Enabling discovery of materials through enhanced generalisability of deep learning models
arxiv(2024)
摘要
The road towards the discovery of new, useful materials is full of
imperfections. Machine learning, which is currently the power horse of material
discovery when it works in concert with density functional theory, has been
able to accelerate the discovery of new materials for various applications by
learning the properties of known, stable materials to infer the properties of
unknown, deformed materials. Physics-informed machine learning (PIML) is
particularly believed to bridge the gap between known materials and the
virtually infinite space of crystal structures with imperfections such as
defects, grain boundaries, composition disorders and others. State of the art
PIML models struggle to bridge this gap, however. In this work we show that a
critical correction of the physics underpinning a PIML, our direct integration
of external potential (DIEP) method, which mimics the integration of the
external potential term in typical density functional theory calculations,
improves the generalisability of the model to a range of imperfect structures,
including diamond defects. DIEP has the potential of enhancing the accuracy of
potential energy surface prediction, which can accelarate structure discovery.
更多查看译文
AI 理解论文
溯源树
样例
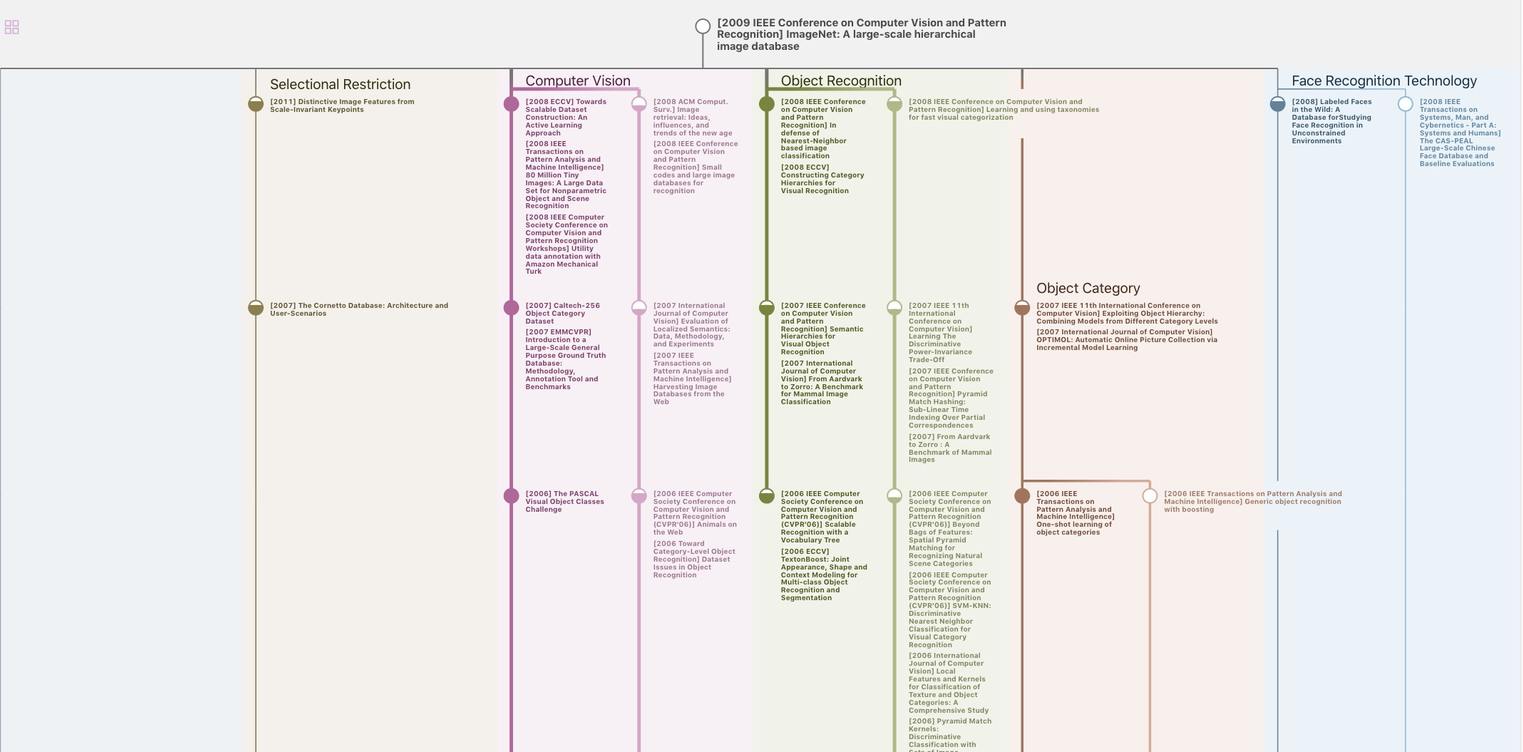
生成溯源树,研究论文发展脉络
Chat Paper
正在生成论文摘要