A Low-Dissipation and Scalable GEMM Accelerator with Silicon Nitride Photonics
CoRR(2024)
摘要
Over the past few years, several microring resonator (MRR)-based analog
photonic architectures have been proposed to accelerate general matrix-matrix
multiplications (GEMMs), which are found in abundance in deep learning
workloads.These architectures have dramatically grown in popularity because
they offer exceptional throughput and energy efficiency compared to their
electronic counterparts. However, such architectures, due to their traditional
realization based on the silicon-on-insulator (SOI) material platform, face two
shortcomings. First, the high-index contrast of the SOI platform incurs high
scattering losses, which mandates the provisioning of high optical input
power.Second, SOI waveguides are susceptible to two-photon absorption, which
can incur substantial optical signal losses at moderate-to-high signal fan-in.
These shortcomings have severely detrimental effects on the achievable
parallelism, throughput, and energy efficiency of SOI MRR-based GEMM
accelerators. To address these shortcomings, we present a novel Silicon Nitride
(SiN)-Based Photonic GEMM Accelerator called SiNPhAR. SiNPhAR architecture
employs SiN-based active and passive devices to implement analog GEMM
functions. Since the SiN material exhibits lower index contrast and no TPA, the
optical signal losses in our SiNPhAR architecture are very low. This advantage
significantly enhances the achievable processing parallelism, throughput, and
energy efficiency of SiNPhAR architecture, compared to SOI-based photonic GEMM
accelerators from prior work. We quantify and compare these benefits of SiNPhAR
architecture via our cross-layer evaluation for a benchmark workload comprising
four modern deep neural network models. From the system-level performance
analysis, SiNPhAR demonstrates at least 1.7x better throughput FPS while
consuming at least 2.8x better energy efficiency (FPS/W) than prior SOI-based
GEMM accelerators.
更多查看译文
关键词
Silicon Nitride,Deep Neural Network,Energy Efficiency,Optical Power,Optical Signal,Optical Loss,Two-photon Absorption,Index Contrast,Processing Throughput,Microring Resonators,Achievable Throughput,Convolutional Neural Network,Input Values,Convolutional Neural Network Model,Analog-to-digital Converter,Elements,Optical Modes,Dot Product,Indium Tin Oxide,Density Matrix,Absorption Loss,Dot Product Operation,Refractive Index Contrast,Photonic Waveguides,Positive Aggregates,Input Encoding,Free Charge Carriers,Access Latency,Wavelength Channels,Analog Voltage
AI 理解论文
溯源树
样例
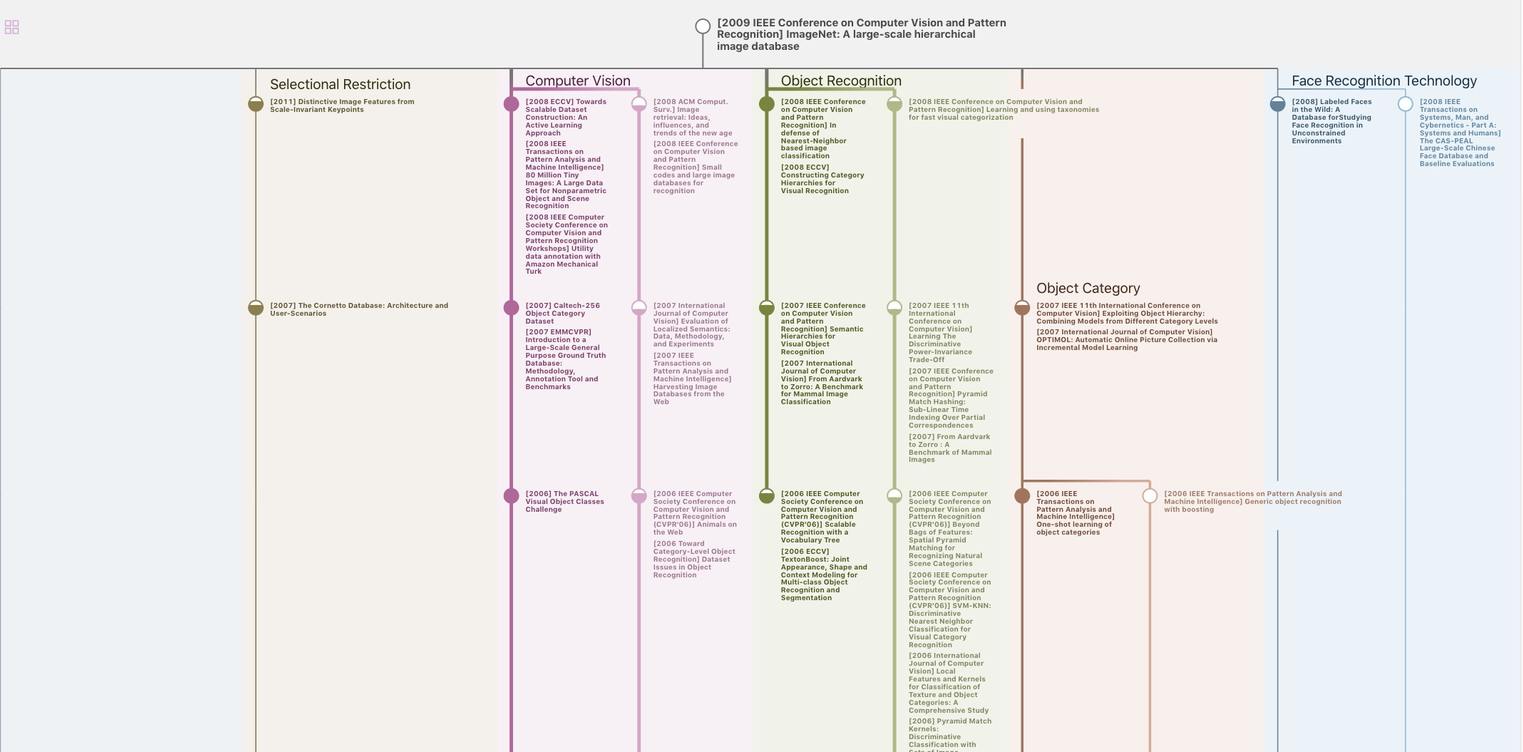
生成溯源树,研究论文发展脉络
Chat Paper
正在生成论文摘要