A quantitative relation for the ductile-brittle transition temperature in pipeline steel
SCRIPTA MATERIALIA(2024)
摘要
An accurate description of pipeline steel at low temperatures requires a comprehensive understanding of its ductile-brittle transition temperature (DBTT). In this work, we collect a data set of low-temperature toughness for pipeline steel and reduce the dimensionality of the data set using several feature screening approaches. Multiple machine learning models, validated via ten-fold cross-validation, are then employed to fit and predict the DBTT. Symbolic regression allows us to derive a relation for DBTT appropriate for pipeline steel. Such a formula is shown to provide a versatile model to estimate the DBTT of pipeline steel. It not only serves as a guide to predict the low-temperature properties of pipeline steel but also lays the groundwork for further research on other steel materials.
更多查看译文
关键词
Pipeline steel,Ductile-brittle transition temperature,Machine learning,Symbolic regression
AI 理解论文
溯源树
样例
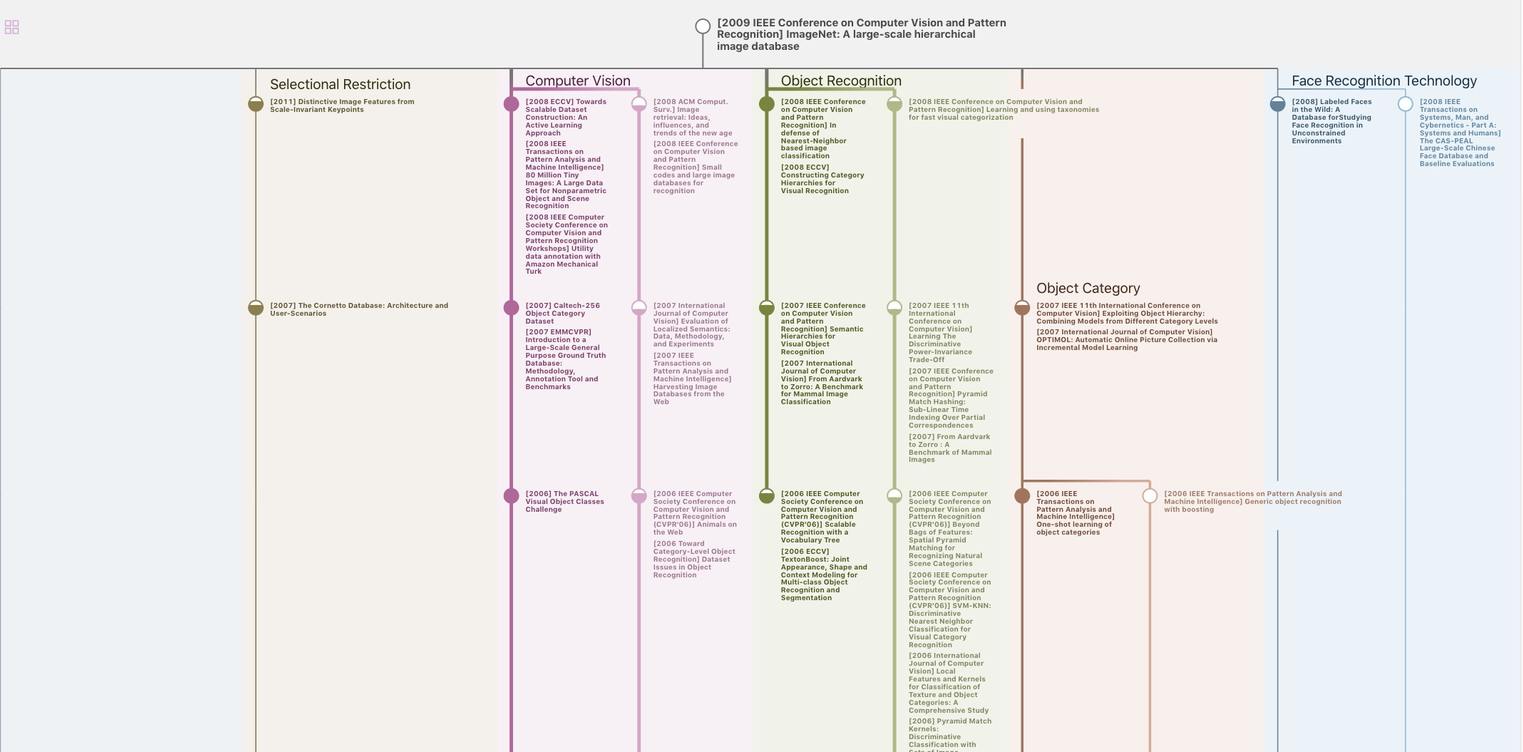
生成溯源树,研究论文发展脉络
Chat Paper
正在生成论文摘要