instructional Design,Teaching Strategies,Higher-Order Thinking,Virtual Learning Environments,Instructional Interventions
AI 理解论文
溯源树
样例
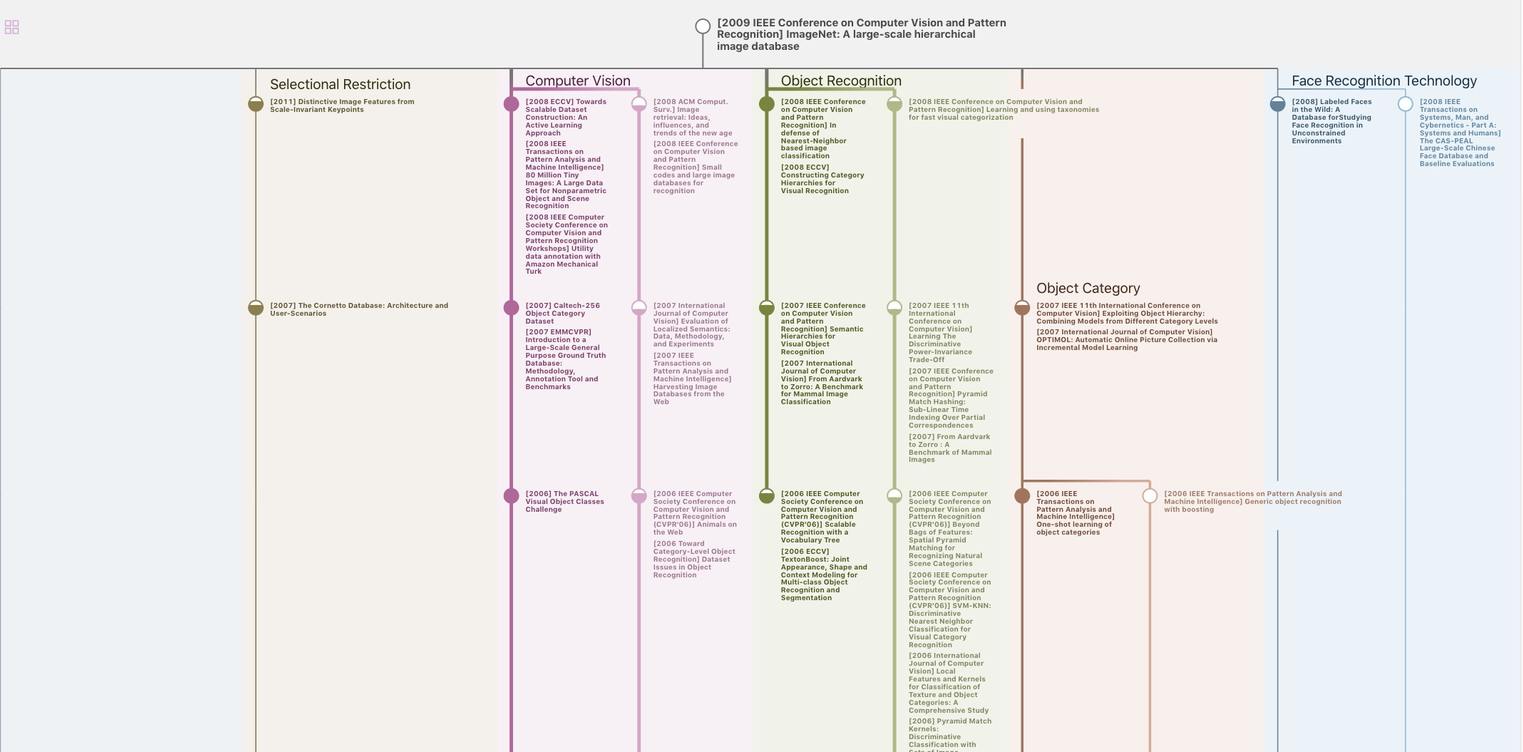
生成溯源树,研究论文发展脉络
Chat Paper
正在生成论文摘要